Stochastic Bridges as Effective Regularizers for Parameter-Efficient Tuning
conf_acl(2023)
摘要
Parameter-efficient tuning methods (PETs) have achieved promising results in tuning large pre-trained language models (PLMs). By formalizing frozen PLMs and additional tunable parameters as systems and controls respectively, PETs can be theoretically grounded to optimal control and further viewed as optimizing terminal cost and running cost in the optimal control literature. Despite the elegance of this theoretical grounding, in practice, existing PETs often ignore the running cost and only optimize the terminal cost, i.e., focus on optimizing the loss function of the output state, regardless of the running cost that depends on the intermediate states. Since it is non-trivial to directly model the intermediate states and design a running cost function, we propose to use latent stochastic bridges to regularize the intermediate states and serve as the running cost of PETs. As the first work to propose regularized PETs that use stochastic bridges as the regularizers (running costs) for intermediate states, we show the effectiveness and generality of this regularization across different tasks, PLMs and PETs. In view of the great potential and capacity, we believe more sophisticated regularizers can be designed for PETs and better performance can be achieved in the future.
更多查看译文
关键词
parameter-efficient tuning,pre-trained model,stochastic process
AI 理解论文
溯源树
样例
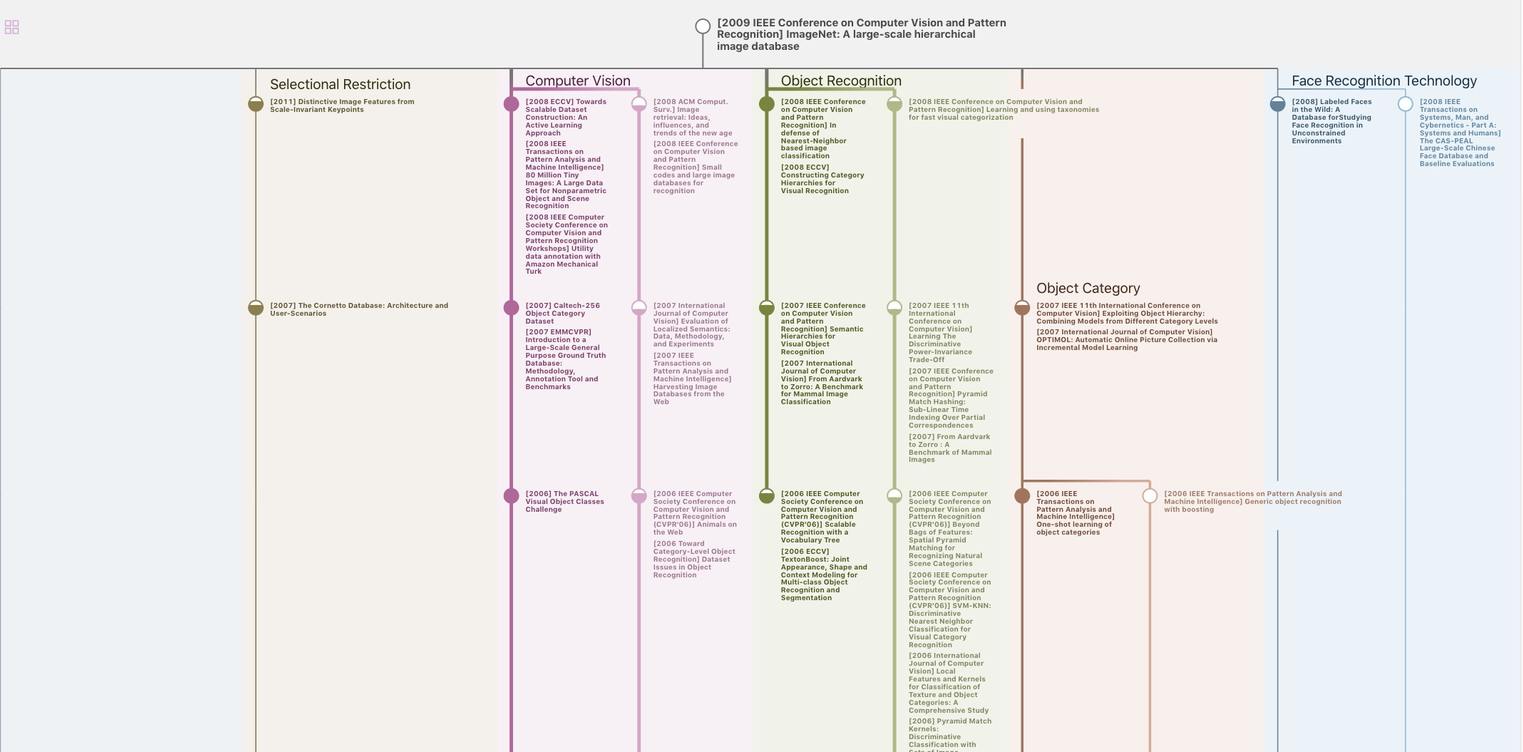
生成溯源树,研究论文发展脉络
Chat Paper
正在生成论文摘要