Thinking fourth dimensionally: Treating Time as a Random Variable in EBMs
ICLR 2023(2023)
摘要
Recent years have seen significant progress in techniques for learning high-dimensional distributions. Many modern methods, from diffusion models to Energy-Based-Models (EBMs), adopt a coarse-to-fine approach. This is often done by introducing a series of auxiliary distributions that gradually change from the data distribution to some simple distribution (e.g. white Gaussian noise). Methods in this category separately learn each auxiliary distribution (or transition between pairs of consecutive distributions) and then use the learned models sequentially to generate samples. In this paper, we offer a simple way to generalize this idea by treating the ``time'' index of the series as a random variable and framing the problem as that of learning a single joint distribution of "time" and samples. We show that this joint distribution can be learned using any existing EBM method and that it allows achieving improved results. As an example, we demonstrate this approach using contrastive divergence (CD) in its most basic form. On CIFAR-10 and CelebA ($32\times 32$), this method outperforms previous CD-based methods in terms of inception and FID scores.
更多查看译文
关键词
energy-based models,markov chain mote carlo,contrastive divergence
AI 理解论文
溯源树
样例
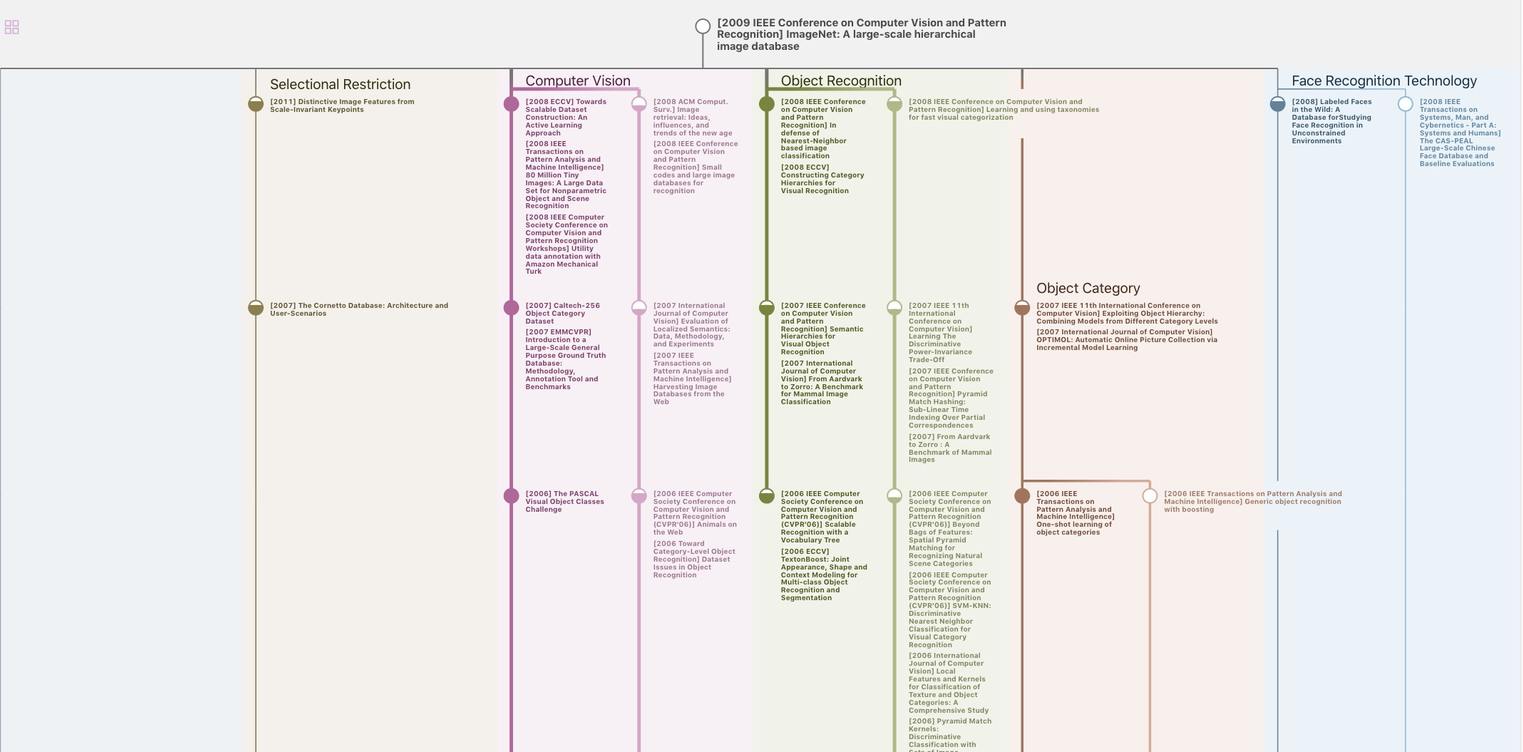
生成溯源树,研究论文发展脉络
Chat Paper
正在生成论文摘要