Global-Scale Species Mapping From Crowdsourced Data
ICLR 2023(2023)
摘要
Estimating the geographical range of a species from in situ observational data is a challenging and important geospatial prediction problem. Given a set of locations indicating where a species has been observed, the goal is to learn a model that can predict how likely it is for the species to be present at any other location. While this is a well-studied problem, traditional approaches are unable to take advantage of more recently available large-scale datasets that cover many locations and species. We propose a new approach that jointly estimates the geographical ranges of tens of thousands of different species simultaneously. We develop a series of benchmark evaluation tasks that measure different aspects of the species range and spatial representation learning problems. We show that our approach scales both in terms of amount of training data and species, where adding more data enables the models to learn better spatial representations that generalize to other species. Despite being only trained on weakly supervised crowdsourced data, our models can approach the predictions of current expert-developed gold standard models.
更多查看译文
关键词
species distribution modeling,coordinate networks,deep learning
AI 理解论文
溯源树
样例
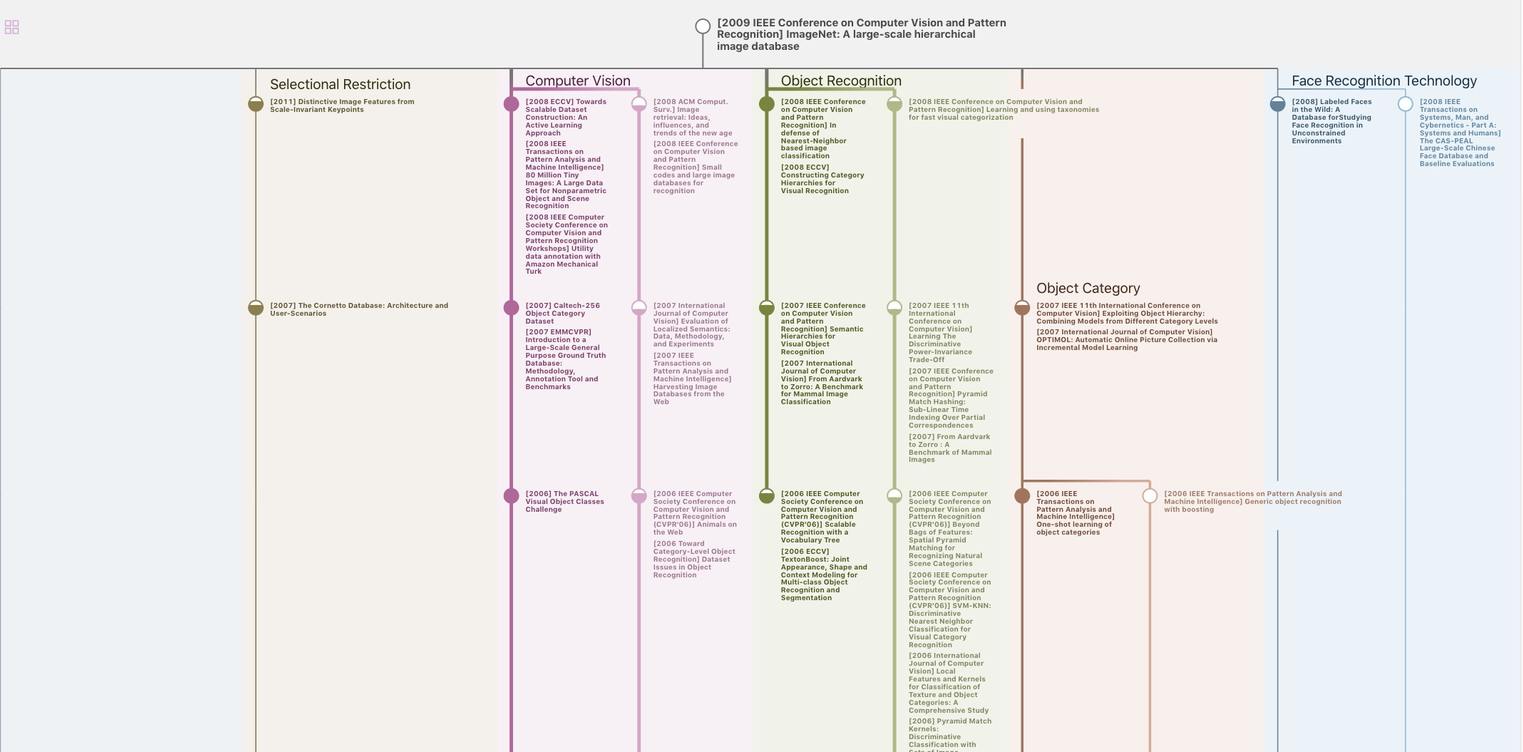
生成溯源树,研究论文发展脉络
Chat Paper
正在生成论文摘要