Kuiper: Moderated Asynchronous Federated Learning on Heterogeneous Mobile Devices with Non-IID Data
ICLR 2023(2023)
摘要
Federated learning allows multiple clients to jointly learn an ML model while keeping their data private. While synchronous federated learning (Sync-FL) requires the devices to share local gradients synchronously to provide better guarantees, it suffers from the problem of stragglers. This is the scenario where the faster clients have to wait for the slower ones, slowing the entire training process. Conventional techniques completely drop the updates from the stragglers and lose the opportunity to learn from the data they hold, which is especially important in a non-iid setting. Asynchronous learning (Async-FL) provides a potential solution to allow the clients to function at their own pace, which typically achieves faster convergence. Since edge devices have a low compute, it is hard to train a video action recognition task on them. We present Kuiper, a variant of Async-FL, to help heterogeneous edge devices with limited resources learn a heavy model on video-action-recognition tasks with data distributed non-IID. Kuiper introduces a novel aggregation scheme, which solves the straggler problem while considering the different data distribution at different clients. Kuiper shows a 11% faster convergence compared to Oort15 [OSDI-21], up to 12% and 9% improvement in test accuracy compared to FedBuff16 [AISTAT-22] and Oort [OSDI-21] on HMDB51, and 10% and 9% on UCF101.
更多查看译文
关键词
Federated Learning,Edge devices,Non-IID,Video action recognition
AI 理解论文
溯源树
样例
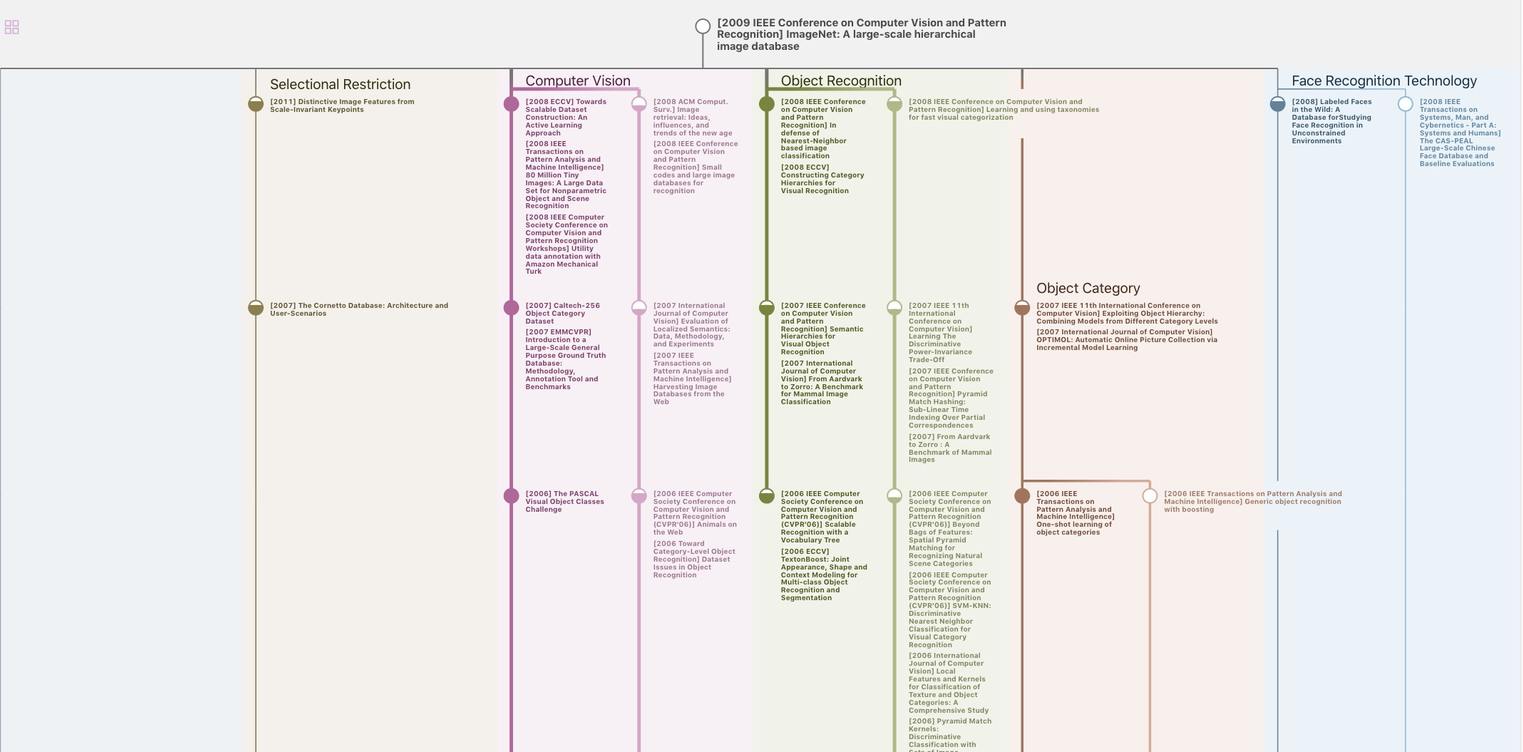
生成溯源树,研究论文发展脉络
Chat Paper
正在生成论文摘要