Convolutional Transformer Fusion Blocks for Multi-Modal Gesture Recognition
IEEE Access(2023)
摘要
Gesture recognition defines an important information channel in human-computer interaction. Intuitively, combining inputs from multiple modalities improves the recognition rate. In this work, we explore multi-modal video-based gesture recognition tasks by fusing spatio-temporal representation of relevant distinguishing features from different modalities. We present a self-attention based transformer fusion architecture to distill the knowledge from different modalities in two-stream convolutional neural networks (CNNs). For this, we introduce convolutions into the self-attention function and design the Convolutional Transformer Fusion Blocks (CTFB) for multi-modal data fusion. These fusion blocks can be easily added at different abstraction levels of the feature hierarchy in existing two-stream CNNs. In addition, the information exchange between two-stream CNNs along the feature hierarchy has so far been barely explored. We propose and evaluate different architectures for multi-level fusion pathways using CTFB to gain insights into the information flow between both streams. Our method achieves state-of-the-art or competitive performance on three benchmark gesture recognition datasets: a) IsoGD, b) NVGesture, and c) IPN hand. Extensive evaluation demonstrates the effectiveness of the proposed CTFB both in terms of recognition rate as well as resource efficiency.
更多查看译文
关键词
Self-attention,transformer,gesture recognition,multi-modal fusion
AI 理解论文
溯源树
样例
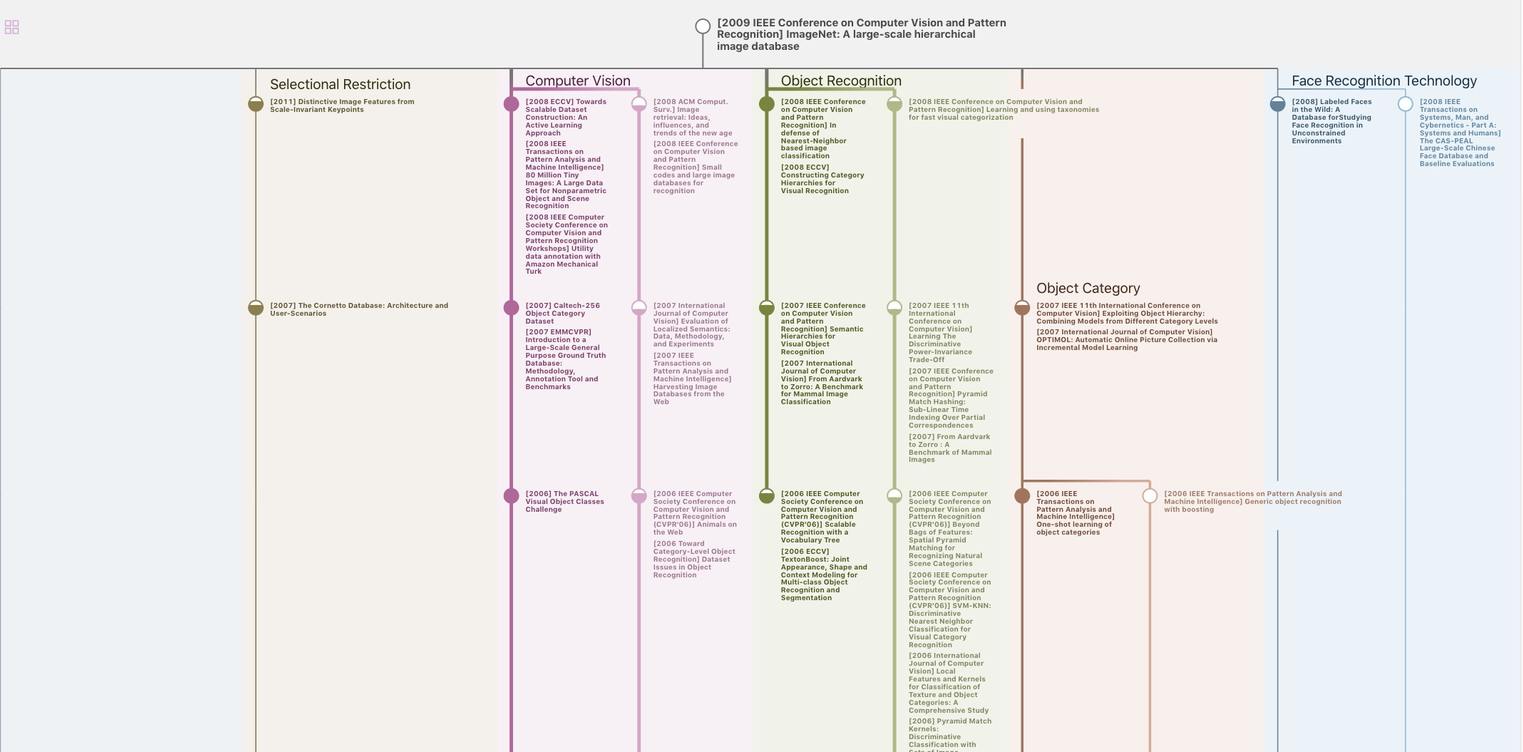
生成溯源树,研究论文发展脉络
Chat Paper
正在生成论文摘要