On The Implicit Bias of Weight Decay in Shallow Univariate ReLU Networks
ICLR 2023(2023)
摘要
We give a complete characterization of the implicit bias of infinitesimal weight decay in the modest setting of univariate one layer ReLU networks. Our main result is a surprisingly simple geometric description of all one layer ReLU networks that exactly fit a dataset $\mathcal D= \set{(x_i,y_i)}$ with the minimum value of the $\ell_2$-norm of the neuron weights. Specifically, we prove that such functions must be either concave or convex between any two consecutive data sites $x_i$ and $x_{i+1}$. Our description implies that interpolating ReLU networks with weak $\ell_2$-regularization achieve the best possible generalization for learning $1d$ Lipschitz functions, up to universal constants.
更多查看译文
关键词
theory,implicit bias,generalization,interpolation,theoretical,shallow ReLU networks,ReLU networks,analysis of weight decay
AI 理解论文
溯源树
样例
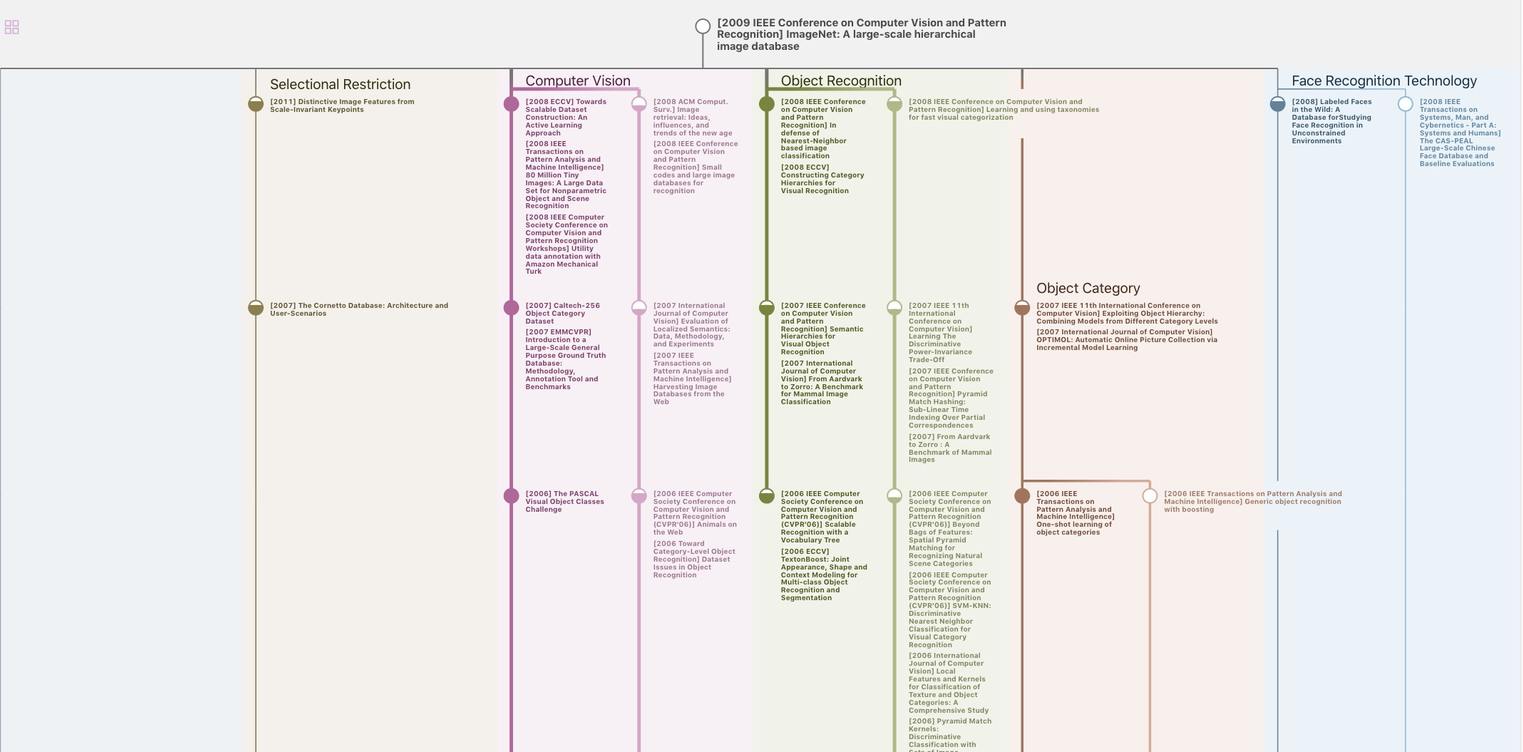
生成溯源树,研究论文发展脉络
Chat Paper
正在生成论文摘要