Mitigating the Limitations of Multimodal VAEs with Coordination-Based Approach
ICLR 2023(2023)
摘要
One of the key challenges in multimodal variational autoencoders (VAEs) is inferring a joint representation from arbitrary subsets of modalities. The state-of-the-art approach to achieving this is to sub-sample the modality subsets and learn to generate all modalities from them. However, this sub-sampling in the mixture-based approach has been shown to degrade other important features of multimodal VAEs, such as quality of generation, and furthermore, this degradation is theoretically unavoidable. In this study, we focus on another approach to learning the joint representation by bringing unimodal inferences closer to joint inference from all modalities, which does not have the above limitation. Although there have been models that can be categorized under this approach, they were derived from different backgrounds; therefore, the relation and superiority between them were not clear. To take a unified view, we first categorize them as coordination-based multimodal VAEs and show that these can be derived from the same multimodal evidence lower bound (ELBO) and that the difference in their performance is related to whether they are more tightly lower bounded. Next, we point out that these existing coordination-based models perform poorly on cross-modal generation (or cross-coherence) because they do not learn to reconstruct modalities from unimodal inferences. Therefore, we propose a novel coordination-based model that incorporates these unimodal reconstructions, which avoids the limitations of both mixture and coordination-based models. Experiments with diverse and challenging datasets show that the proposed model mitigates the limitations in multimodal VAEs and performs well in both cross-coherence and generation quality.
更多查看译文
关键词
mutlimodal learning,deep generative models
AI 理解论文
溯源树
样例
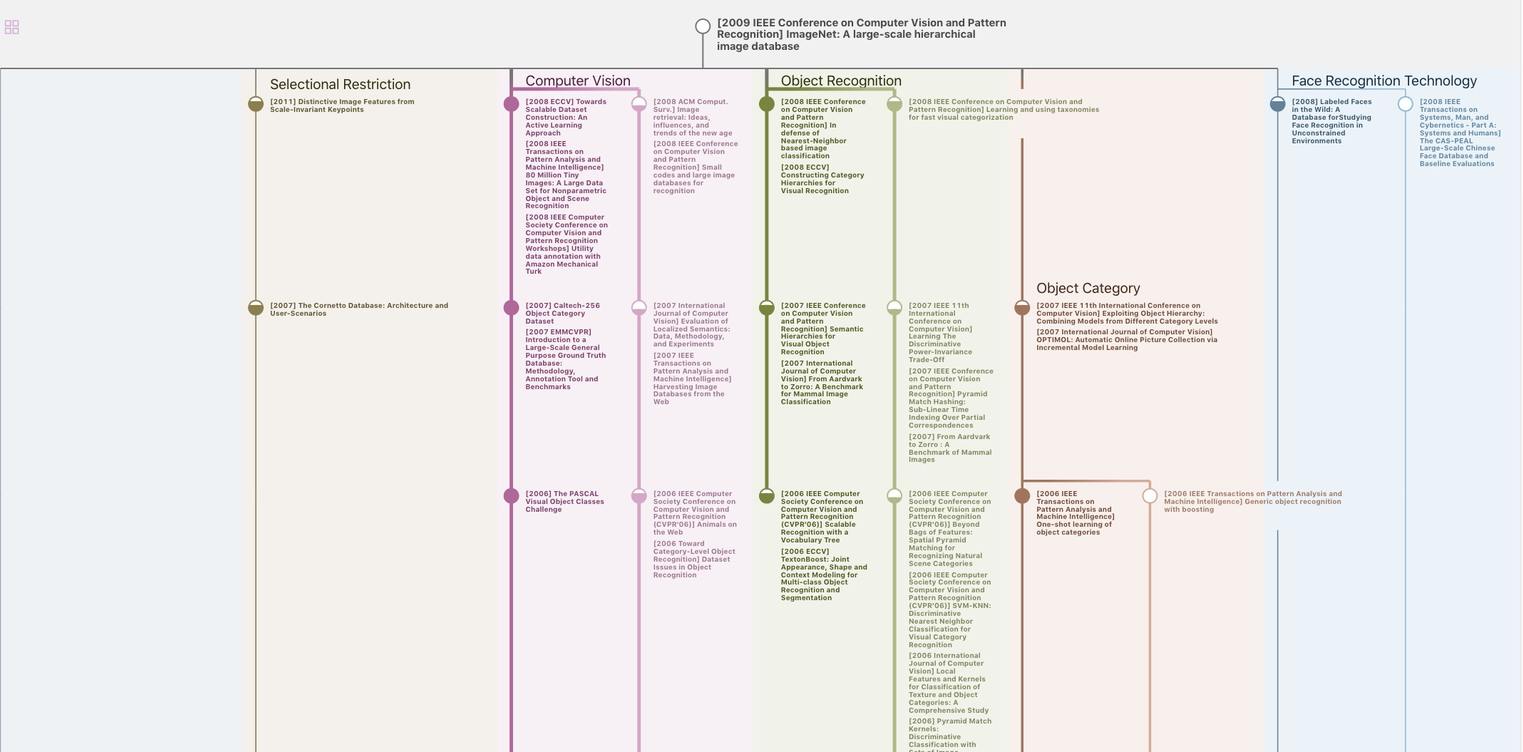
生成溯源树,研究论文发展脉络
Chat Paper
正在生成论文摘要