Learning metric volume estimation of fruits and vegetables from short monocular video sequences.
Heliyon(2023)
摘要
We present a novel approach for extracting metric volume information of fruits and vegetables from short monocular video sequences and associated inertial data recorded with a hand-held smartphone. Estimated segmentation masks from a pre-trained object detector are fused with the predicted change in relative pose obtained from the inertial data to predict the class and volume of the objects of interest. Our approach works with simple RGB video frames and inertial data which are readily available from modern smartphones. It does not require reference objects of known size in the video frames. Using a balanced validation dataset, we achieve a classification accuracy of 95% and a mean absolute percentage error for the volume prediction of 16% on untrained objects, which is comparable to state-of-the-art results requiring more elaborated data recording setups. A very accurate estimation of the model uncertainty is achieved through ensembling and the use of Gaussian negative log-likelihood loss. The dataset used in our experiments including ground-truth volume information is available at https://sst.aau.at/cns/datasets.
更多查看译文
关键词
Deep learning,Food datasets,Fusion,Image recognition,Sensor,Volume estimation
AI 理解论文
溯源树
样例
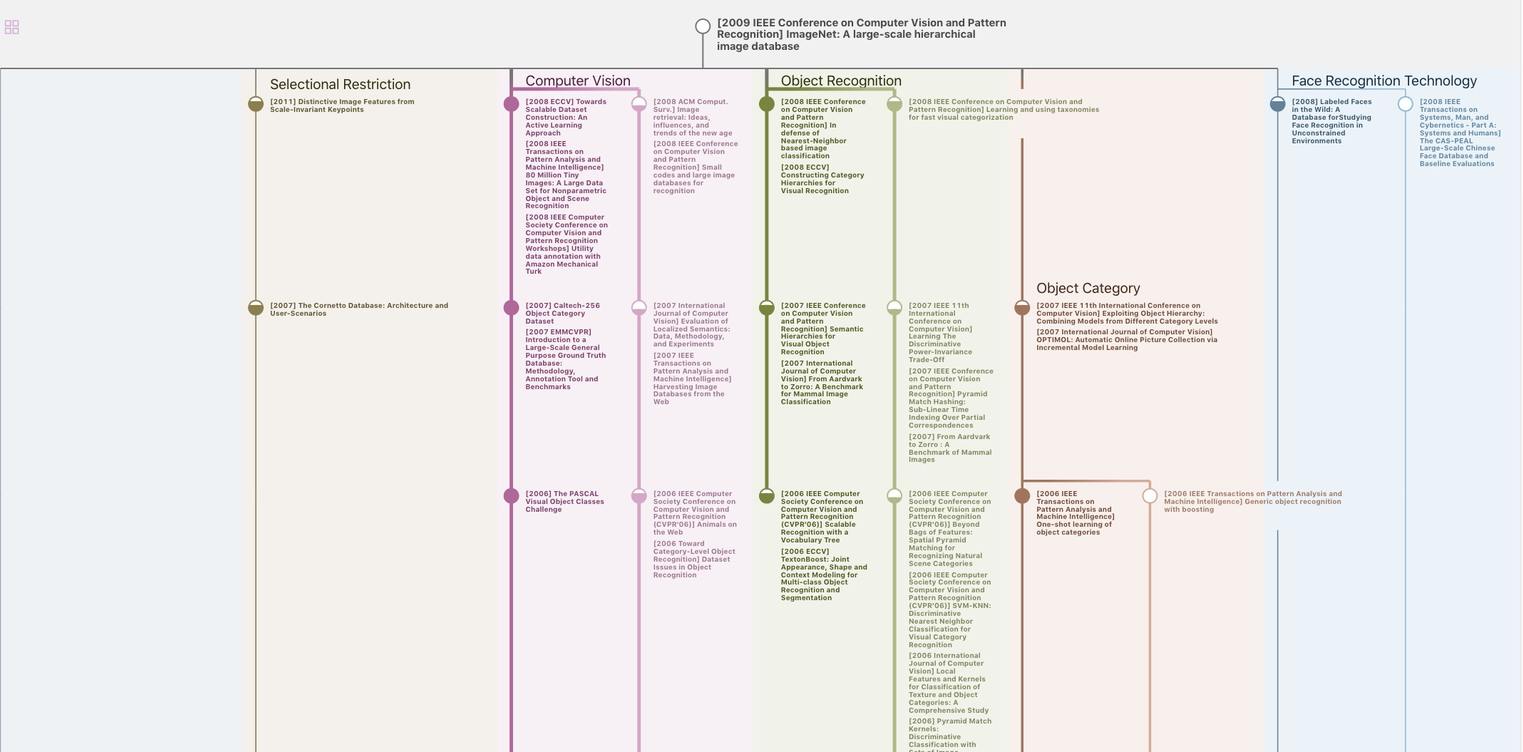
生成溯源树,研究论文发展脉络
Chat Paper
正在生成论文摘要