Is end-to-end learning enough for fitness activity recognition?
ICLR 2023(2023)
摘要
End-to-end learning has taken hold of many computer vision tasks, in particular, related to still images, with task-specific optimization yielding very strong performance. Nevertheless, human-centric action recognition is still largely dominated by hand-crafted pipelines, and only individual components are replaced by neural networks that typically operate on individual frames. As a testbed to study the relevance of such pipelines, we present a new fully annotated video dataset of fitness activities. Any recognition capabilities in this domain are almost exclusively a function of human poses and their temporal dynamics, so pose-based solutions should perform well. We show that, with this labelled data, end-to-end learning on raw pixels can compete with state-of-the-art action recognition pipelines based on pose estimation. We also show that end-to-end learning can support temporally fine-grained tasks such as real-time repetition counting.
更多查看译文
关键词
end-to-end learning,action recognition,3D convolution,video,fitness
AI 理解论文
溯源树
样例
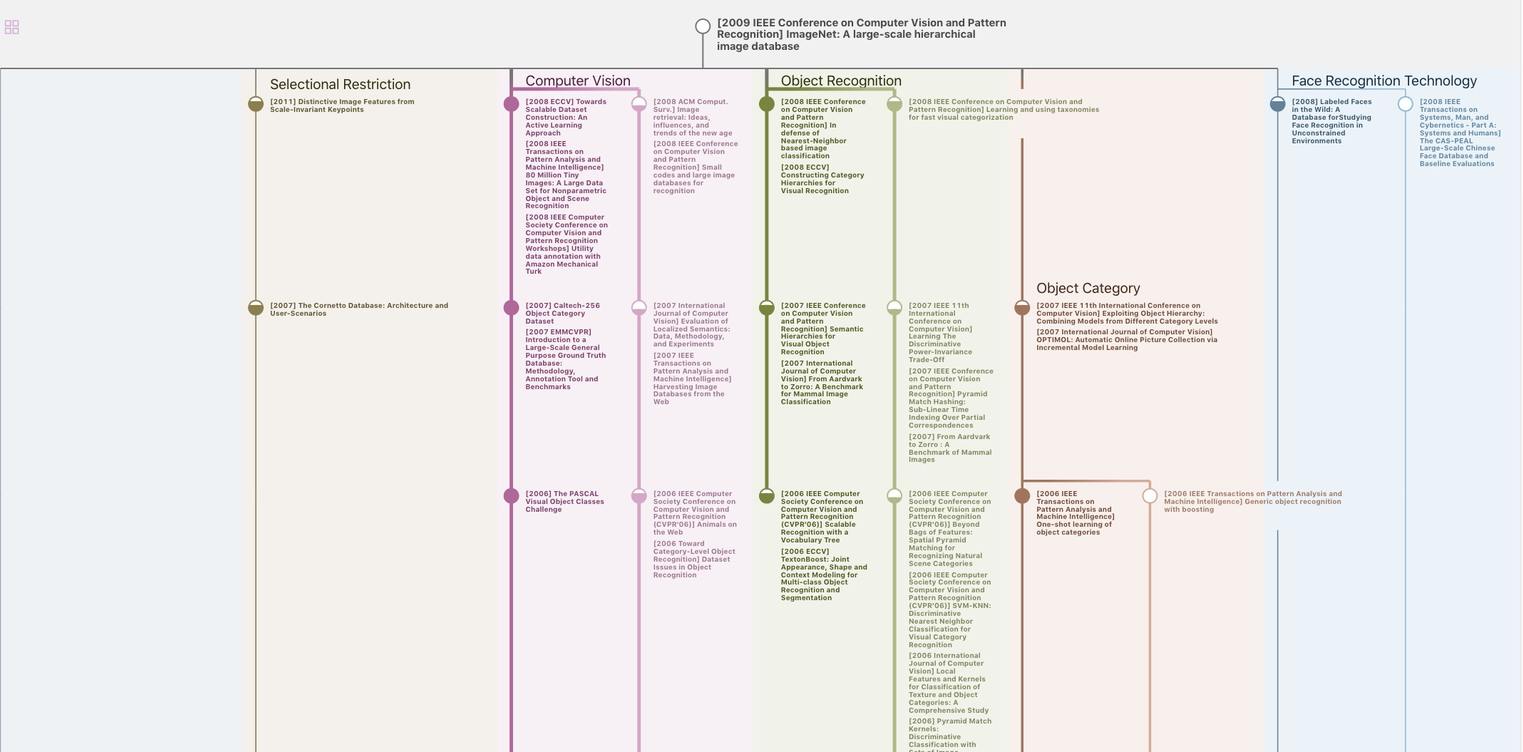
生成溯源树,研究论文发展脉络
Chat Paper
正在生成论文摘要