Factors Influencing Generalization in Chaotic Dynamical Systems
ICLR 2023(2023)
摘要
Many real-world systems exhibit chaotic behaviour, for example: weather, fluid dynamics, stock markets, natural ecosystems, and disease transmission. While chaotic systems are often thought to be completely unpredictable, in fact there are patterns within and across that experts frequently describe and contrast qualitatively. We hypothesize that given the right supervision / task definition, representation learning systems will be able to pick up on these patterns, and successfully generalize both in- and out-of-distribution (OOD).
Thus, this work explores and identifies key factors which lead to good generalization. We observe a variety of interesting phenomena, including: learned representations transfer much better when fine-tuned vs. frozen; forecasting appears to be the best pre-training task; OOD robustness falls off very quickly outside the training distribution; recurrent architectures generally outperform others on OOD generalization.
Our findings are of interest to any domain of prediction where chaotic dynamics play a role.
更多查看译文
关键词
generalization,multi-task learning,chaos,dynamical systems,representation learning
AI 理解论文
溯源树
样例
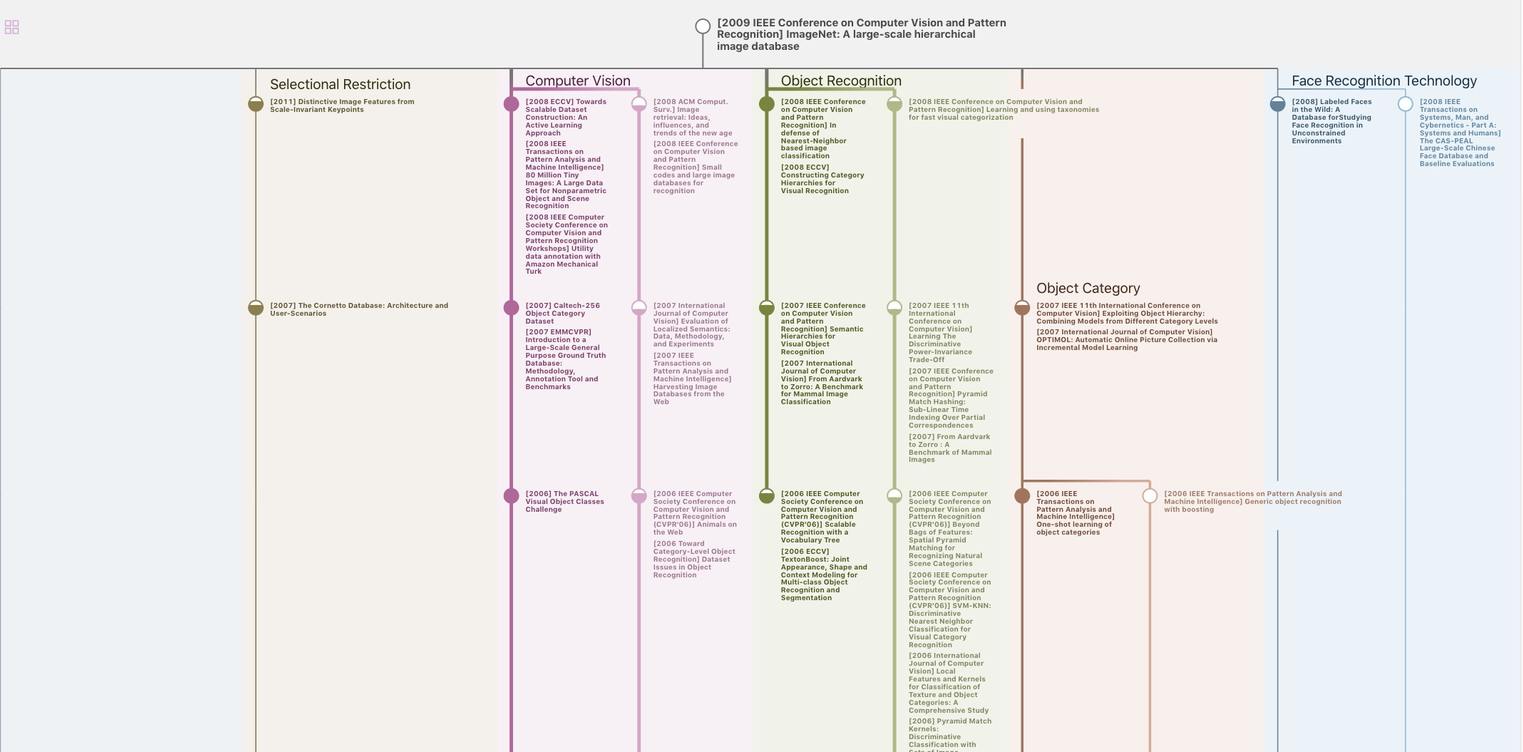
生成溯源树,研究论文发展脉络
Chat Paper
正在生成论文摘要