Global Counterfactual Explanations Are Reliable Or Efficient, But Not Both
ICLR 2023(2023)
摘要
Counterfactual explanations have been widely studied in explainability, with a range of application dependent methods emerging in fairness, recourse and model understanding. The major shortcoming associated with these methods, however, is their inability to provide explanations beyond the local or instance-level. While many works touch upon the notion of a global explanation, typically suggesting to aggregate masses of local explanations in the hope of ascertaining global properties, few provide frameworks that are both reliable and computationally tractable. Meanwhile, practitioners are requesting more efficient and interactive explainability tools. We take this opportunity to investigate existing methods, improving the efficiency of Actionable Recourse Summaries (AReS), one of the only known global recourse frameworks, and proposing Global & Efficient Counterfactual Explanations (GLOBE-CE), a novel and flexible framework that tackles the scalability issues associated with current state-of-the-art, particularly on higher dimensional datasets and in the presence of continuous features. Furthermore, we provide a unique mathematical analysis of categorical feature translations, utilising it in our method. Experimental evaluation with real world datasets and user studies verify the speed, reliability and interpretability improvements of our framework.
更多查看译文
关键词
Global,counterfactual,explanations,recourse,fairness,efficiency,reliability,black box
AI 理解论文
溯源树
样例
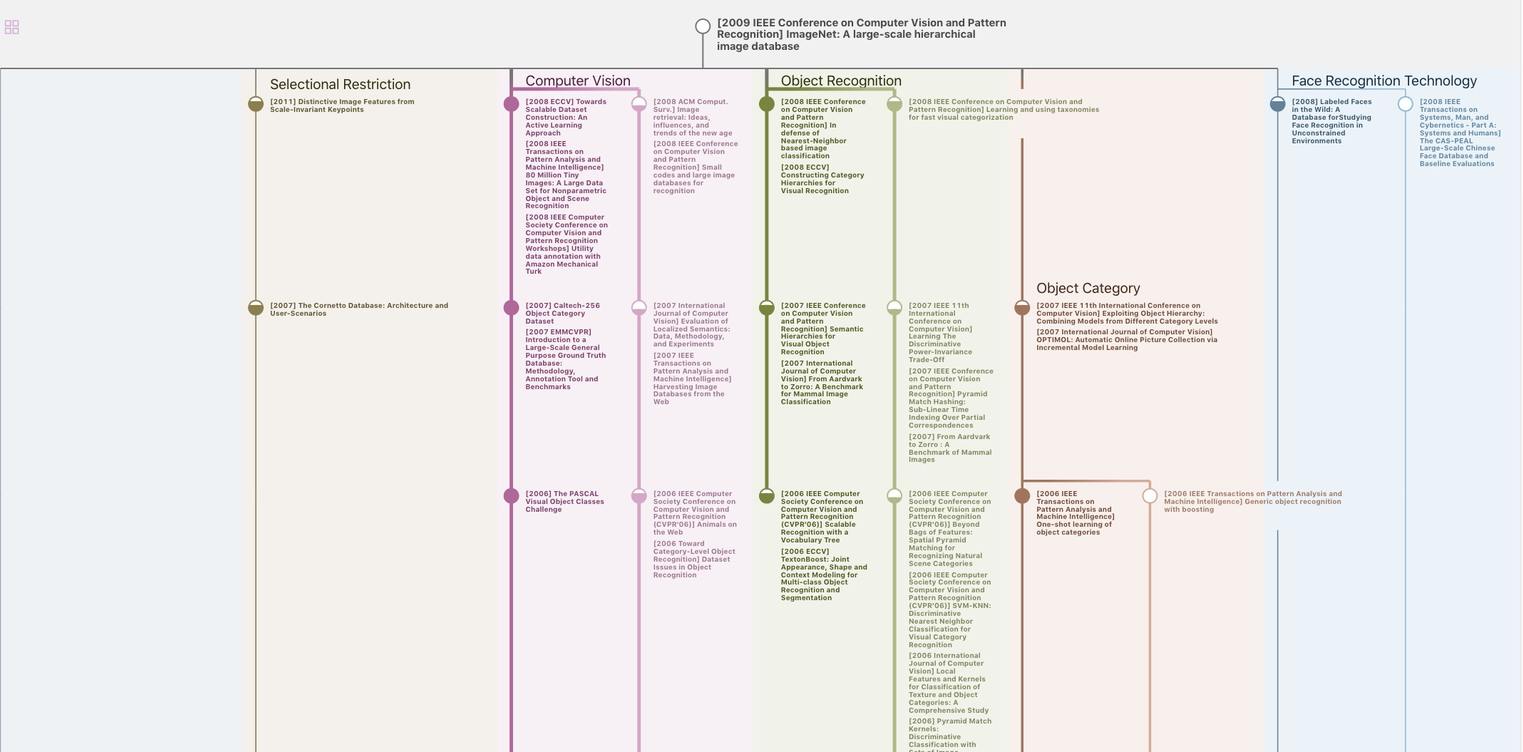
生成溯源树,研究论文发展脉络
Chat Paper
正在生成论文摘要