Reinforcement Learning Control With Knowledge Shaping
IEEE TRANSACTIONS ON NEURAL NETWORKS AND LEARNING SYSTEMS(2024)
摘要
We aim at creating a transfer reinforcement learning framework that allows the design of learning controllers to leverage prior knowledge extracted from previously learned tasks and previous data to improve the learning performance of new tasks. Toward this goal, we formalize knowledge transfer by expressing knowledge in the value function in our problem construct, which is referred to as reinforcement learning with knowledge shaping (RL-KS). Unlike most transfer learning studies that are empirical in nature, our results include not only simulation verifications but also an analysis of algorithm convergence and solution optimality. Also different from the well-established potential-based reward shaping methods which are built on proofs of policy invariance, our RL-KS approach allows us to advance toward a new theoretical result on positive knowledge transfer. Furthermore, our contributions include two principled ways that cover a range of realization schemes to represent prior knowledge in RL-KS. We provide extensive and systematic evaluations of the proposed RL-KS method. The evaluation environments not only include classical RL benchmark problems but also include a challenging task of real-time control of a robotic lower limb with a human user in the loop.
更多查看译文
关键词
Task analysis,Knowledge transfer,Reinforcement learning,Transfer learning,Silicon,Knowledge representation,Convergence,Reinforcement learning (RL),reward shaping,transfer learning,value function
AI 理解论文
溯源树
样例
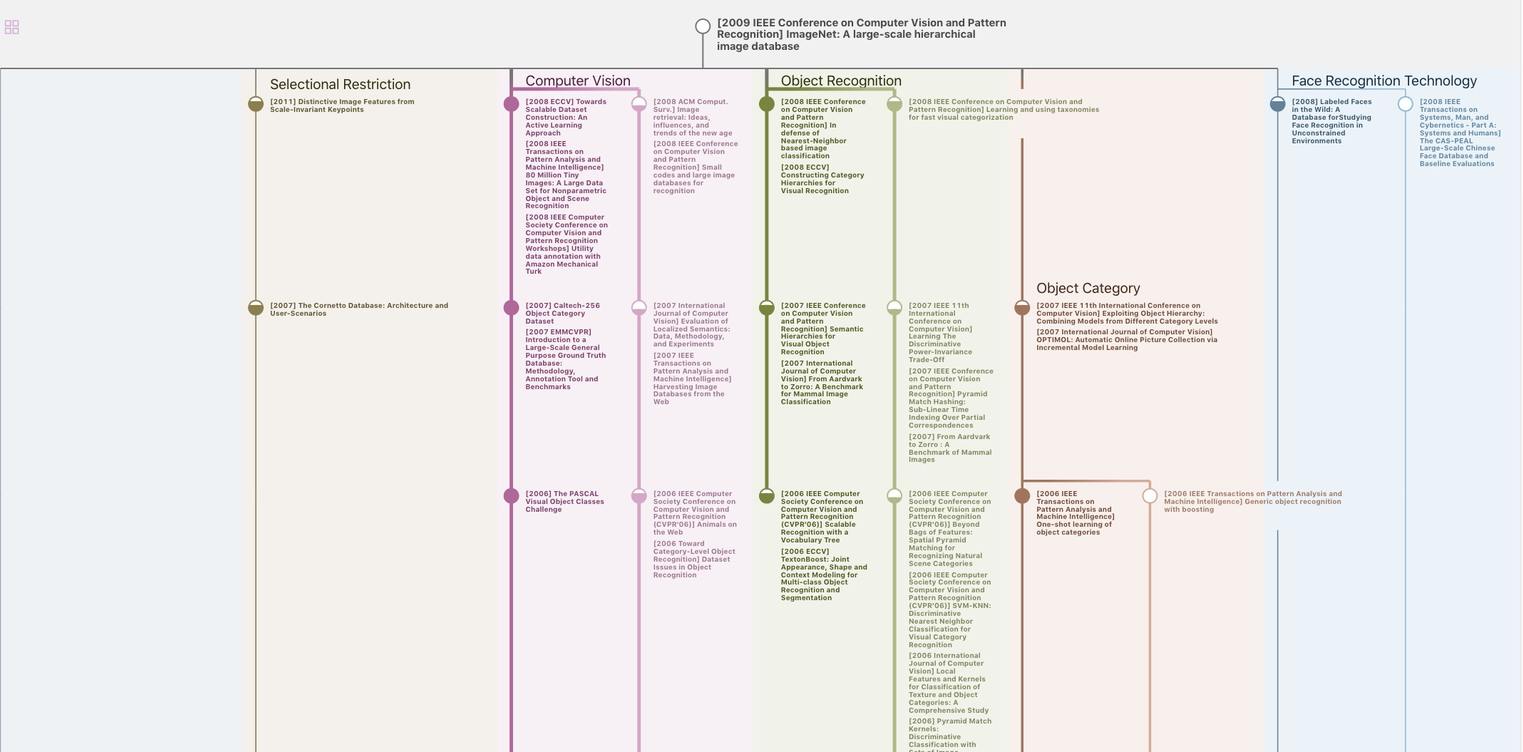
生成溯源树,研究论文发展脉络
Chat Paper
正在生成论文摘要