Efficient Exploration via Fragmentation and Recall
ICLR 2023(2023)
摘要
Efficient exploration and model-building are critical for learning in large state- spaces. However, agents typically face problems like getting stuck locally during exploration and catastrophic forgetting in their construction of models when the environments are heterogeneous. Here, we propose and apply the concept of Fragmentation-and-Recall to solve spatial (FarMap) and reinforcement learning problems (FarCuriosity). Agents construct local maps or local models, respectively, which are used to predict the current observation. High surprisal points lead to a fragmentation event. At fracture points, we store the current map or model fragment in a long-term memory (LTM) and initialize a new fragment. On the other hand, Fragments are recalled (and thus reused) from LTM if the observations of their fracture points match the agent’s current observation during exploration. The set of fracture points defines a set of intrinsic potential subgoals. Agents choose their next subgoal from the set of near and far potential subgoals in the current fragment or LTM, respectively. Thus, local maps and model fragments guide exploration locally and avoid catastrophic forgetting in learning heterogeneous environments, while LTM promotes exploration more globally. We evaluate FarMap and FarCuriosity on complex procedurally-generated spatial environments and on reinforcement learning benchmarks and demonstrate that the proposed methods are more efficient at exploration and memory use, and in harvesting extrinsic rewards, respectively.
更多查看译文
关键词
fragmentation,recall,exploration,cognitive science,neuroscience,curiosity,reinforcement learning,spatial navigation
AI 理解论文
溯源树
样例
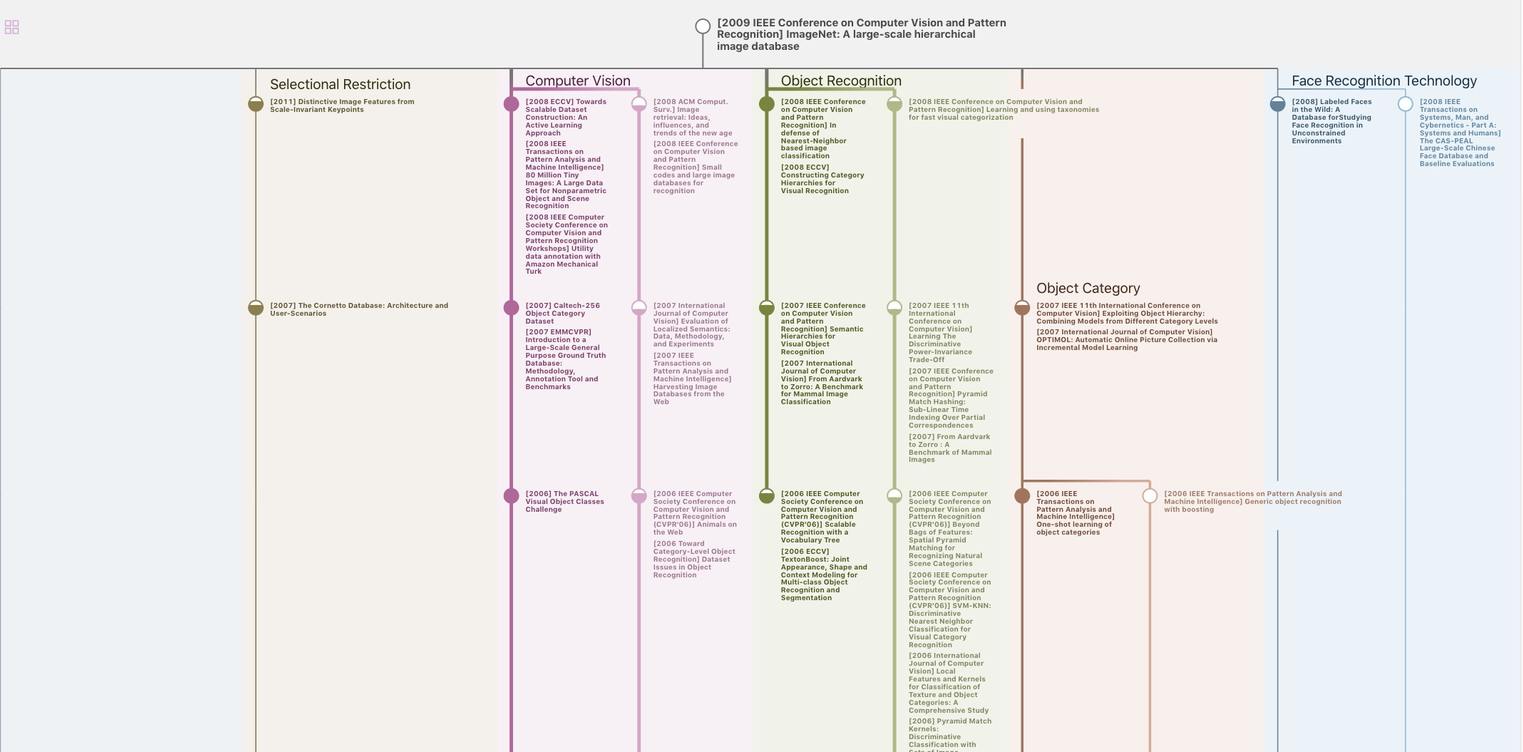
生成溯源树,研究论文发展脉络
Chat Paper
正在生成论文摘要