A novel deep clustering network using multi-representation autoencoder and adversarial learning for large cross-domain fault diagnosis of rolling bearings
Expert Systems with Applications(2023)
摘要
Intelligent fault diagnosis based on deep learning has been more attractive in practical engineering. However, its accuracy is constrained by unlabeled data and large domain shift for cross-domain fault diagnosis. As an unsupervised learning network, the autoencoder (AE) can relieve the pressure of unlabeled data. Using it as a building block, this paper presents a novel deep clustering network, named as clustering graph convolutional network with multiple adversarial learning (c-GCN-MAL) for intelligent fault diagnosis of various bearings. First, multiple representation of datasets, i.e., the datasets and their structured relatedness, are extracted by AE and graph convolutional network (GCN) to recognize different classes and their related information. Then, the dataadversarial and domain-adversarial are individually set by newly defining the loss function of the proposed deep network, so that its clustering and transfer ability for new dataset can be enhanced. In experiments, it is applied to four open-source bearing datasets and one dataset of practical bearings. Transfer tasks between different rotation speeds, damage generation, and experimental bearings, even the transfer from experimental bearings to wheelset bearings used in real tests, are assigned to verify the effectiveness of the proposed network. The experimental results and comparisons indicate that the proposed deep clustering network is feasible for the transfer with large domain shift and can provide noticeably higher accuracy and stable results for cross-domain fault diagnosis of bearings.
更多查看译文
关键词
Data clustering,Autoencoder,Deep learning,Transfer learning,Fault diagnosis,Bearing
AI 理解论文
溯源树
样例
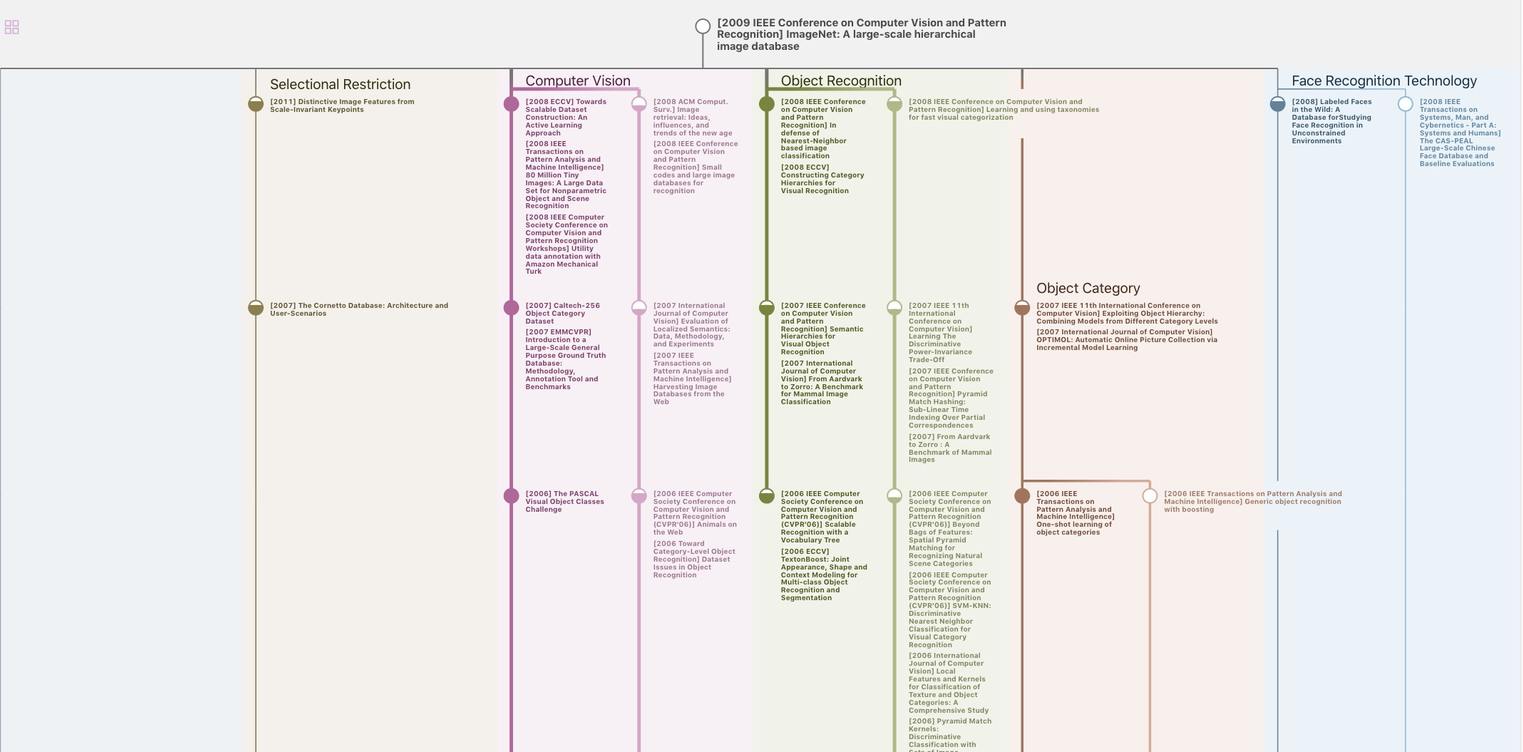
生成溯源树,研究论文发展脉络
Chat Paper
正在生成论文摘要