Variational Pseudo Labels for Meta Test-time Adaptation
ICLR 2023(2023)
摘要
Test-time model adaptation has shown great effectiveness in generalizing over domain shifts. A most successful tactic for test-time adaptation conducts further optimization on the target data using the predictions by the source-trained model. However, due to domain shifts, the source-trained model predictions themselves can be largely inaccurate, which results in a model misspecified to the target data and therefore damages their adaptation ability. In this paper, we address test-time adaptation from a probabilistic perspective. We formulate model adaption as a probabilistic inference problem, which incorporates the uncertainty into source model predictions by modeling pseudo labels as distributions. Based on the probabilistic formalism, we propose variational pseudo labels that explore the information of neighboring target samples to improve pseudo labels and achieve a model better specified to target data. By a meta-learning paradigm, we train our model by simulating domain shifts and the test-time adaptation procedure. In doing so, our model learns the ability to generate more accurate pseudo-label distributions and to adapt to new domains. Experiments on three widely used datasets demonstrate the effectiveness of our proposal.
更多查看译文
关键词
Test-time adaptation,probabilistic framework,variational pseudo label,meta learning
AI 理解论文
溯源树
样例
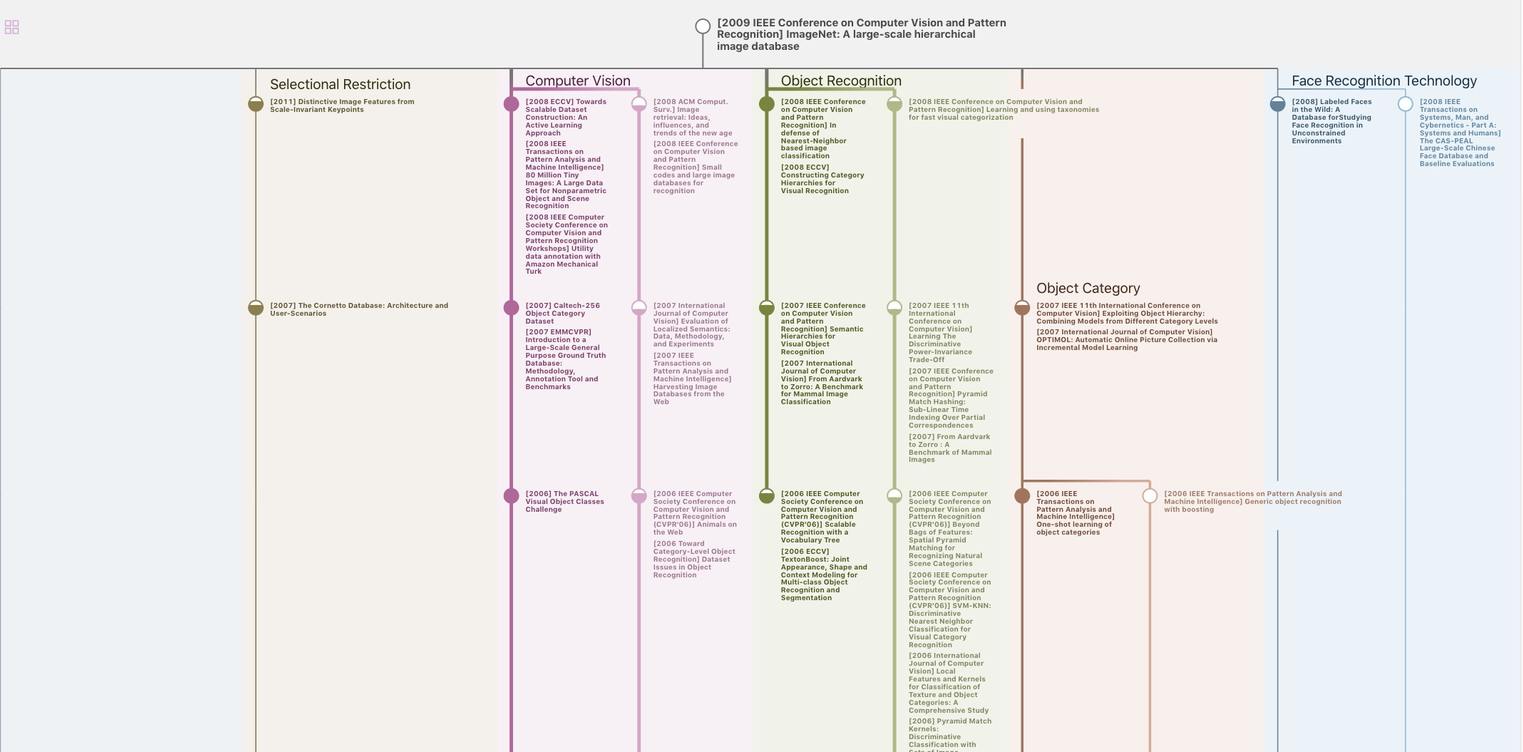
生成溯源树,研究论文发展脉络
Chat Paper
正在生成论文摘要