Multi-Modal U-net for Segmenting Gross Tumor Volume in Lungs during Radiotherapy
crossref(2023)
摘要
In this work we introduce an end-to-end multi-modal neural network to segment the Gross Tumor Volume (GTV) from 3D-CBCT’s during radiotherapy. We improve the tumor segmentation by using a U-net which takes additional information such as the tumor mask generated at the planning phase along with the CBCT volume. The mask contains relevant information about the tumor’s location and can guide the model to use this knowledge appropriately to give a better prediction. This technique could become an alternative to produce segmentation masks of GTV in CBCT automatically during radiotherapy as in the traditional RT-pipeline, they are not segmented. We have evaluated our model on a dataset of 82 patients who have undergone radiotherapy. We compare the results of registered target volumes from planning CT as mask seed with 2 different types of multi-modal architectures. Our model shows a DSC of 0.706±0.002 with Late Fusion and 0.702±0.015 with Early Fusion using the GTV Mask. The performance of the two models on this mask is similar, so we perform further experiments with different types of masks which suggest that Late Fusion model produces a better segmentation of the tumor than the Early Fusion model. We also provide an ablation study consisting of a single modality U-net and a metric based on the Planning CT mask registration. It indicates a clear advantage of using our model to produce segmentation for this type of imaging.
更多查看译文
AI 理解论文
溯源树
样例
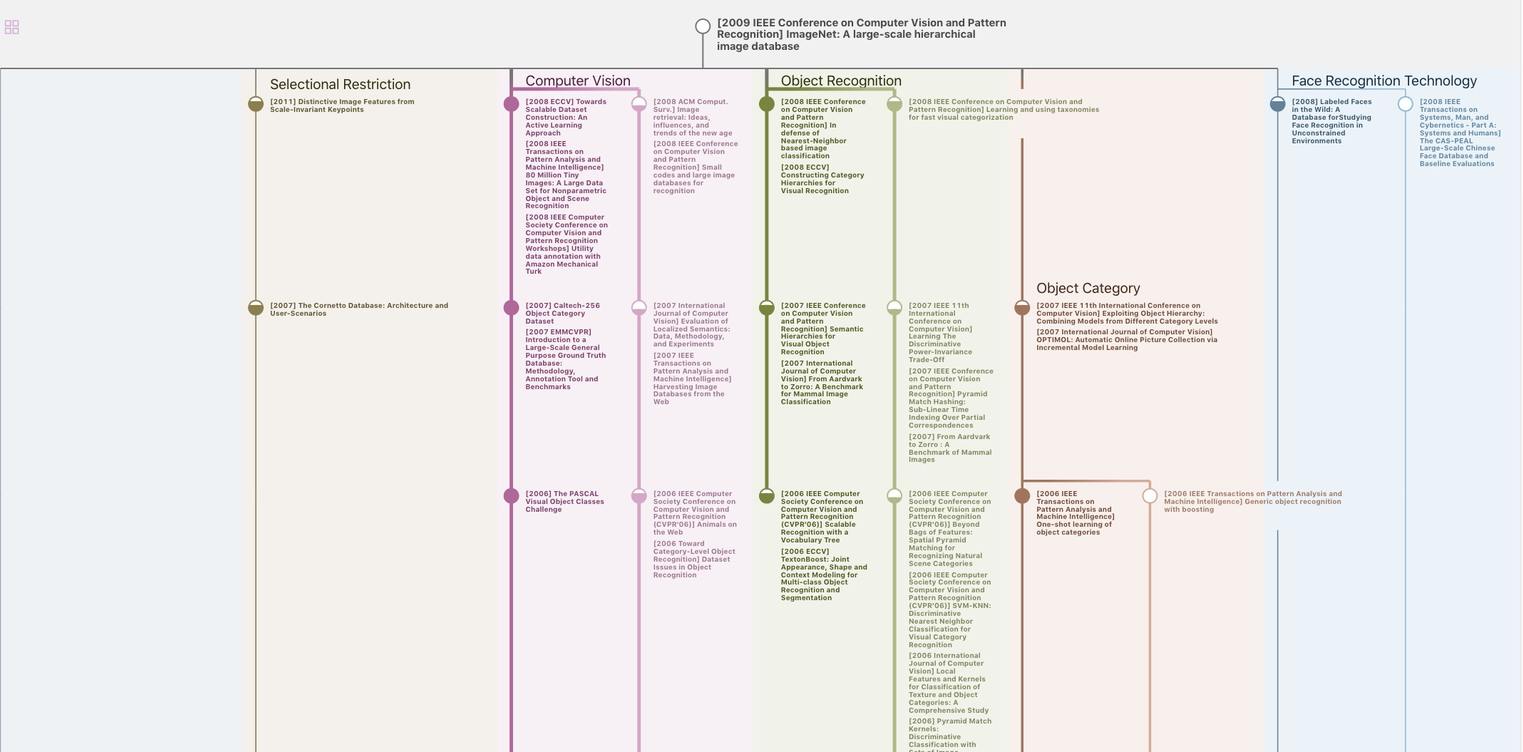
生成溯源树,研究论文发展脉络
Chat Paper
正在生成论文摘要