Adversarial Text to Continuous Image Generation
ICLR 2023(2024)
摘要
Implicit Neural Representations (INR) provide a natural way to parametrize images as a continuous signal, using an MLP that predicts the RGB color at an (x, y) image location. Recently, it has been demonstrated that high-quality INR-decoders can be designed and integrated with Generative Adversarial Networks (GANs) to facilitate unconditional continuous image generation, that are no longer bounded to a spatial resolution. In this paper, we introduce HyperCGAN, a conceptually simple approach for Adversarial Text to Continuous Image Generation based on HyperNetworks, which are networks that produce parameters for another network. HyperCGAN utilizes HyperNetworks to condition an INR-based GAN model on text. In this setting, the generator and the discriminator weights are controlled by their corresponding HyperNetworks, which modulate weight parameters using the provided text query. We propose an effective Word-level hyper-modulation Attention operator, termed WhAtt, which encourages grounding words to independent pixels at input (x, y) coordinates. To the best of our knowledge, our work is the first that explores text-controllable continuous image generation. We conduct comprehensive experiments on the COCO 256x256, CUB 256x256, and the ArtEmis 256x256 benchmark which we introduce in this paper. HyperCGAN improves the performance of text-controllable image generators over the baselines while significantly reducing the gap between text-to-continuous and text-to-discrete image synthesis. Additionally, we show that HyperCGAN, when conditioned on text, retains the desired properties of continuous generative models (e.g., extrapolation outside of image boundaries, accelerated inference of low-
resolution images, out-of-the-box superresolution).
更多查看译文
关键词
gan,generative modelling,text-to-image,text2image,hypernetworks
AI 理解论文
溯源树
样例
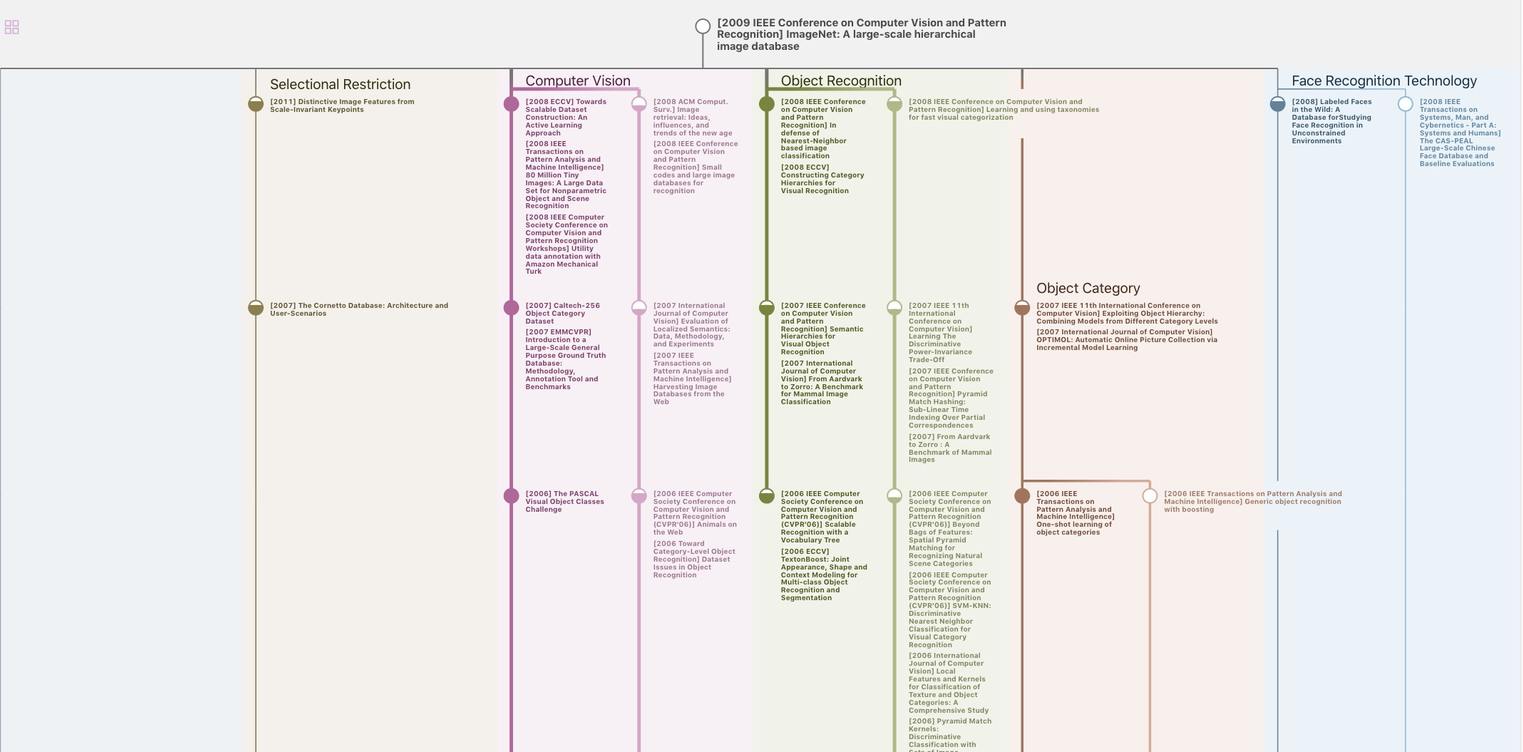
生成溯源树,研究论文发展脉络
Chat Paper
正在生成论文摘要