Gradient-Based Transfer Learning
ICLR 2023(2023)
摘要
We formulate transfer learning as a meta-learning problem by extending upon the current meta-learning paradigm in that support and query data are drawn from different, but related distributions of tasks. Inspired by the success of Gradient-Based Meta-Learning (GBML), we propose to expand it to the transfer learning setting by constructing a general encoder-decoder architecture that learns a map between functionals of different domains. This is achieved by leveraging on the idea that the task-adapted parameters of a meta-learner can serve as an informative representation of the task itself. We demonstrate the proposed method on regression, prediction of dynamical systems and meta-imitation learning problems.
更多查看译文
关键词
meta-learning,gradient-based meta-learning,transfer learning,representation learning
AI 理解论文
溯源树
样例
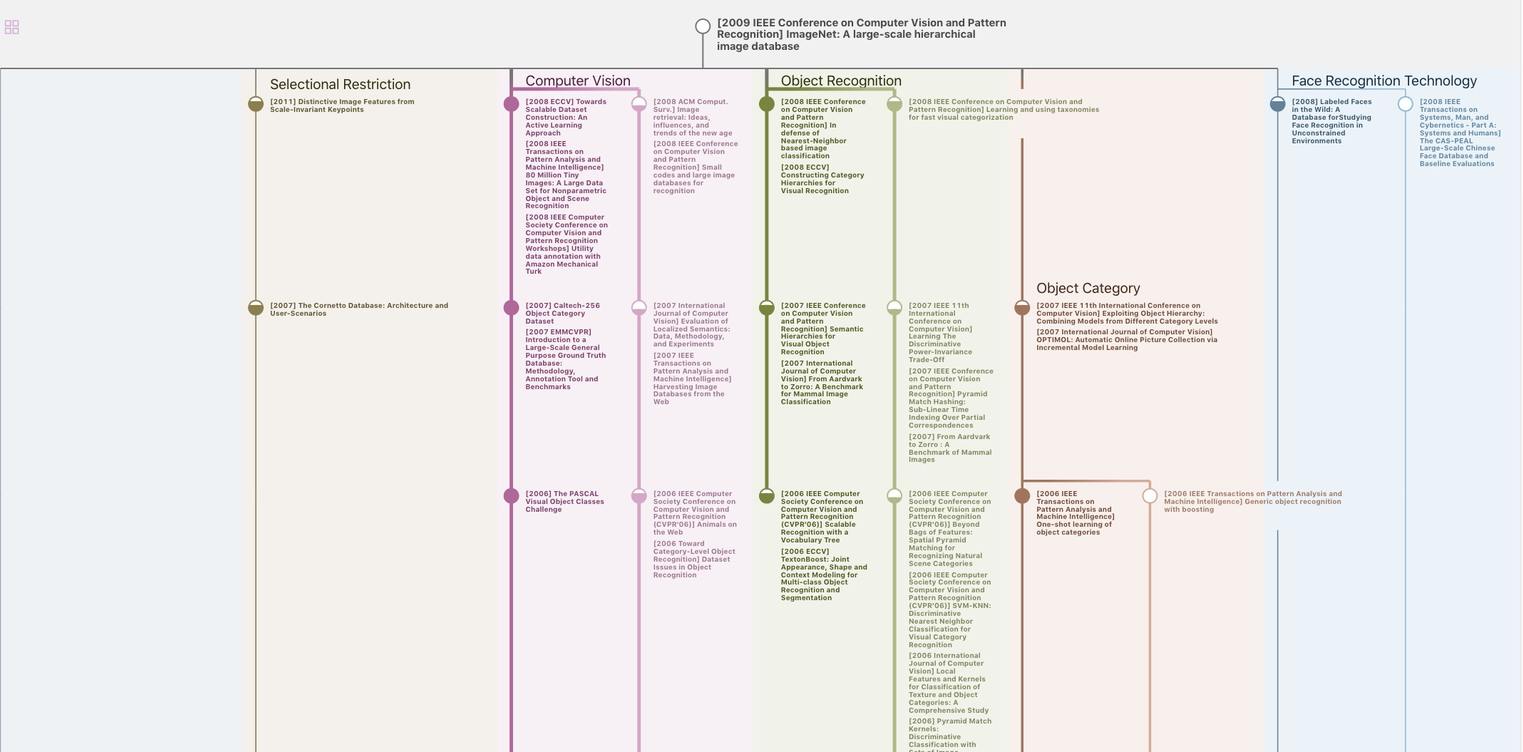
生成溯源树,研究论文发展脉络
Chat Paper
正在生成论文摘要