Generalized Precision Matrix for Scalable Estimation of Nonparametric Markov Networks
arXiv (Cornell University)(2023)
摘要
A Markov network characterizes the conditional independence structure, or Markov property, among a set of random variables. Existing work focuses on specific families of distributions (e.g., exponential families) and/or certain structures of graphs, and most of them can only handle variables of a single data type (continuous or discrete). In this work, we characterize the conditional independence structure in general distributions for all data types (i.e., continuous, discrete, and mixed-type) with a Generalized Precision Matrix (GPM). Besides, we also allow general functional relations among variables, thus giving rise to a Markov network structure learning algorithm in one of the most general settings. To deal with the computational challenge of the problem, especially for large graphs, we unify all cases under the same umbrella of a regularized score matching framework. We validate the theoretical results and demonstrate the scalability empirically in various settings.
更多查看译文
关键词
markov networks,scalable estimation,nonparametric
AI 理解论文
溯源树
样例
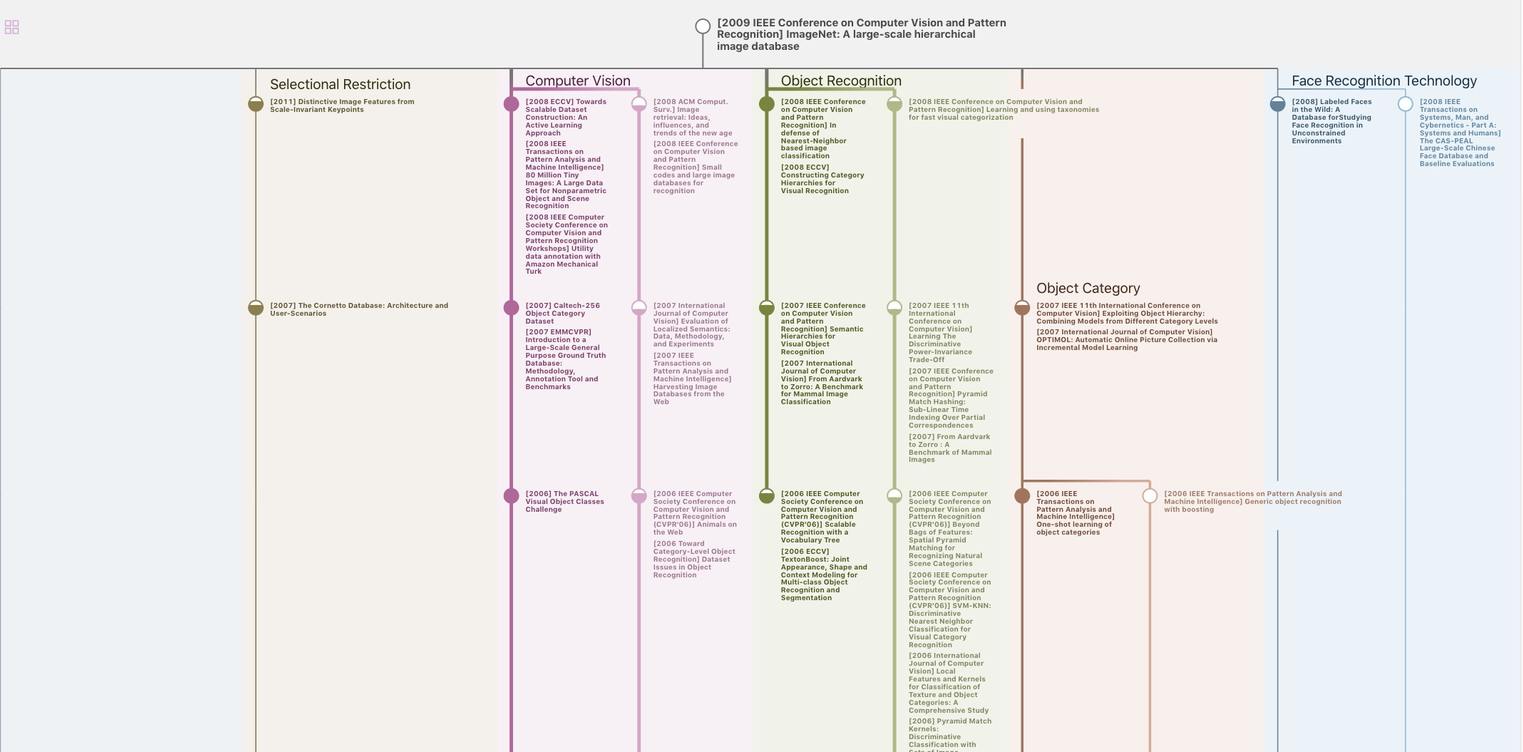
生成溯源树,研究论文发展脉络
Chat Paper
正在生成论文摘要