Tensor-Empowered Adaptive Learning for Few-Shot Streaming Tasks.
IEEE transactions on neural networks and learning systems(2023)
摘要
Various stream learning methods are emerging in an endless stream to provide a wealth of solutions for artificial intelligence in streaming data scenarios. However, when each data stream is oriented to a different target space, it forces stream learning approaches oriented to the same task to be no longer applicable. Due to inconsistent target spaces for different tasks, the previous approaches fail on the new streaming tasks or it is impracticable to be trained from scratch with few labeled samples at the beginning. To this end, we have proposed an adaptive learning scheme for few-shot streaming tasks with the contributions of tensor and meta-learning. This adaptive scheme is conducive to mitigating the domain shift when a new task has few labeled samples. We elaborate a novel tensor-empowered attention mechanism derived from nonlocal neural networks, which enables to capture long-range dependency and preserve the high-dimensional structure to refine the global features of streaming tasks. Furthermore, we develop a fine-grained similarity computing approach, which is prone to better characterize the difference across few-shot streaming tasks. To show the superiority of our method, we have carried out extensive experiments on three popular few-shot datasets to simulate streaming tasks and evaluate the performance of adaptation. The results show that our proposed method has achieved competitive performance for few-shot streaming tasks compared with the state-of-the-art (SOTA).
更多查看译文
关键词
Task analysis,Tensors,Training,Feature extraction,Adaptive learning,Measurement,Computational modeling,crossing attention,few-shot learning (FSL),streaming task learning,tensor representation
AI 理解论文
溯源树
样例
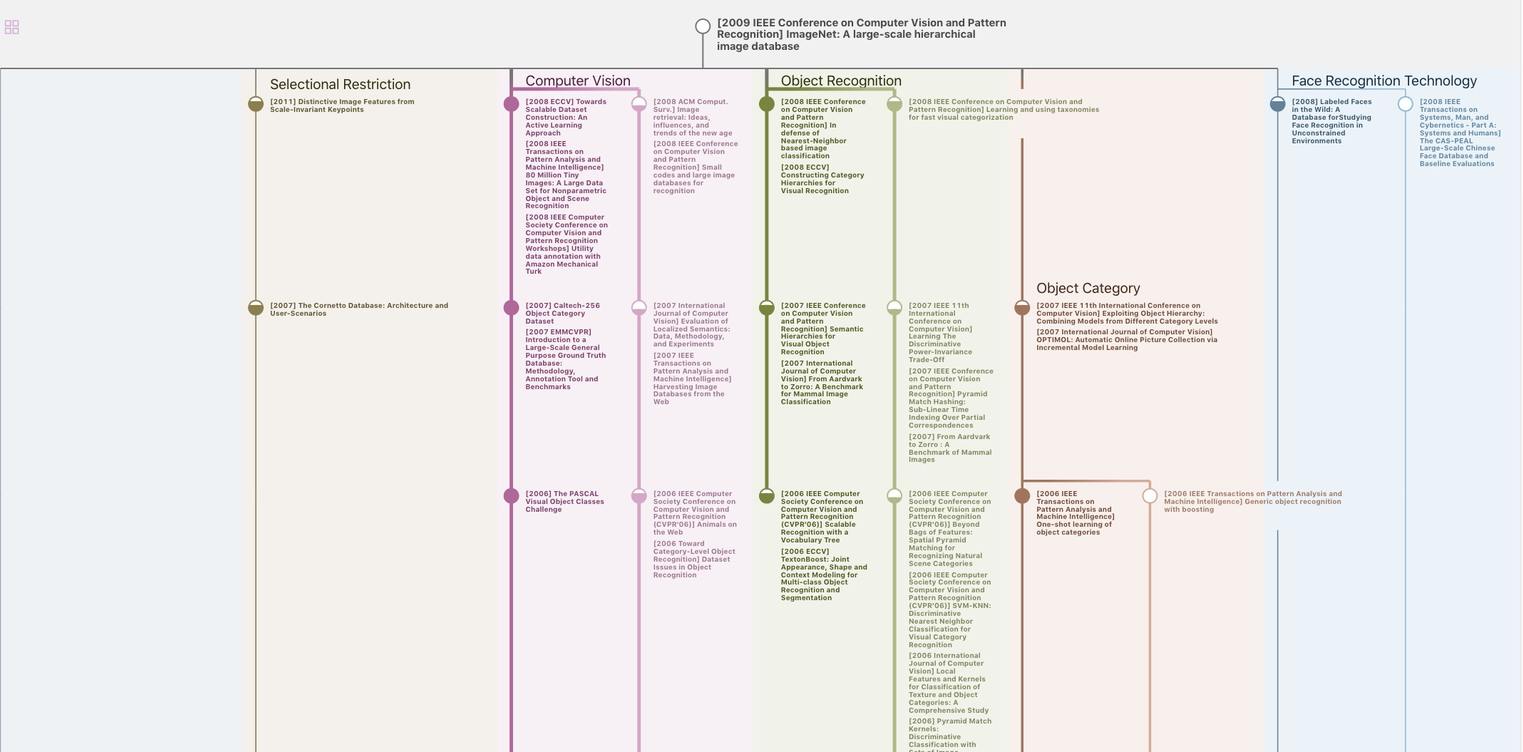
生成溯源树,研究论文发展脉络
Chat Paper
正在生成论文摘要