Reach the Remote Neighbors: Dual-Encoding Transformer for Graphs
ICLR 2023(2023)
摘要
Despite recent successes in natural language processing and computer vision, Transformer suffers from the scalability problem when dealing with graphs. Computing node-to-node attentions is infeasible on complicated graphs, e.g., knowledge graphs. One solution is to consider only the near neighbors, which, however, will lose the key merit of Transformer that attends to the elements at any distance. In this paper, we propose a new Transformer architecture, named dual-encoding Transformer (DET), which has a structural encoder to aggregate information from near neighbors and a semantic encoder to focus on useful semantically close neighbors. The two encoders can be incorporated to boost each other's performance. Our experiments demonstrate that DET achieves superior performance compared to the respective state-of-the-art attention-based methods in dealing with molecules, networks and knowledge graphs.
更多查看译文
关键词
konwledge graph prediction,node classification,graph property prediction,MSA Transformer
AI 理解论文
溯源树
样例
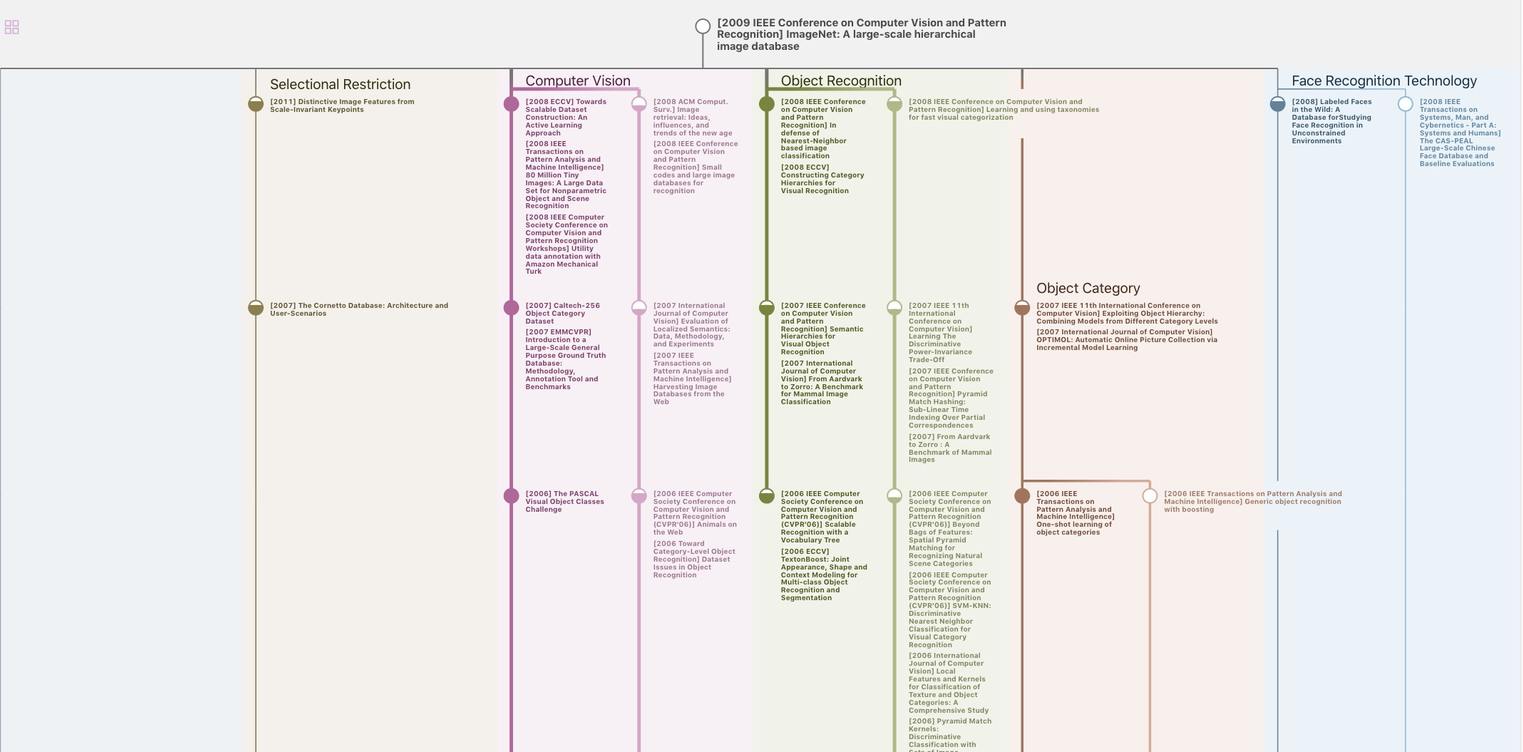
生成溯源树,研究论文发展脉络
Chat Paper
正在生成论文摘要