Robustness Exploration of Semantic Information in Adversarial Training
ICLR 2023(2023)
摘要
In this paper, we look into the problem of adversarial robustness from the semantic information perspective. We demonstrate a novel insight that adversarial attacks destroy the correlation between visual representations and semantic word vectors, and adversarial training fixed it. We further find that the correlation between robust features of different categories is consistent with the correlation between corresponding semantic word vectors. Based on that, we introduce the semantic information to assist model training and propose Semantic Constraint Adversarial Robust Learning (SCARL). First, we follow an information-theoretical lens to formulate the mutual information between the visual representation and the corresponding semantic word vector in the embedding space to bridge the information gap. We further provide a differentiable lower bound to optimize such mutual information efficiently. Second, we propose a novel semantic structural constraint, encouraging the trained model to keep the structure of visual representations consistent with that of semantic word vectors. Finally, we combine these two techniques with adversarial training to learn robust visual representation. Experimentally, we conduct extensive experiments on several benchmarks, demonstrating that semantic information is indeed beneficial to model robustness.
更多查看译文
关键词
Adversarial training,Semantic information,Adversarial robustness
AI 理解论文
溯源树
样例
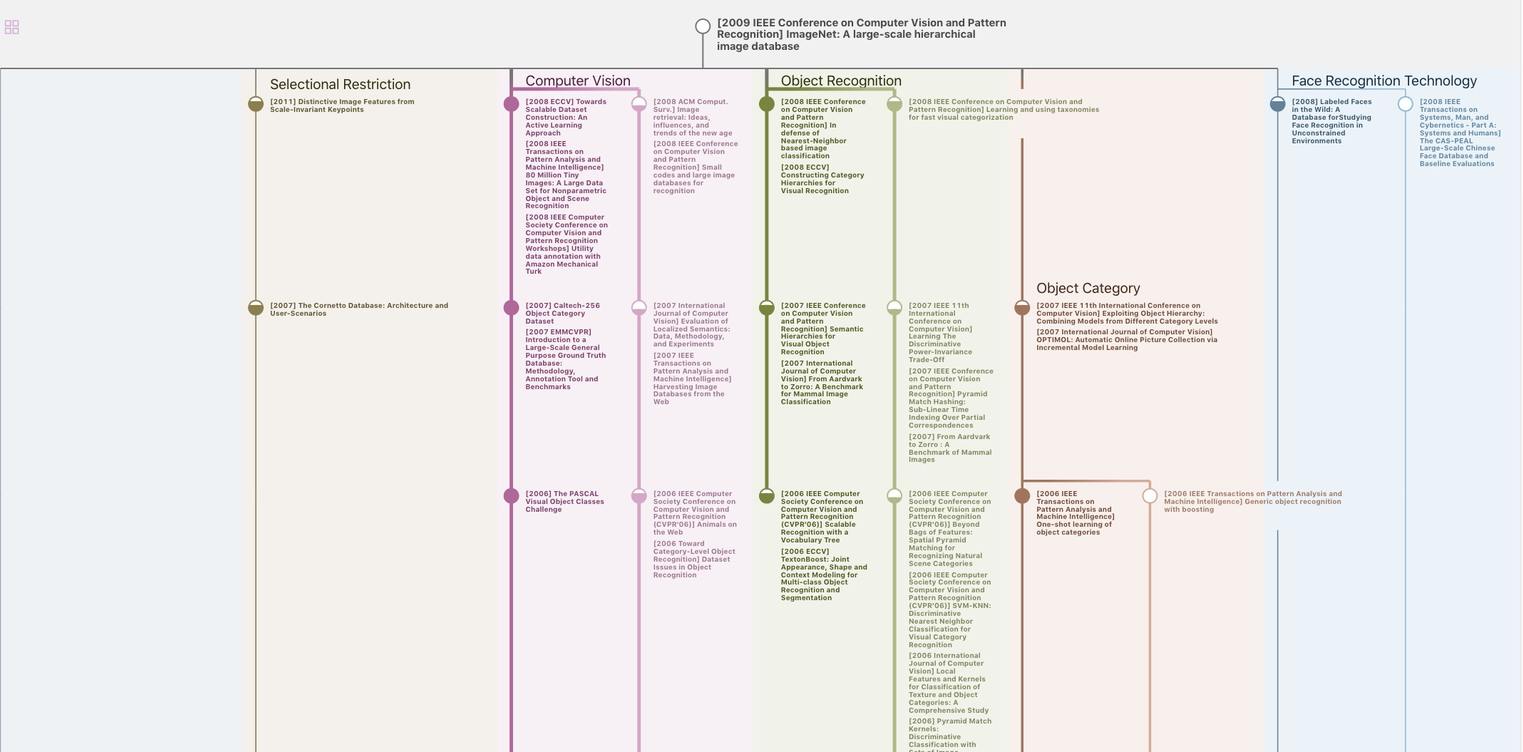
生成溯源树,研究论文发展脉络
Chat Paper
正在生成论文摘要