Can Spectral Information Work While Extracting Spatial Distribution? - An Online Spectral Information Compensation Network for HSI Classification.
IEEE transactions on image processing : a publication of the IEEE Signal Processing Society(2023)
摘要
In the past few years, deep learning-based methods have shown commendable performance for hyperspectral image (HSI) classification. Many works focus on designing independent spectral and spatial branches and then fusing the output features from two branches for category prediction. In this way, the correlation that exists between spectral and spatial information is not completely explored, and spectral information extracted from one branch is always not sufficient. Some studies also try to directly extract spectral-spatial features using 3D convolutions but are accompanied by the severe over-smoothing phenomenon and poor representation ability of spectral signatures. Unlike the above-mentioned approaches, in this paper, we propose a novel online spectral information compensation network (OSICN) for HSI classification, which consists of a candidate spectral vector mechanism, progressive filling process, and multi-branch network. To the best of our knowledge, this paper is the first to online supplement spectral information into the network when spatial features are extracted. The proposed OSICN makes the spectral information participate in network learning in advance to guide spatial information extraction, which truly processes spectral and spatial features in HSI as a whole. Accordingly, OSICN is more reasonable and more effective for complex HSI data. Experimental results on three benchmark datasets demonstrate that the proposed approach has more outstanding classification performance compared with the state-of-the-art methods, even with a limited number of training samples.
更多查看译文
关键词
Feature extraction, Data mining, Three-dimensional displays, Filling, Kernel, Task analysis, Information retrieval, HSI classification, deep learning, online spectral information compensation, progressive filling, multi-scale features
AI 理解论文
溯源树
样例
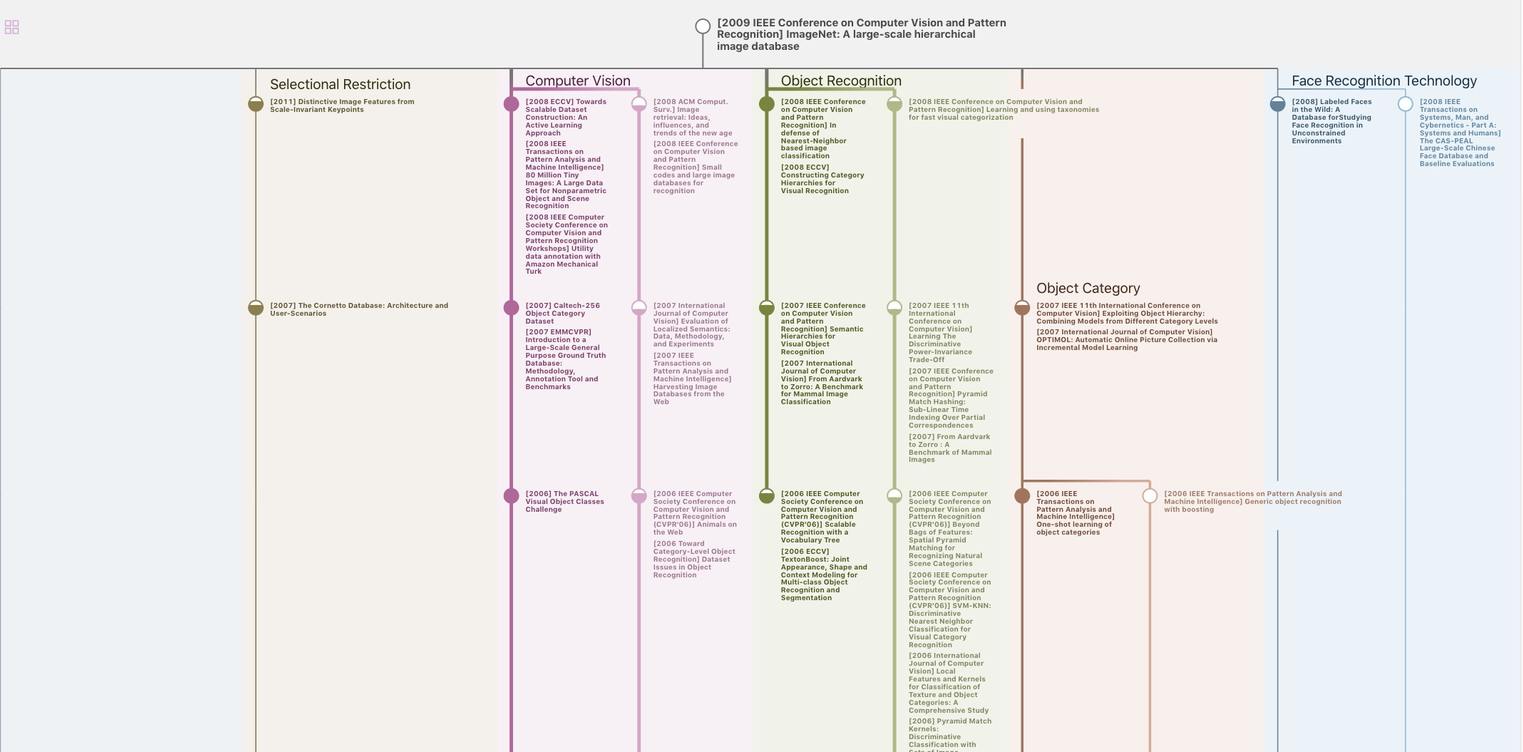
生成溯源树,研究论文发展脉络
Chat Paper
正在生成论文摘要