Point-SLAM: Dense Neural Point Cloud-based SLAM
CoRR(2023)
摘要
We propose a dense neural simultaneous localization and mapping (SLAM) approach for monocular RGBD input which anchors the features of a neural scene representation in a point cloud that is iteratively generated in an input-dependent data-driven manner. We demonstrate that both tracking and mapping can be performed with the same point-based neural scene representation by minimizing an RGBD-based re-rendering loss. In contrast to recent dense neural SLAM methods which anchor the scene features in a sparse grid, our point-based approach allows dynamically adapting the anchor point density to the information density of the input. This strategy reduces runtime and memory usage in regions with fewer details and dedicates higher point density to resolve fine details. Our approach performs either better or competitive to existing dense neural RGBD SLAM methods in tracking, mapping and rendering accuracy on the Replica, TUM-RGBD and ScanNet datasets. The source code is available at https://github.com/tfy14esa/Point-SLAM.
更多查看译文
关键词
point-slam,cloud-based
AI 理解论文
溯源树
样例
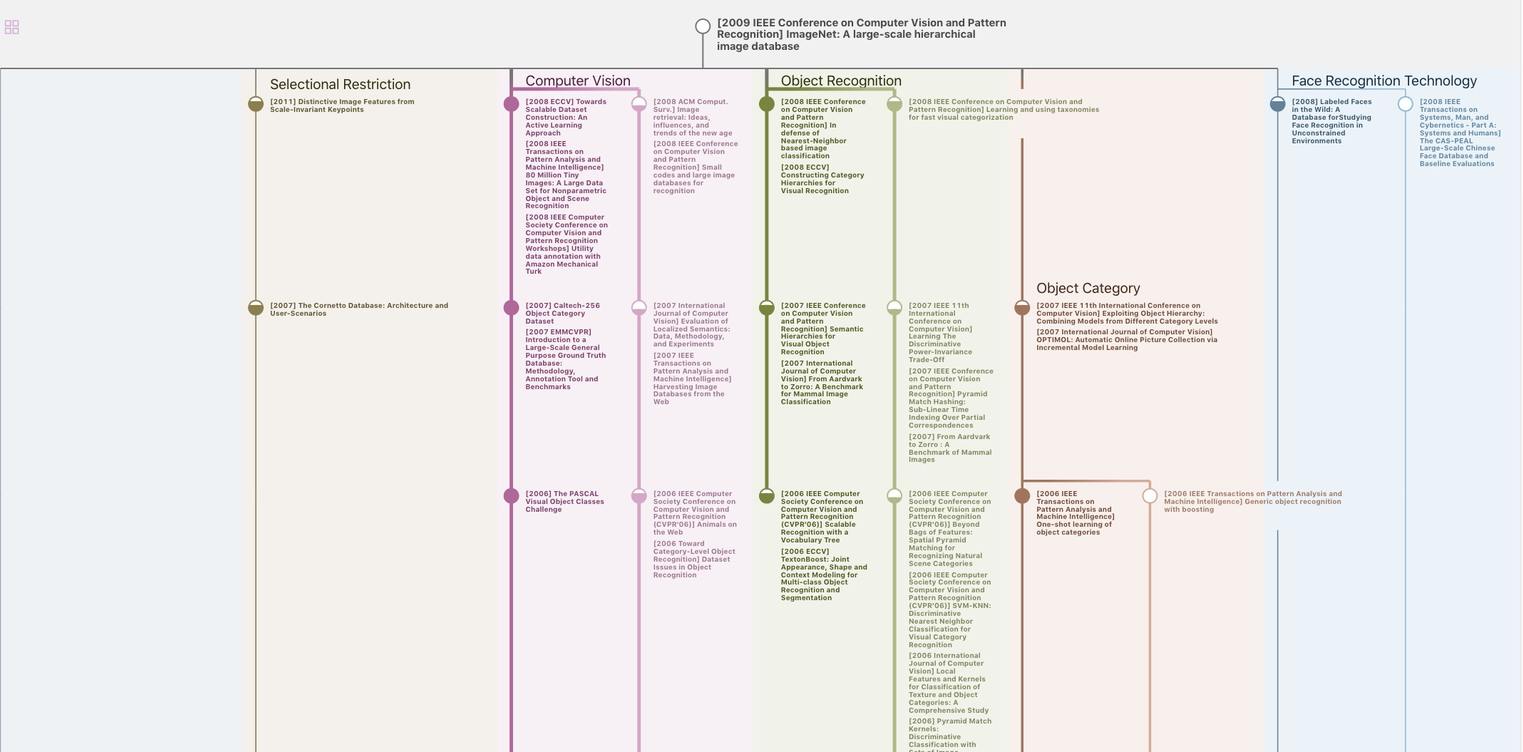
生成溯源树,研究论文发展脉络
Chat Paper
正在生成论文摘要