Noise Transforms Feed-Forward Networks into Sparse Coding Networks
ICLR 2023(2023)
摘要
A hallmark of biological neural networks, which distinguishes them from their artificial counterparts, is the high degree of sparsity in their activations. Here, we show that by simply injecting symmetric, random, noise during training in reconstruction or classification tasks, artificial neural networks with ReLU activation functions eliminate this difference; the neurons converge to a sparse coding solution where only a small fraction are active for any input. The resulting network learns receptive fields like those of primary visual cortex and remains sparse even when noise is removed in later stages of learning.
更多查看译文
关键词
Sparse Coding,Sparsity,Top-K Activation,Noise,Biologically Inspired
AI 理解论文
溯源树
样例
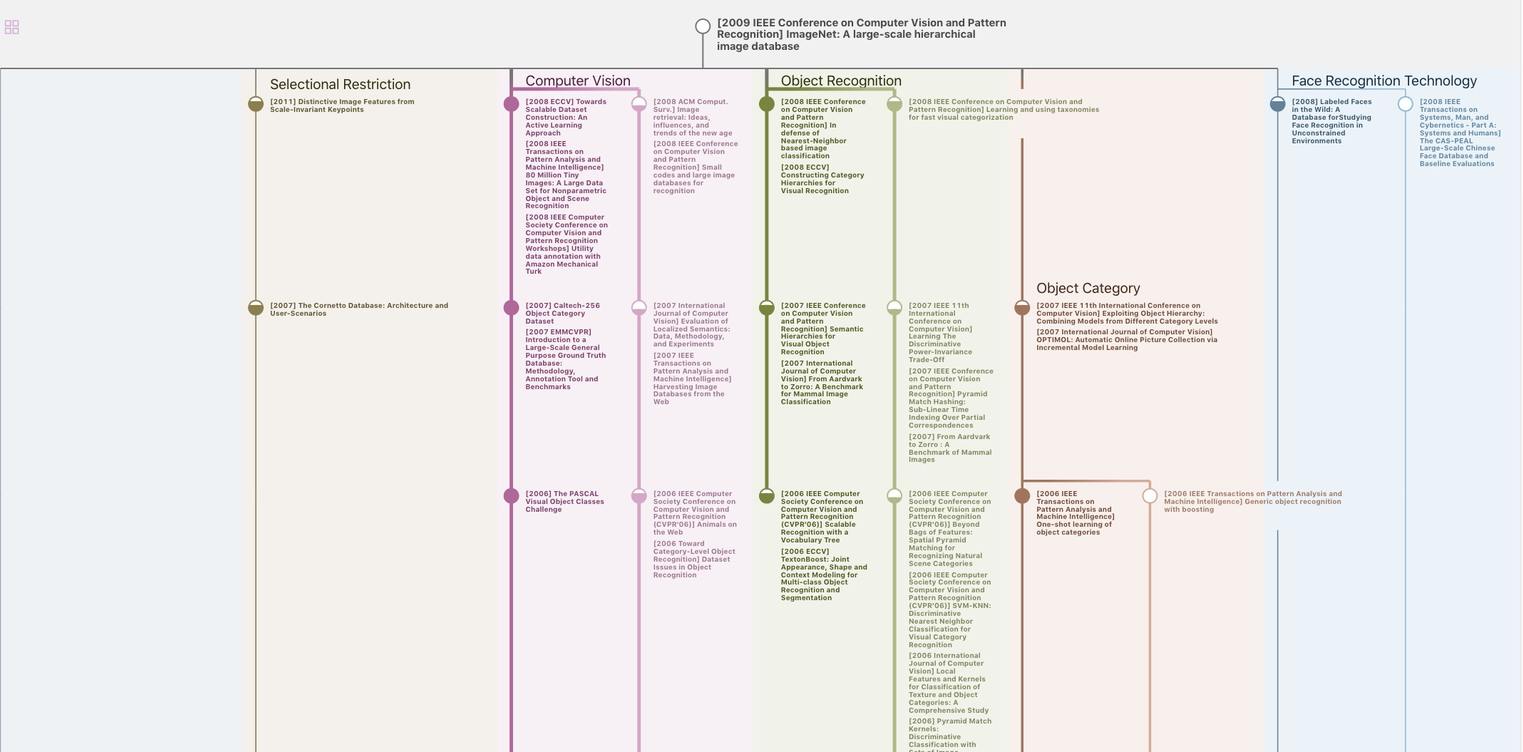
生成溯源树,研究论文发展脉络
Chat Paper
正在生成论文摘要