Exp-$\alpha$: Beyond Proportional Aggregation in Federated Learning
ICLR 2023(2023)
Abstract
Federated Learning (FL) is a distributed learning paradigm, which computes gradients of a model locally on different clients and aggregates the updates to construct a new model collectively. Typically, the updates from local clients are aggregated with weights proportional to the size of clients' local datasets. In practice, clients have different local datasets suffering from data heterogeneity, such as imbalance. Although proportional aggregation still theoretically converges to the global optimum, it is provably slower when non-IID data is present (under convexity assumptions), the effect of which is exacerbated in practice. We posit that this analysis ignores convergence rate, which is especially important under such settings in the more realistic non-convex real world. To account for this, we analyze a generic and time-varying aggregation strategy to reveal a surprising trade-off between convergence rate and convergence error under convexity assumptions. Inspired by the theory, we propose a new aggregation strategy, Exp-$\alpha$, which weights clients differently based on their severity of data heterogeneity. It achieves stronger convergence rates at the theoretical cost of a non-vanishing convergence error. Through a series of controlled experiments, we empirically demonstrate the superior convergence behavior (both in terms of rate and, in practice, even error) of the proposed aggregation on three types of data heterogeneity: imbalance, label-flipping, and domain shift when combined with existing FL algorithms. For example, on our imbalance benchmark, Exp-$\alpha$, combined with FedAvg, achieves a relative $12\%$ increase in convergence rate and a relative $3\%$ reduction in error across four FL communication settings.
MoreTranslated text
Key words
Federated Learning
AI Read Science
Must-Reading Tree
Example
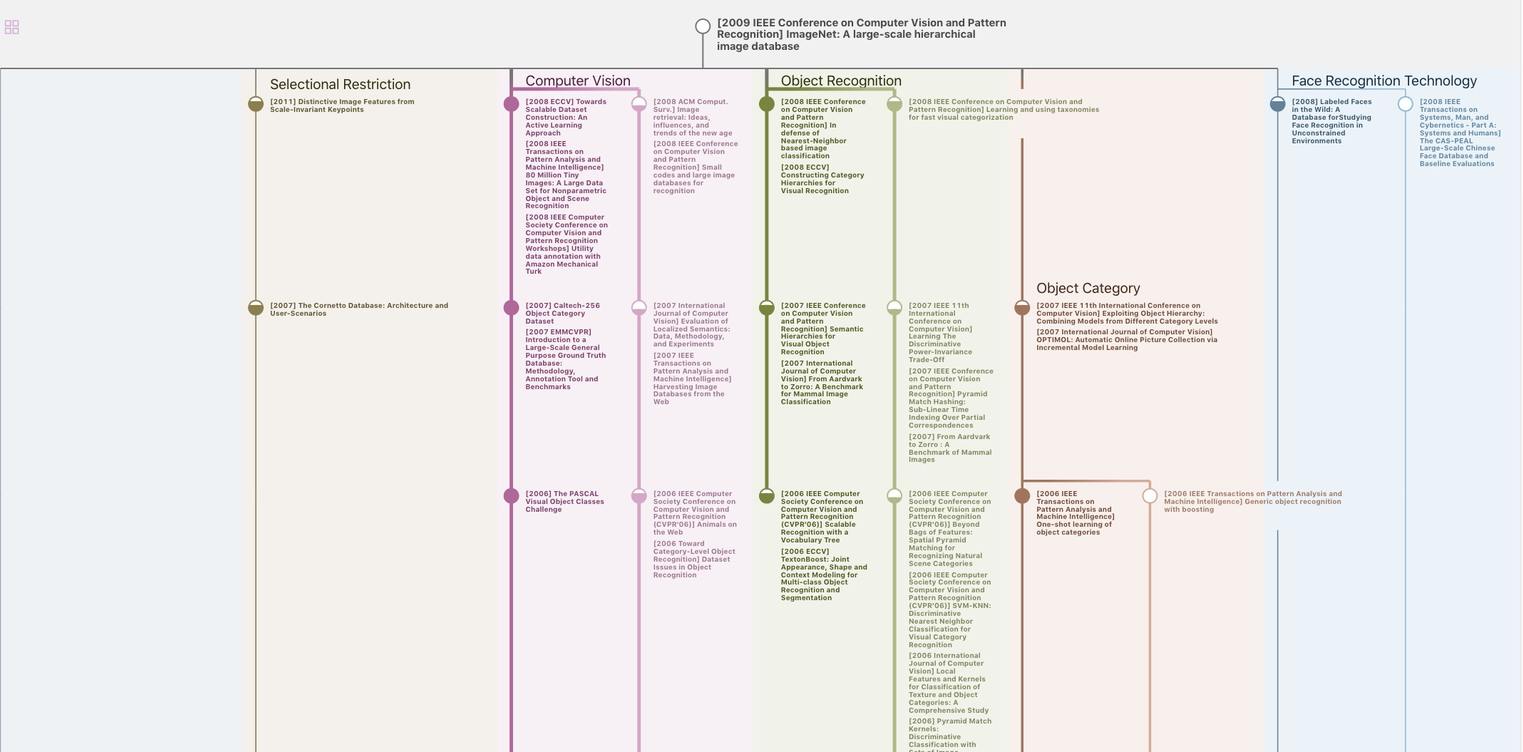
Generate MRT to find the research sequence of this paper
Chat Paper
Summary is being generated by the instructions you defined