Learning to estimate heart rate from accelerometer and user's demographics during physical exercises.
IEEE journal of biomedical and health informatics(2023)
摘要
Getting prompt insights about health and well-being in a non-invasive way is one of the most popular features available on wearable devices. Among all vital signs available, heart rate (HR) monitoring is one of the most important since other measurements are based on it. Real-time HR estimation in wearables mostly relies on photoplethysmography (PPG), which is a fair technique to handle such a task. However, PPG is vulnerable to motion artifacts (MA). As a consequence, the HR estimated from PPG signals is strongly affected during physical exercises. Different approaches have been proposed to deal with this problem, however, they struggle to handle exercises with strong movements, such as a running session. In this paper, we present a new method for HR estimation in wearables that uses an accelerometer signal and user demographics to support the HR prediction when the PPG signal is affected by motion artifacts. This algorithm requires a tiny memory allocation and allows on-device personalization since the model parameters are finetuned in real time during workout executions. Also, the model may predict HR for a few minutes without using a PPG, which represents a useful contribution to an HR estimation pipeline. We evaluate our model on five different exercise datasets - performed on treadmills and in outdoor environments - and the results show that our method can improve the coverage of a PPG-based HR estimator while keeping a similar error performance, which is particularly useful to improve user experience.
更多查看译文
关键词
Heart Rate,PPG,Accelerometer,Wearables
AI 理解论文
溯源树
样例
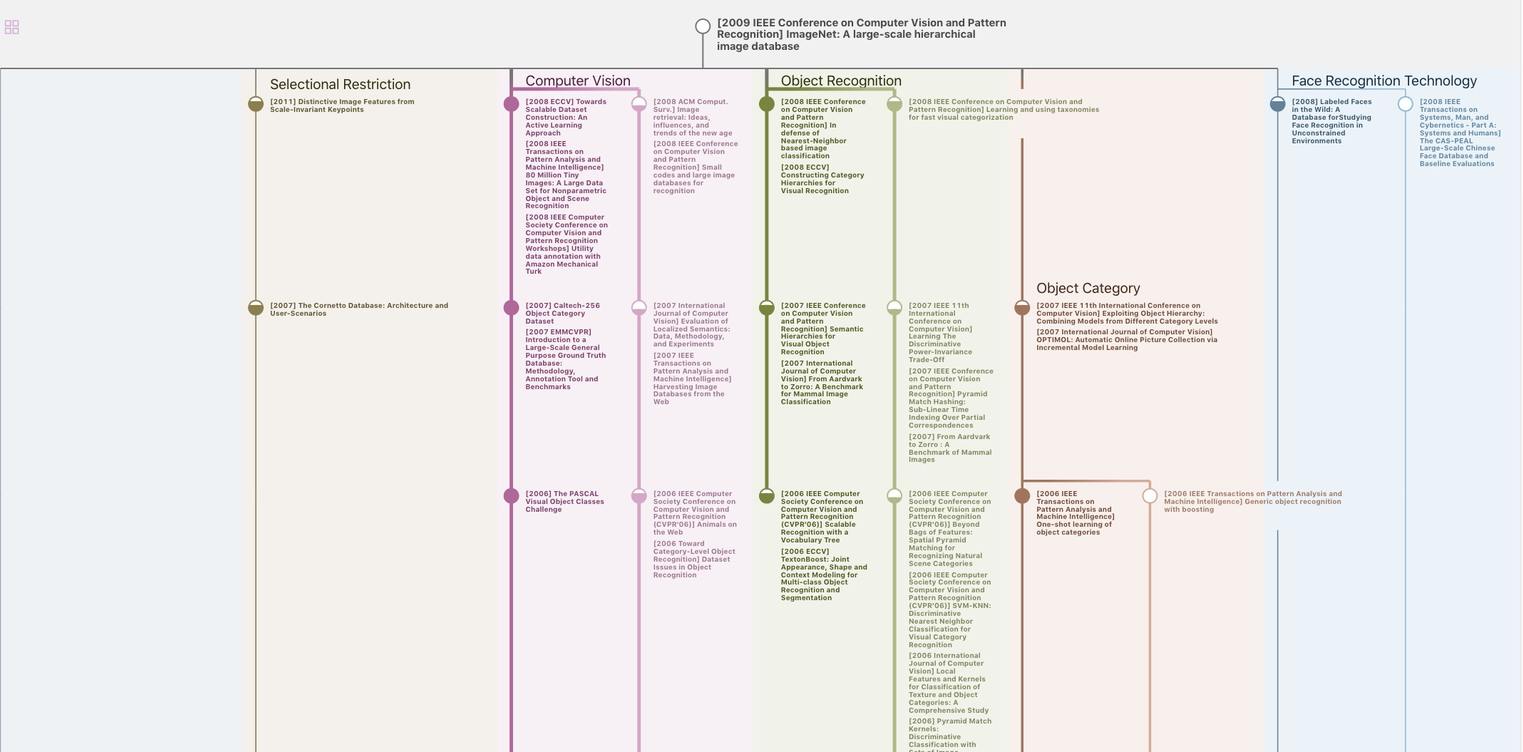
生成溯源树,研究论文发展脉络
Chat Paper
正在生成论文摘要