Ensemble Modeling for Time Series Forecasting: an Adaptive Robust Optimization Approach
CoRR(2023)
摘要
Accurate time series forecasting is critical for a wide range of problems with temporal data. Ensemble modeling is a well-established technique for leveraging multiple predictive models to increase accuracy and robustness, as the performance of a single predictor can be highly variable due to shifts in the underlying data distribution. This paper proposes a new methodology for building robust ensembles of time series forecasting models. Our approach utilizes Adaptive Robust Optimization (ARO) to construct a linear regression ensemble in which the models' weights can adapt over time. We demonstrate the effectiveness of our method through a series of synthetic experiments and real-world applications, including air pollution management, energy consumption forecasting, and tropical cyclone intensity forecasting. Our results show that our adaptive ensembles outperform the best ensemble member in hindsight by 16-26% in root mean square error and 14-28% in conditional value at risk and improve over competitive ensemble techniques.
更多查看译文
关键词
ensemble modeling,time series forecasting,adaptive robust
AI 理解论文
溯源树
样例
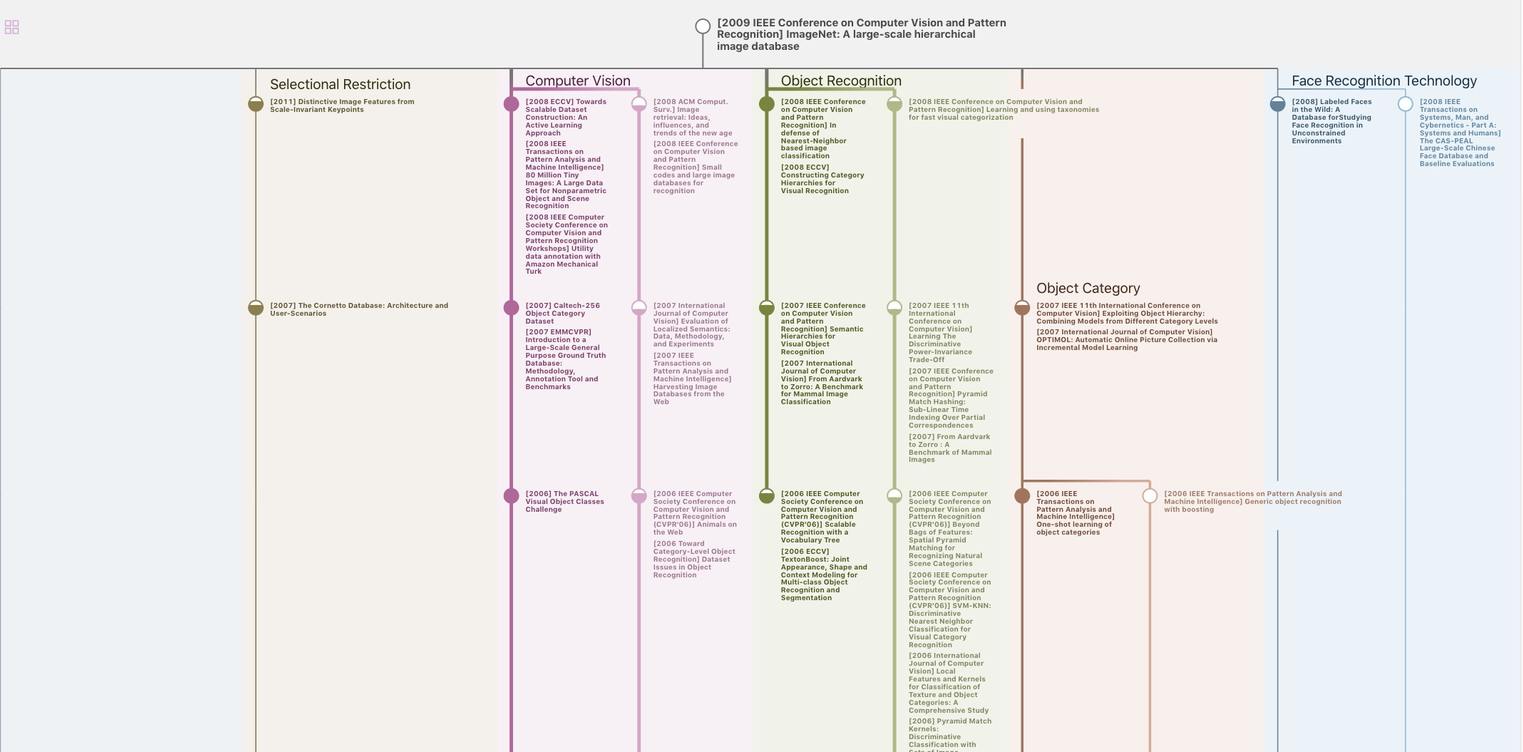
生成溯源树,研究论文发展脉络
Chat Paper
正在生成论文摘要