Enhancing Detection of Control State for High-Speed Asynchronous SSVEP-BCIs Using Frequency-Specific Framework.
IEEE transactions on neural systems and rehabilitation engineering : a publication of the IEEE Engineering in Medicine and Biology Society(2023)
摘要
This study proposed a novel frequency-specific (FS) algorithm framework for enhancing control state detection using short data length toward high-performance asynchronous steady-state visual evoked potential (SSVEP)-based brain-computer interfaces (BCI). The FS framework sequentially incorporated task-related component analysis (TRCA)-based SSVEP identification and a classifier bank containing multiple FS control state detection classifiers. For an input EEG epoch, the FS framework first identified its potential SSVEP frequency using the TRCA-based method and then recognized its control state using one of the classifiers trained on the features specifically related to the identified frequency. A frequency-unified (FU) framework that conducted control state detection using a unified classifier trained on features related to all candidate frequencies was proposed to compare with the FS framework. Offline evaluation using data lengths within 1 s found that the FS framework achieved excellent performance and significantly outperformed the FU framework. 14-target FS and FU asynchronous systems were separately constructed by incorporating a simple dynamic stopping strategy and validated using a cue-guided selection task in an online experiment. Using averaged data length of 591.63±5.65 ms, the online FS system significantly outperformed the FU system and achieved an information transfer rate, true positive rate, false positive rate, and balanced accuracy of 124.95±12.35 bits/min, 93.16±4.4%, 5.21±5.85%, and 92.89±4.02%, respectively. The FS system was also of higher reliability by accepting more correctly identified SSVEP trials and rejecting more wrongly identified ones. These results suggest that the FS framework has great potential to enhance the control state detection for high-speed asynchronous SSVEP-BCIs.
更多查看译文
关键词
Integrated circuits,Feature extraction,Classification algorithms,Frequency control,Task analysis,Electroencephalography,Heuristic algorithms,Asynchronous brain-computer interface,control state detection,steady-state visually evoked potentials (SSVEP)
AI 理解论文
溯源树
样例
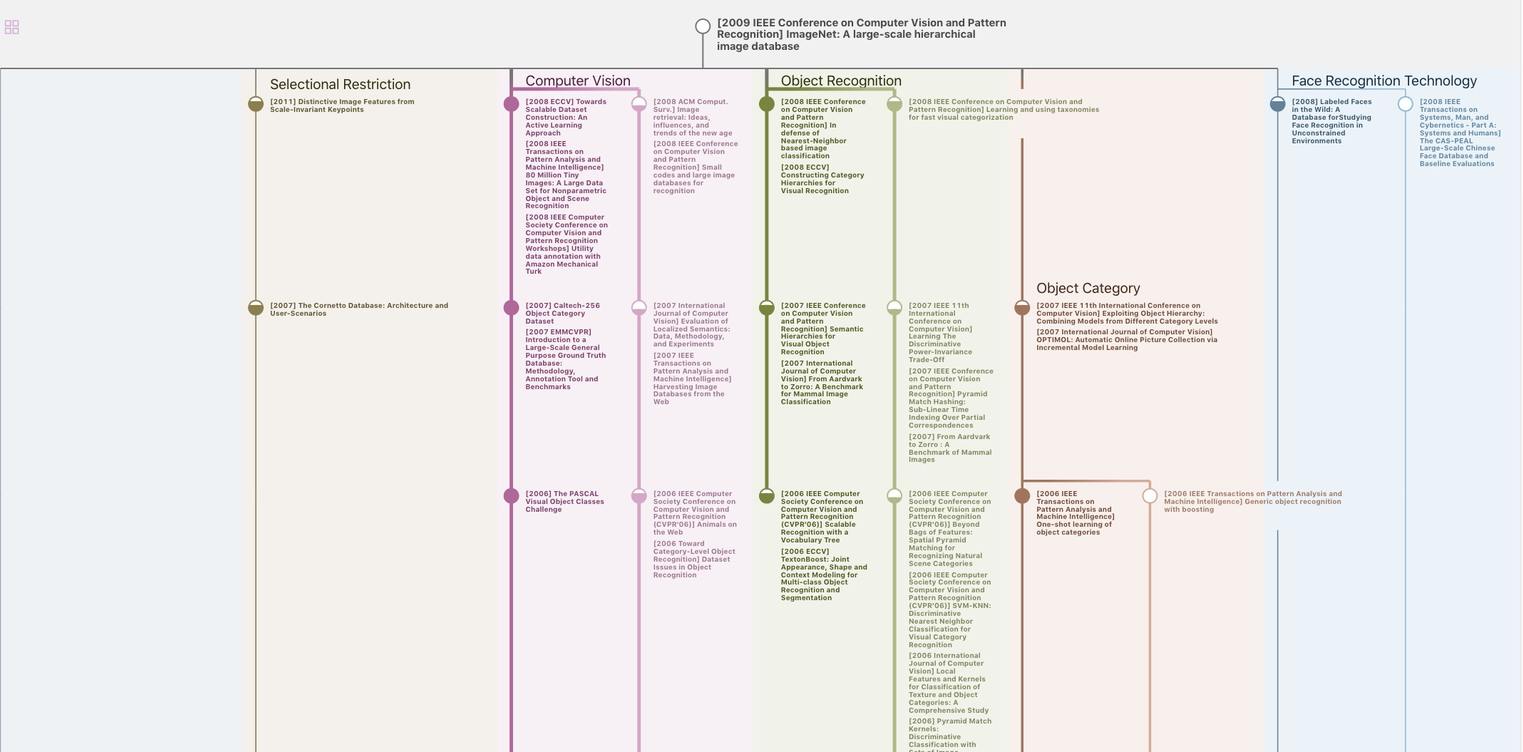
生成溯源树,研究论文发展脉络
Chat Paper
正在生成论文摘要