Do Not Train It: A Linear Neural Architecture Search of Graph Neural Networks
ICLR 2023(2023)
摘要
Neural architecture search (NAS) for Graph neural networks (GNNs), called NAS-GNNs, has achieved significant performance over manually designed GNN architectures. However, these methods inherit issues from the conventional NAS methods, such as high computational cost and optimization difficulty. More importantly, previous NAS methods have ignored the uniqueness of GNNs, where the non-linearity has limited effect. Based on this, we are the first to theoretically prove that a GNN fixed with random weights can obtain optimal outputs under mild conditions. With the randomly-initialized weights, we can then seek the optimal architecture parameters via the sparse coding objective and derive a novel NAS-GNNs method, namely neural architecture coding (NAC). Consequently, our NAC holds a no-update scheme on GNNs and can efficiently compute in linear time. Empirical evaluations on multiple GNN benchmark datasets demonstrate that our approach leads to state-of-the-art performance, which is up to $200\times$ faster and $18.8\%$ more accurate than the strong baselines.
更多查看译文
关键词
Neural Architecture Search,Graph neural network,Automated Machine Learning
AI 理解论文
溯源树
样例
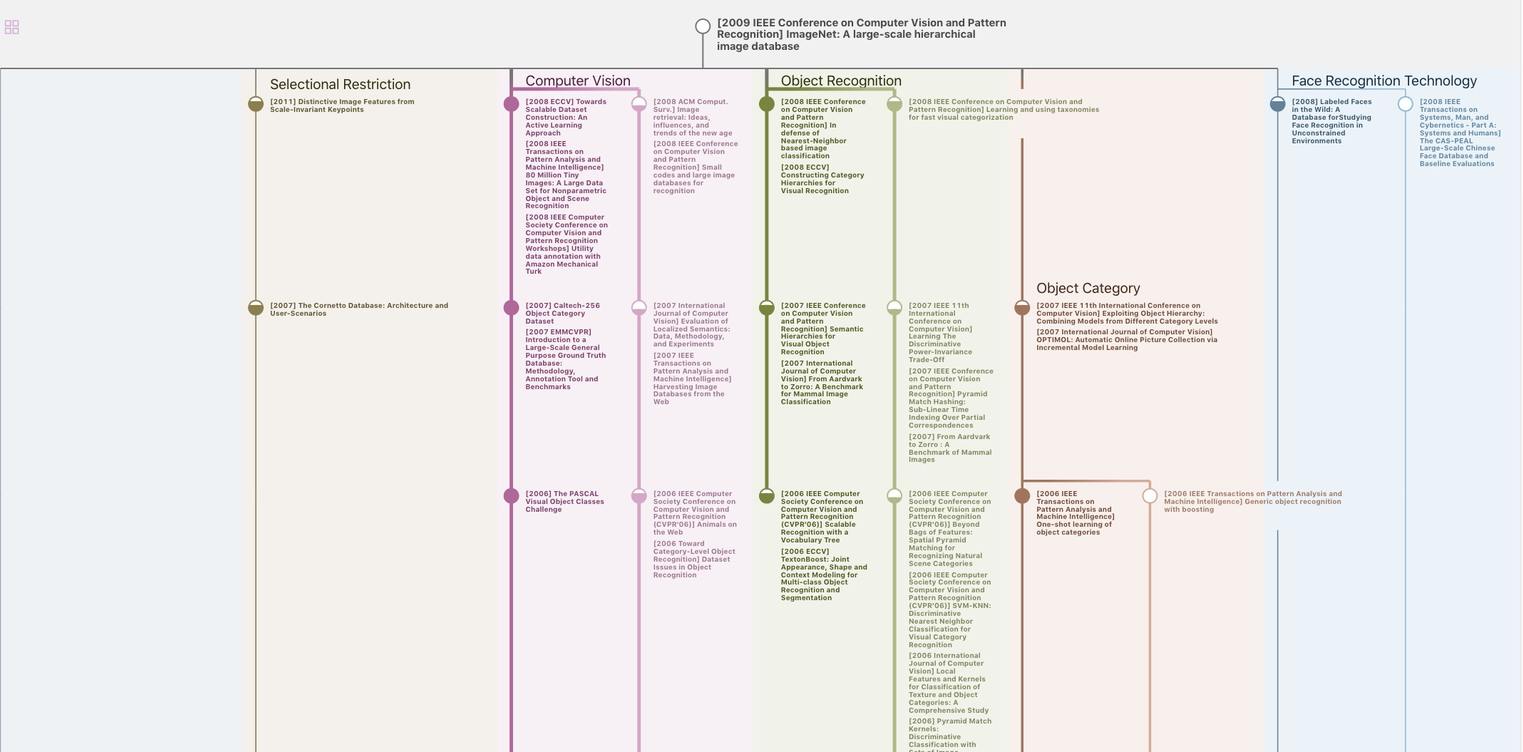
生成溯源树,研究论文发展脉络
Chat Paper
正在生成论文摘要