Revisiting Information-Based Clustering with Pseudo-Posterior Models
ICLR 2023(2023)
摘要
Maximization of mutual information (MI) between the network's input and output motivates standard losses for unsupervised discriminative clustering enforcing "decisiveness" and "fairness". In the context of common softmax models, we clarify several general properties of such discriminative losses that were previously not well understood: the relation to K-means, or lack thereof, and "margin-maximization". In particular, we show that "desiciveness" without the extra regularization term can lead to poor classification margins. Also, non-convexity of information-based losses motivates us to focus on self-supervised approaches introducing effective higher-order optimization algorithms with auxiliary variables. Addressing limitations of existing formulations, we propose a new self-supervised loss with soft auxiliary variables, or "pseudo-confidence" estimates. In particular, we introduce "strong" fairness and motivate the "reverse" cross-entropy as a robust loss for network training from noisy pseudo-confidence estimates. The latter is efficiently computed using variational inference - we derive a new EM algorithm with closed-form solutions for E and M steps. Empirically, our algorithm improves the performance of earlier methods for information-based clustering.
更多查看译文
AI 理解论文
溯源树
样例
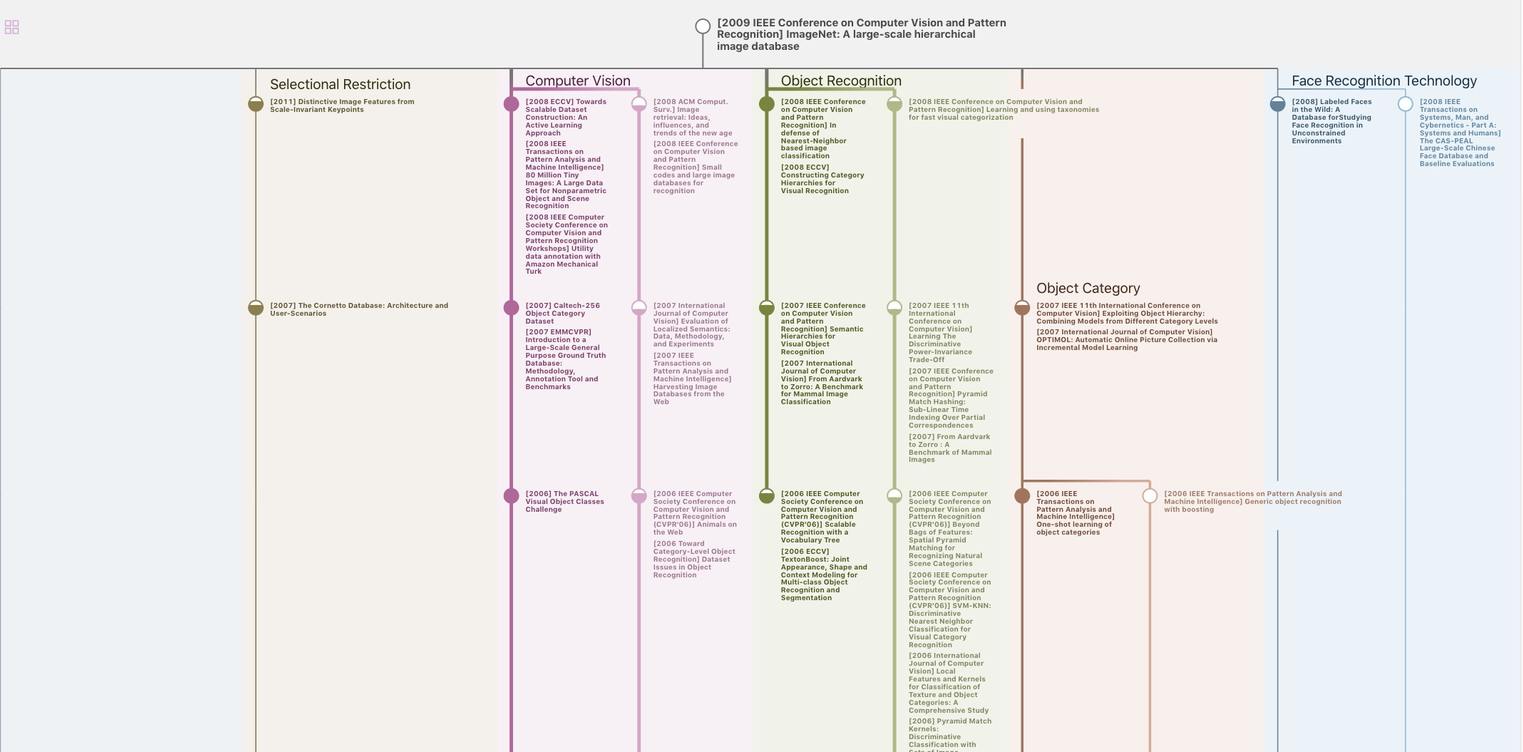
生成溯源树,研究论文发展脉络
Chat Paper
正在生成论文摘要