A Probabilistic Framework for Modular Continual Learning
arXiv (Cornell University)(2023)
摘要
Modular approaches, which use a different composition of modules for each problem and avoid forgetting by design, have been shown to be a promising direction in continual learning (CL). However, searching through the large, discrete space of possible module compositions is a challenge because evaluating a composition's performance requires a round of neural network training. To address this challenge, we develop a modular CL framework, called PICLE, that accelerates search by using a probabilistic model to cheaply compute the fitness of each composition. The model combines prior knowledge about good module compositions with dataset-specific information. Its use is complemented by splitting up the search space into subsets, such as perceptual and latent subsets. We show that PICLE is the first modular CL algorithm to achieve different types of transfer while scaling to large search spaces. We evaluate it on two benchmark suites designed to capture different desiderata of CL techniques. On these benchmarks, PICLE offers significantly better performance than state-of-the-art CL baselines.
更多查看译文
关键词
modular,probabilistic framework,learning
AI 理解论文
溯源树
样例
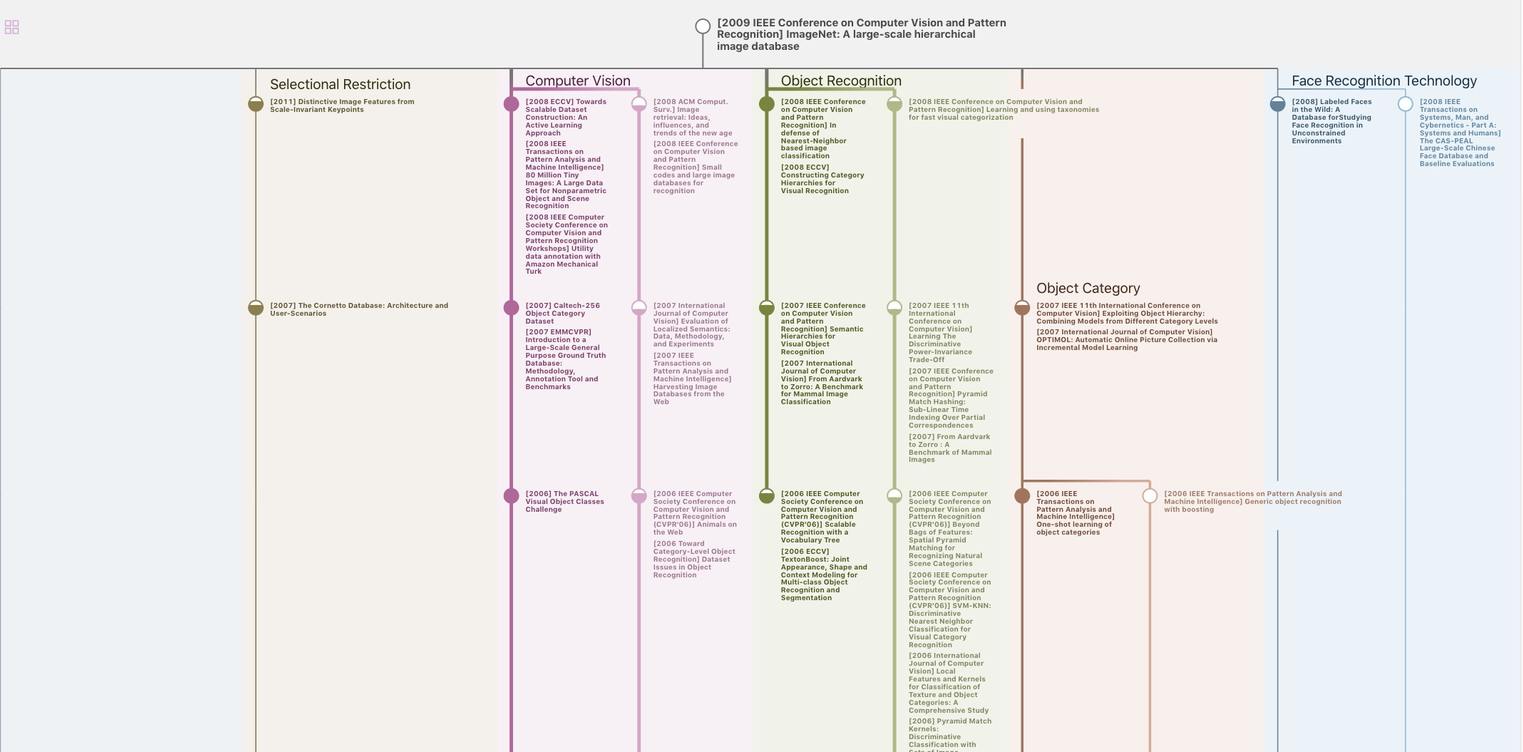
生成溯源树,研究论文发展脉络
Chat Paper
正在生成论文摘要