Towards Active Learning for Action Spotting in Association Football Videos
CoRR(2023)
摘要
Association football is a complex and dynamic sport, with numerous actions occurring simultaneously in each game. Analyzing football videos is challenging and requires identifying subtle and diverse spatio-temporal patterns. Despite recent advances in computer vision, current algorithms still face significant challenges when learning from limited annotated data, lowering their performance in detecting these patterns. In this paper, we propose an active learning framework that selects the most informative video samples to be annotated next, thus drastically reducing the annotation effort and accelerating the training of action spotting models to reach the highest accuracy at a faster pace. Our approach leverages the notion of uncertainty sampling to select the most challenging video clips to train on next, hastening the learning process of the algorithm. We demonstrate that our proposed active learning framework effectively reduces the required training data for accurate action spotting in football videos. We achieve similar performances for action spotting with NetVLAD++ on SoccerNet-v2, using only one-third of the dataset, indicating significant capabilities for reducing annotation time and improving data efficiency. We further validate our approach on two new datasets that focus on temporally localizing actions of headers and passes, proving its effectiveness across different action semantics in football. We believe our active learning framework for action spotting would support further applications of action spotting algorithms and accelerate annotation campaigns in the sports domain.
更多查看译文
关键词
accurate action,action spotting models,active learning framework,annotated data,annotation campaigns,annotation effort,annotation time,association football videos,challenging video clips,complex sport,different action semantics,diverse spatio-temporal patterns,dynamic sport,improving data efficiency,informative video samples,learning process,numerous actions,required training data,subtle spatio-temporal patterns,temporally localizing actions,towards active learning
AI 理解论文
溯源树
样例
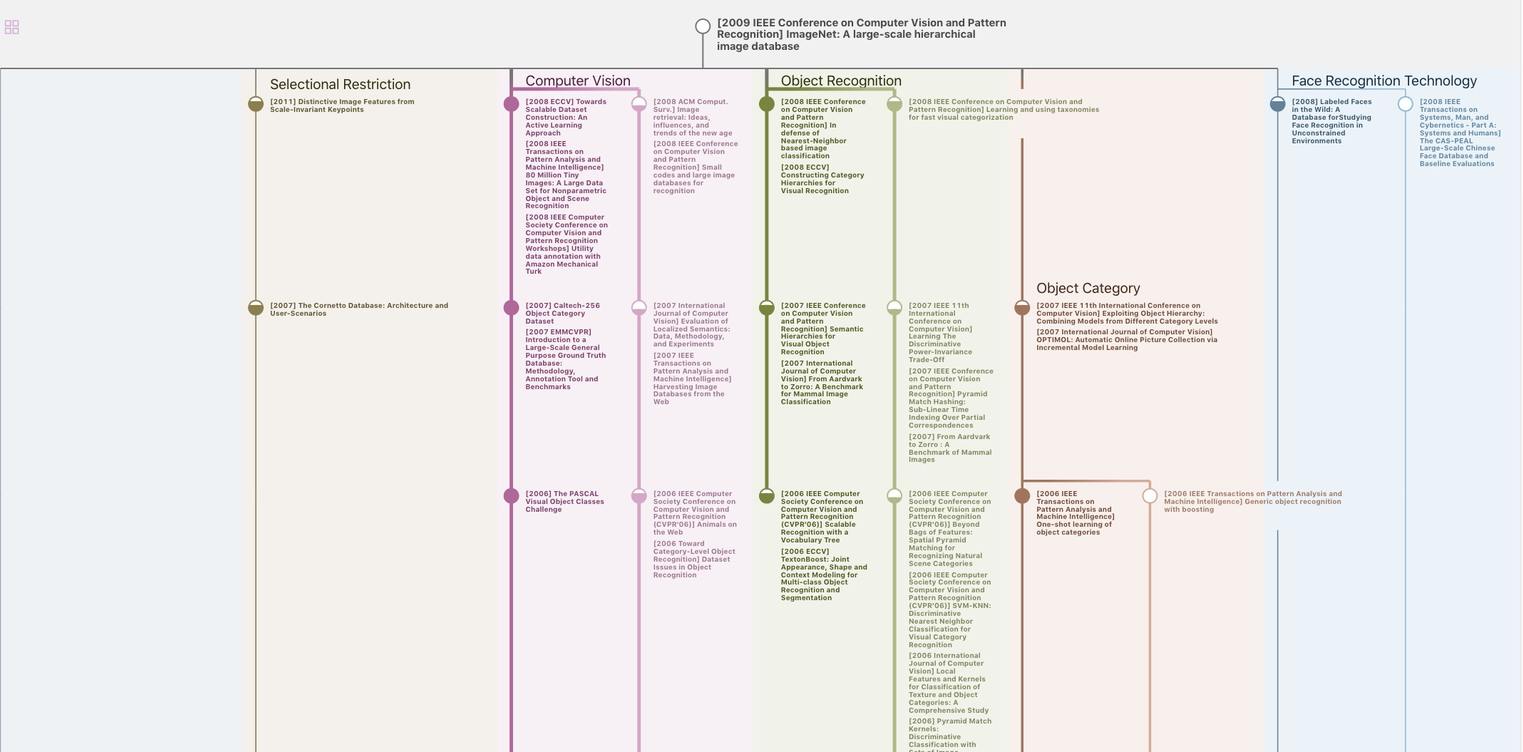
生成溯源树,研究论文发展脉络
Chat Paper
正在生成论文摘要