Evaluating Fairness Without Sensitive Attributes: A Framework Using Only Auxiliary Models
ICLR 2023(2023)
摘要
Although the volume of literature and public attention on machine learning fairness has been growing significantly in recent years, in practice some tasks as basic as measuring fairness, which is the first step in studying and promoting fairness, can be challenging. This is because the sensitive attributes are often unavailable in a machine learning system due to privacy regulations. The straightforward solution is to use auxiliary models to predict the missing sensitive attributes. However, our theoretical analyses show that the estimation error of the directly measured fairness metrics is proportional to the error rates of auxiliary models' predictions. Existing works that attempt to reduce the estimation error often require strong assumptions, e.g. access to the ground-truth sensitive attributes in a subset of samples, auxiliary models' training data and the target data are i.i.d, or some form of conditional independence. In this paper, we drop those assumptions and propose a framework that uses only off-the-shelf auxiliary models. The main challenge is how to reduce the negative impact of imperfectly predicted sensitive attributes on the fairness metrics without knowing the ground-truth sensitive attribute values. Inspired by the noisy label learning literature, we first derive a closed-form relationship between the directly measured fairness metrics and their corresponding ground-truth metrics. And then we estimate some key statistics (most importantly transition matrix in the noisy label literature), which we use, together with the derived relationship, to calibrate the fairness metrics. Our framework can be applied to all popular group fairness definitions as well as multi-class classifiers and multi-category sensitive attributes. In addition, we theoretically prove the upper bound of the estimation error in our calibrated metrics and show our method can substantially decrease the estimation error especially when auxiliary models are inaccurate or the target model is highly biased. Experiments on COMPAS and CelebA validate our theoretical analyses and show our method can measure fairness significantly more accurately than baselines under favorable circumstances.
更多查看译文
关键词
Fairness evaluation,noise transition matrix,sensitive attributes
AI 理解论文
溯源树
样例
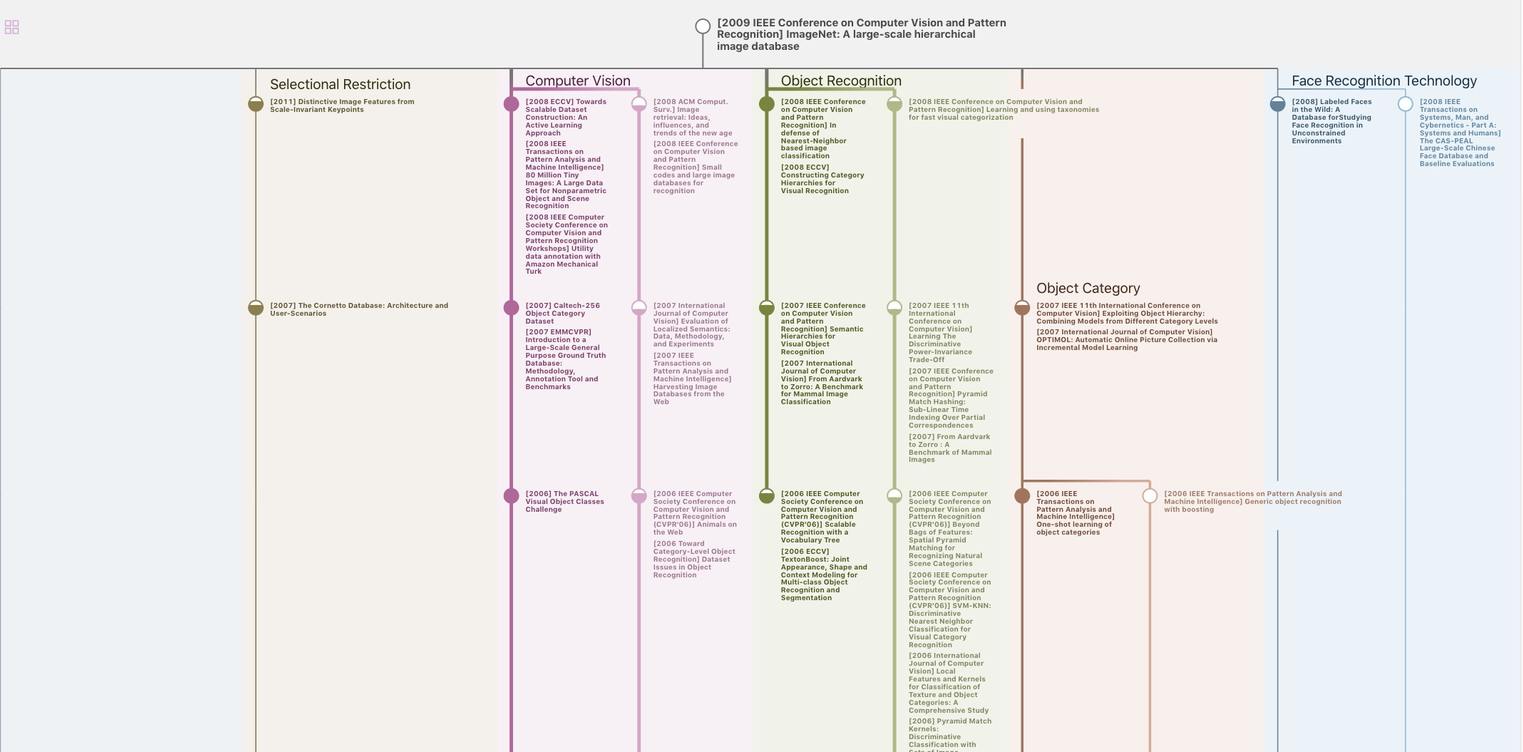
生成溯源树,研究论文发展脉络
Chat Paper
正在生成论文摘要