Precision Collaboration for Federated Learning
ICLR 2023(2023)
摘要
Inherent heterogeneity of local data distributions, which causes inefficient model learning and significant degradation of model performance, has been a key challenge in Federated Learning (FL). So far, plenty of efforts have focused on addressing data heterogeneity by relying on a hypothetical clustering structure or a consistent information sharing mechanism. However, because of the diversity of the real-world local data, these assumptions may be largely violated. In this work, we argue that information sharing is mostly fragmented in the federated network in reality. More specifically, the distribution overlaps are not consistent but scattered in local clients. In this work, we propose the concept ``Precision Collaboration'' which refers to learning from the informative overlaps precisely while avoiding the potential negative transfer induced by others. In particular, we propose to infer the local data manifolds and estimate the exact local data density simultaneously. The learned manifold aims to precisely identify the overlaps from other clients, and the estimated likelihood allows to generate samples from the manifold in an optimal sampling density. Experiments show that our proposed PCFL significantly overcomes baselines on benchmarks and a real-world clinical scenario.
更多查看译文
关键词
federated learning,personalized federated learning
AI 理解论文
溯源树
样例
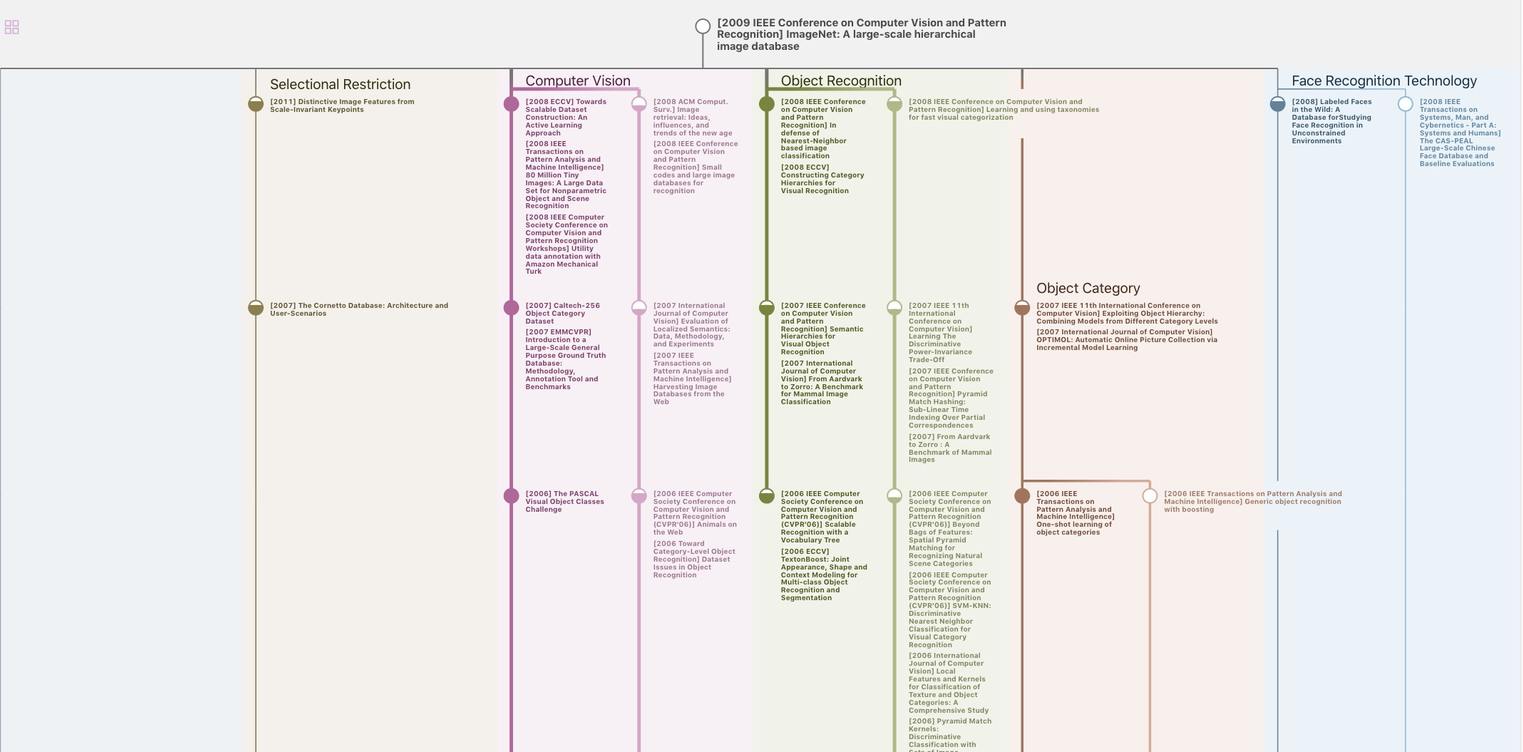
生成溯源树,研究论文发展脉络
Chat Paper
正在生成论文摘要