Variational Classification
ICLR 2023(2023)
摘要
Classification tasks, ubiquitous across machine learning, are commonly tackled by a suitably designed neural network with a softmax output layer, mapping each data point to a categorical distribution over class labels.
We extend this familiar model from a latent variable perspective to variational classification (VC), analogous to how the variational auto-encoder relates to its deterministic counterpart. We derive a training objective based on the ELBO together with an \textit{adversarial} approach for optimising it.
Within this framework, we identify design choices made implicitly in off-the-shelf softmax functions and can instead include domain-specific assumptions, such as class-conditional latent priors. We demonstrate benefits of the VC model in image classification. We show on several standard datasets, that treating inputs to the softmax layer as latent variables under a mixture of Gaussians prior, improves several desirable aspects of a classifier, such as prediction accuracy, calibration, out-of-domain calibration and adversarial robustness.
更多查看译文
关键词
Latent priors,classification
AI 理解论文
溯源树
样例
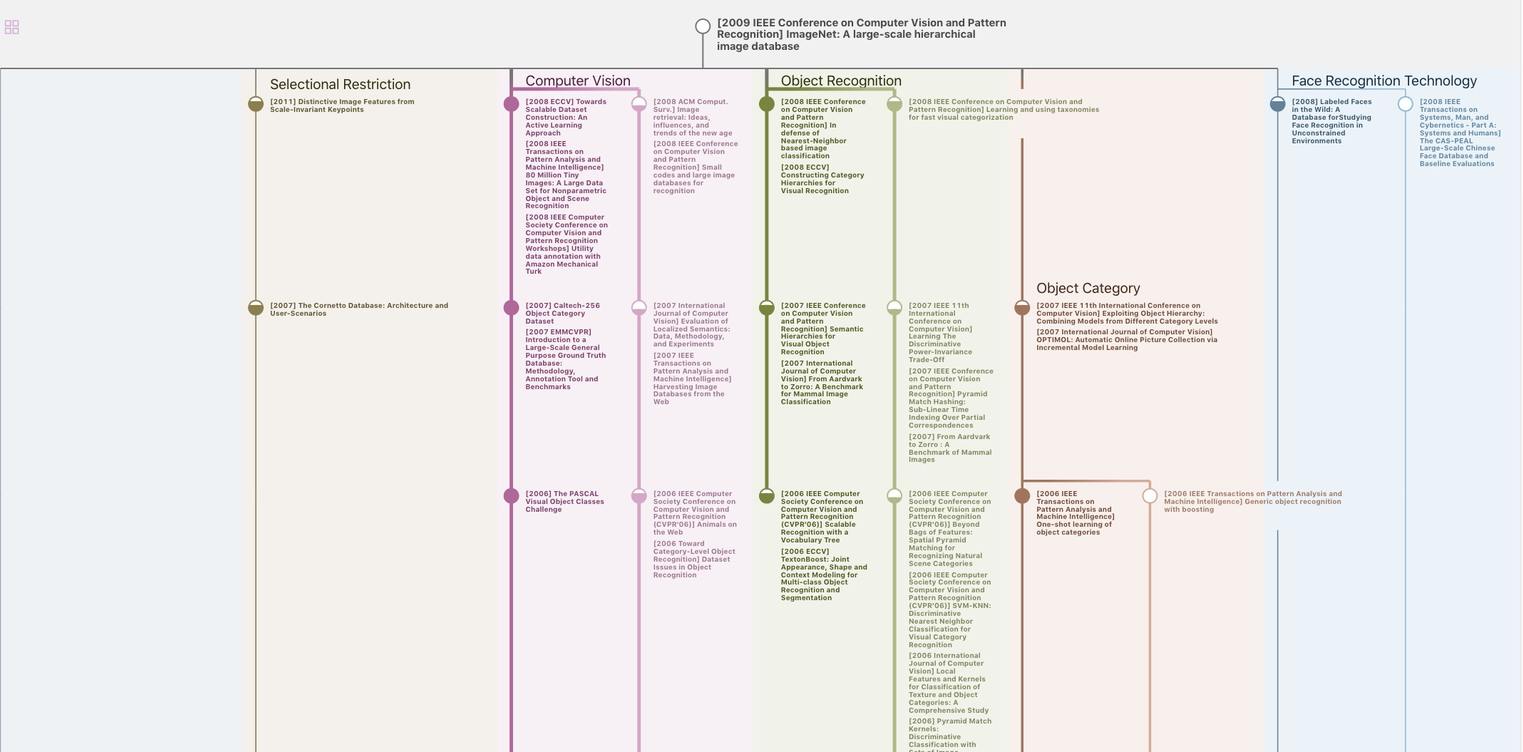
生成溯源树,研究论文发展脉络
Chat Paper
正在生成论文摘要