Adjustable privacy using autoencoder-based learning structure
NEUROCOMPUTING(2024)
摘要
Inference centers need more data to have a more comprehensive and beneficial learning model, and for this purpose, they need to collect data from data providers. On the other hand, data providers are cautious about delivering their datasets to inference centers in terms of privacy considerations. In this paper, by modifying the structure of the autoencoder, we present a method that manages the utility-privacy trade-off well. To be more precise, the data is first compressed using the encoder, then confidential and non-confidential features are separated and uncorrelated using the classifier. The confidential feature is appropriately combined with noise, and the non-confidential feature is enhanced, and at the end, data with the original data format is produced by the decoder. The suggested architecture additionally enables data providers to modify the degree of privacy needed for private features and the level of utility for non-private features. The proposed method has been examined for both image and categorical databases, and the results show a significant performance improvement compared to previous methods.
更多查看译文
关键词
Privacy,Utility,Deep neural networks,Autoencoders,Collaborative learning
AI 理解论文
溯源树
样例
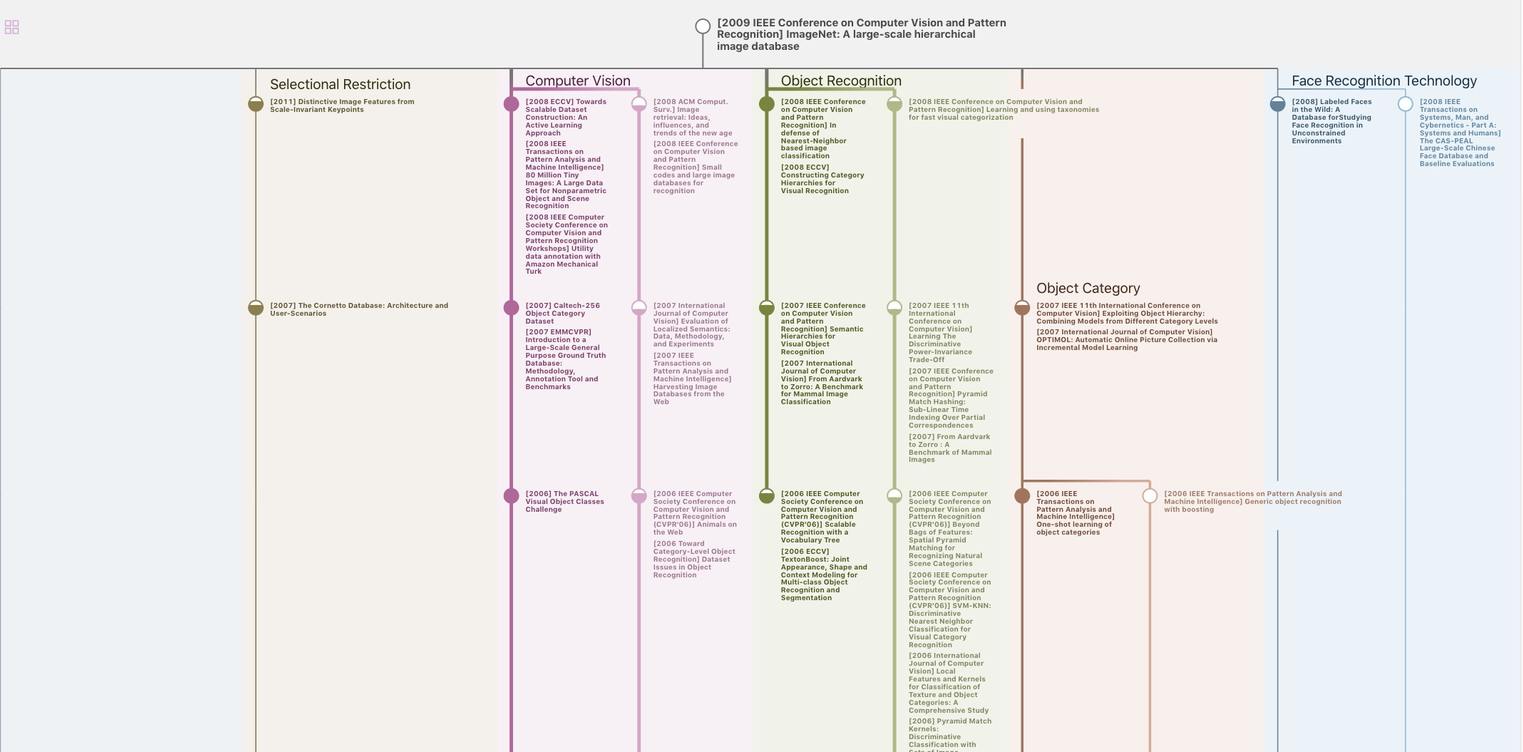
生成溯源树,研究论文发展脉络
Chat Paper
正在生成论文摘要