Semi-supervised consistency regularization for accurate cell type fraction and gene expression estimation
ICLR 2023(2023)
摘要
Abstract Cell deconvolution is the estimation of cell type fractions and cell type-specific gene expression from mixed data with unknown composition. In biomedical research, cell deconvolution, which is a source separation task, is used to obtain mechanistic and diagnostic insights into human disease. An unmet challenge in cell deconvolution, however, is the scarcity of realistic training data and the strong domain shift observed in synthetic training data that is used in contemporary methods. Here, we hypothesize that simultaneous consistency regularization of the target and training domains will improve deconvolution performance. By adding this novel biologically motivated consistency loss to two deep learning-based deconvolution algorithms, we achieve state-of-the-art performance on both cell fraction and gene expression estimation. Our method, DISSECT, outperforms competing algorithms across several gene expression datasets and can be easily adapted to deconvolve other biomedical data types, as exemplified by our spatial expression deconvolution experiments.
更多查看译文
关键词
accurate cell type fraction,gene expression,semi-supervised
AI 理解论文
溯源树
样例
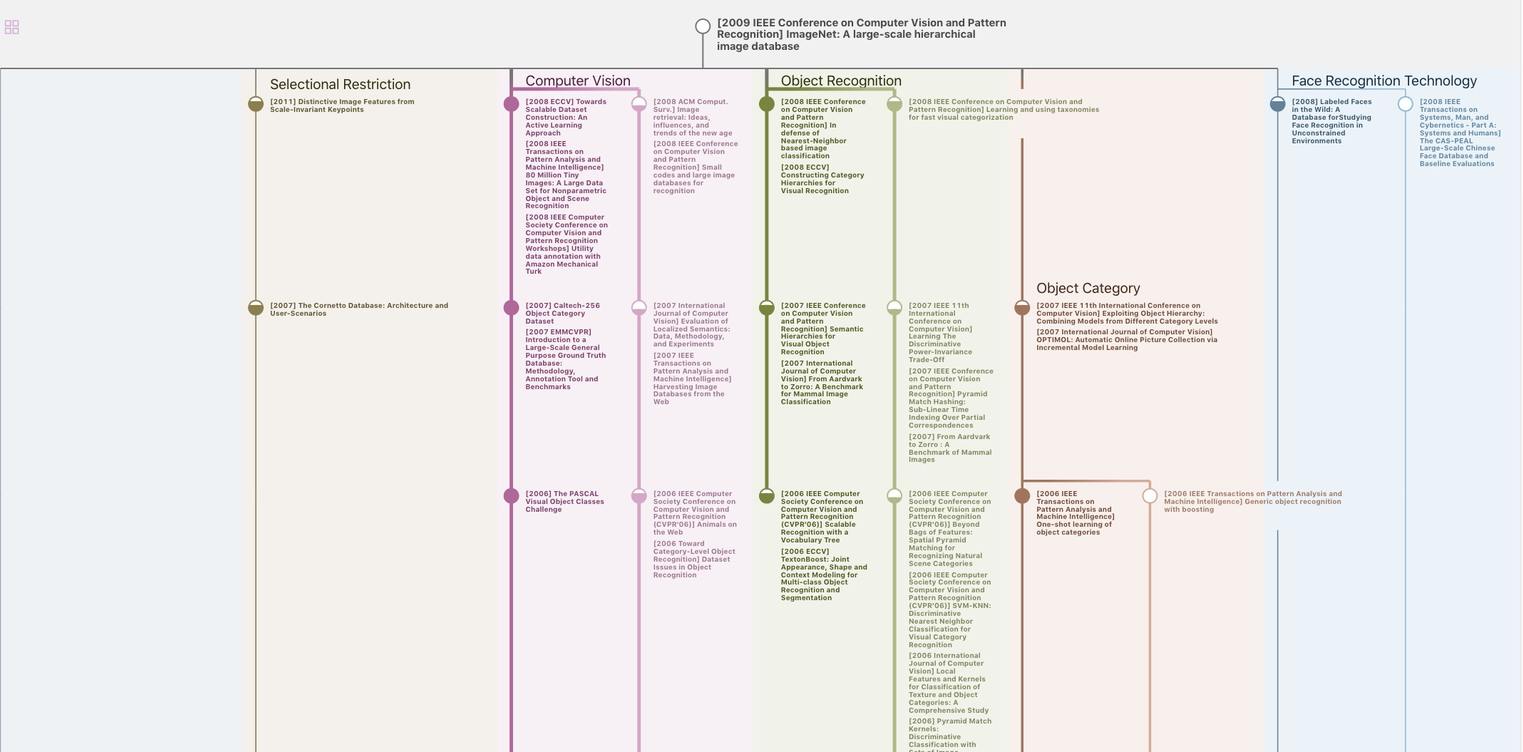
生成溯源树,研究论文发展脉络
Chat Paper
正在生成论文摘要