Learning from conflicting data with hidden contexts
ICLR 2023(2023)
摘要
Classical supervised learning assumes a stable relation between inputs and outputs. However, this assumption is often invalid in real-world scenarios where the input-output relation in the data depends on some hidden contexts. We formulate a more general setting where the training data is sampled from multiple unobservable domains, while different domains may possess semantically distinct input-output maps. Training data exhibits inherent conflict in this setting, rendering vanilla empirical risk minimization problematic. We propose to tackle this problem by introducing an allocation function that learns to allocate conflicting data to different prediction models, resulting in an algorithm that we term LEAF. We draw an intriguing connection between our approach and a variant of the Expectation-Maximization algorithm. We provide theoretical justifications for LEAF on its identifiability, learnability, and generalization error. Empirical results demonstrate the efficacy and potential applications of LEAF in a range of regression and classification tasks on both synthetic data and real-world datasets.
更多查看译文
关键词
Conflicting data,hidden contexts,subjective learning,multi-domain learning
AI 理解论文
溯源树
样例
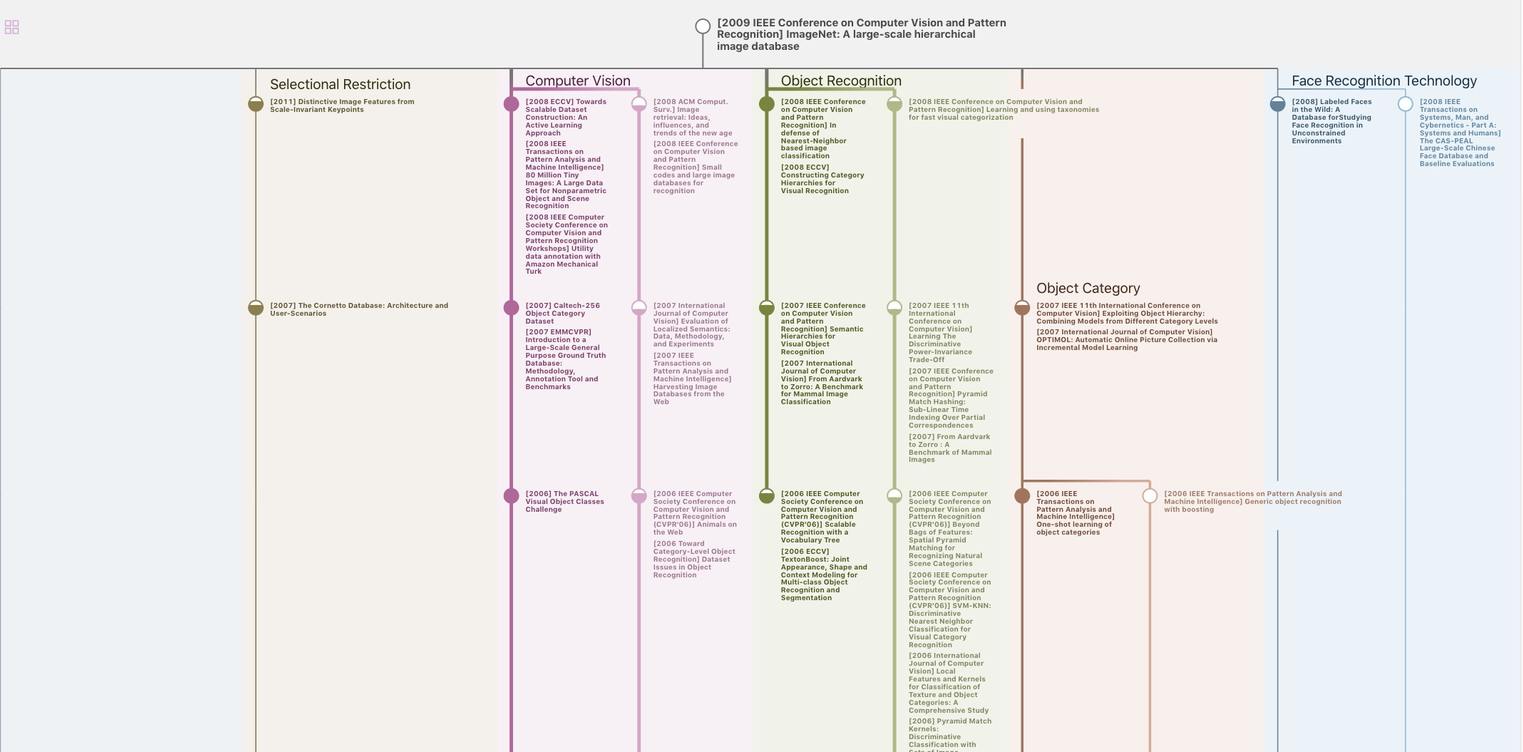
生成溯源树,研究论文发展脉络
Chat Paper
正在生成论文摘要