Learning with Instance-Dependent Label Noise: Balancing Accuracy and Fairness
ICLR 2023(2023)
摘要
Incorrect labels hurt model performance when the model overfits to noise. Many state-of-the-art approaches that address label noise assume that label noise is independent from the input features. In practice, however, label noise is often feature or instance-\textit{dependent}, and therefore is biased (i.e., some instances are more likely to be mislabeled than others). Approaches that ignore this dependence can produce models with poor discriminative performance, and depending on the task, can exacerbate issues around fairness. In light of these limitations, we propose a two-stage approach to learn from datasets with instance-dependent label noise. Our approach utilizes \textit{anchor points}, a small subset of data for which we know the ground truth labels. On many tasks, our approach leads to consistent improvements over the state-of-the-art in discriminative performance (AUROC) while balancing model fairness (area under the equalized odds curve, AUEOC). For example, when predicting acute respiratory failure onset on the MIMIC-III dataset, the harmonic mean of the AUROC and AUEOC of our approach is 0.84 (SD 0.01) while that of the next best baseline is 0.81 (SD 0.01). Overall, our approach leads to learning more accurate and fair models compared to existing approaches in the presence of instance-dependent label noise.
更多查看译文
关键词
noisy labels,supervised learning
AI 理解论文
溯源树
样例
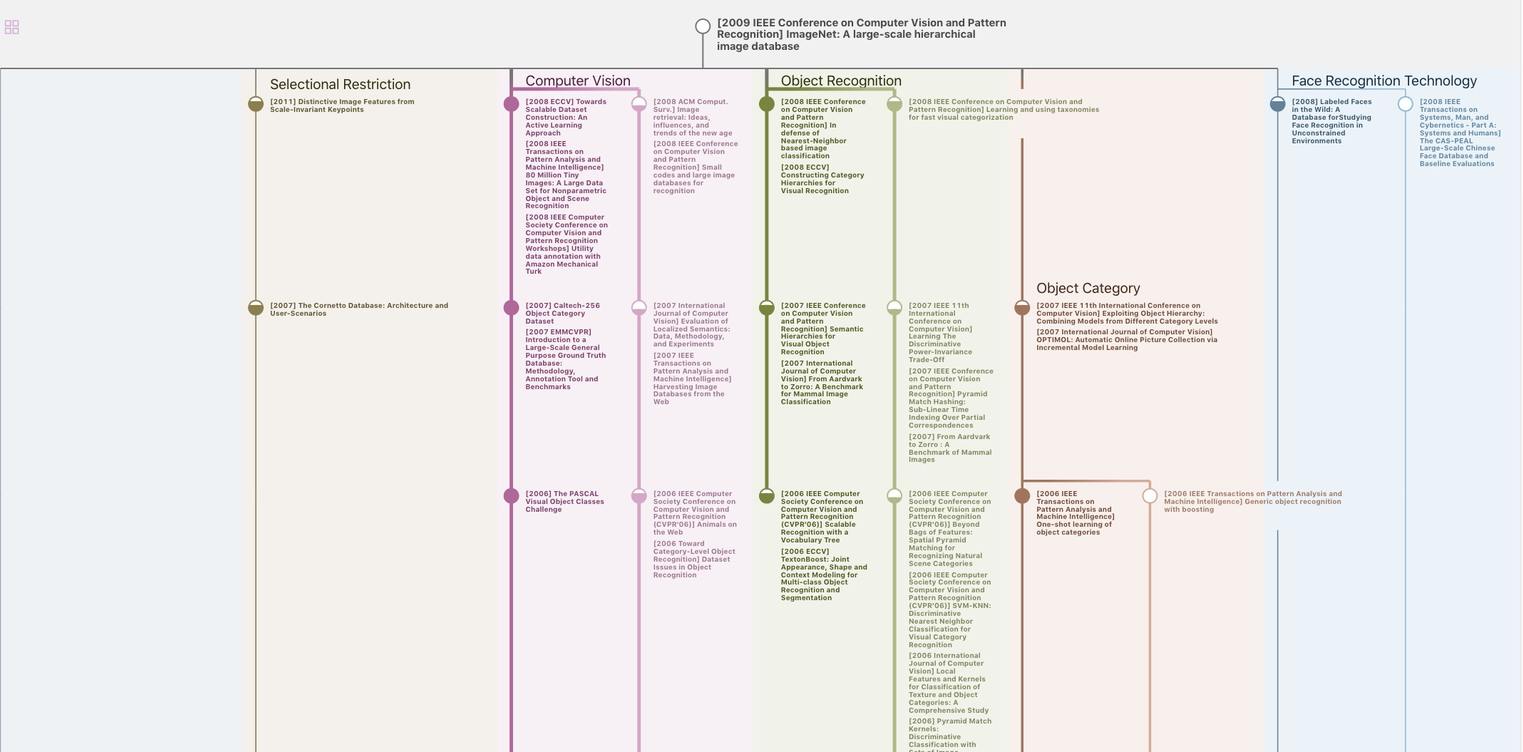
生成溯源树,研究论文发展脉络
Chat Paper
正在生成论文摘要