Evaluation of Active Feature Acquisition Methods under Missing Data
ICLR 2023(2023)
摘要
Machine learning (ML) methods generally assume the full set of features are available at no cost. If the acquisition of a certain feature is costly at run-time, one might want to balance the acquisition cost and the predictive value of the feature for the ML task. The task of training an AI agent to decide which features are necessary to be acquired is called active feature acquisition (AFA). Current AFA methods, however, are challenged when the AFA agent has to be trained/tested with datasets that contain missing data. We formulate, for the first time, the problem of active feature acquisition performance evaluation (AFAPE) under missing data, i.e. the problem of adjusting for the inevitable missingness distribution shift between train/test time and run-time. We first propose a new causal graph, the AFA graph, that characterizes the AFAPE problem as an intervention on the reinforcement learning environment used to train AFA agents. Here, we discuss that for handling missing data in AFAPE, the conventional approaches (off-policy reinforcement learning, blocked feature acquisitions, imputation and inverse probability weighting (IPW)) often lead to biased results or are data inefficient. We then propose active feature acquisition importance sampling (AFAIS), a novel estimator that is more data efficient than IPW. We demonstrate the detrimental conclusions to which biased estimators can lead as well as the high data efficiency of AFAIS in multiple experiments using simulated and real-world data under induced MCAR, MAR and MNAR missingness.
更多查看译文
关键词
Active feature acquisition,active sensing,missing data,reinforcement learning,distribution-shift,off-environment policy evaluation,AFA graph,AFAPE,AFAIS,MCAR,MAR,MNAR,causal inference
AI 理解论文
溯源树
样例
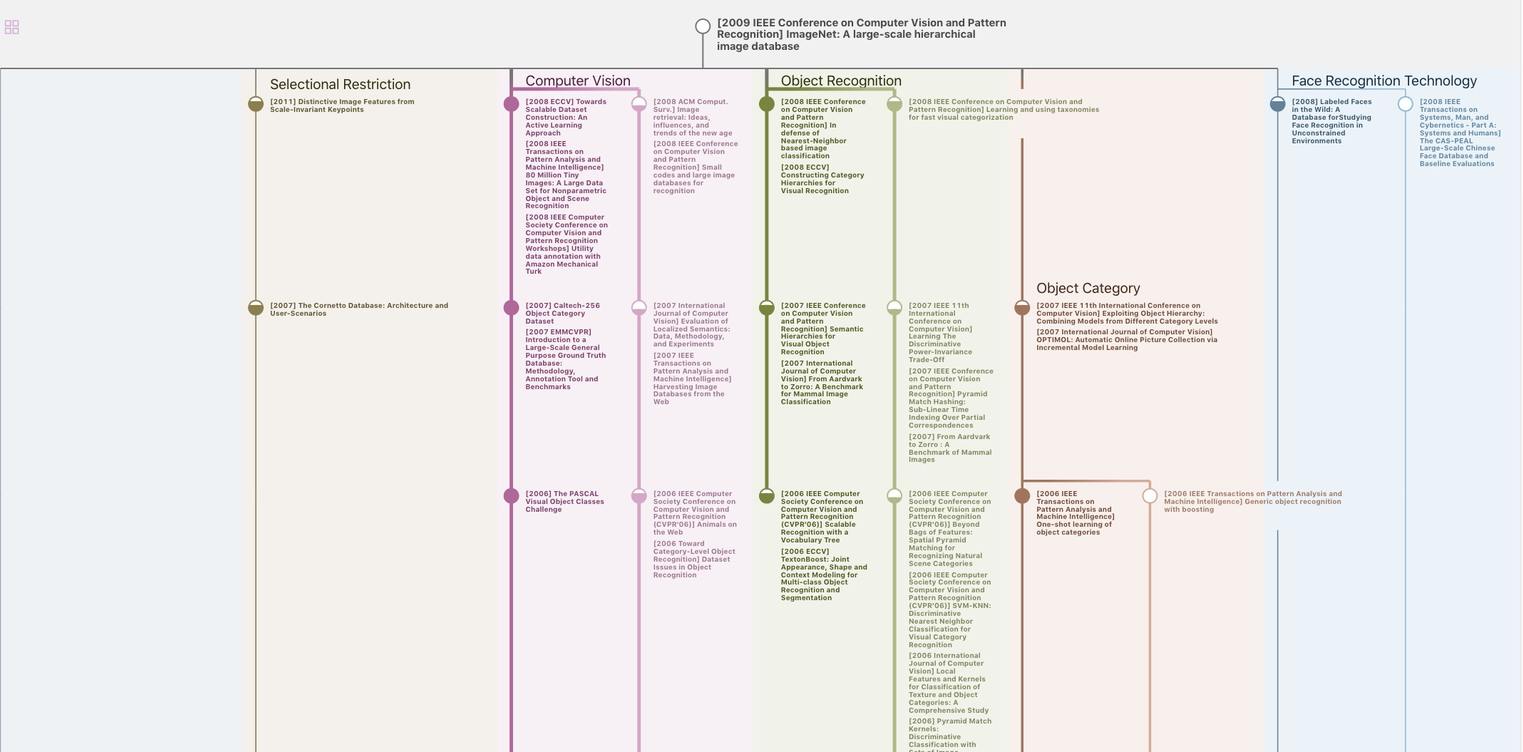
生成溯源树,研究论文发展脉络
Chat Paper
正在生成论文摘要