m-NLP Inference Models Using Simulation and Regression Techniques.
Journal of geophysical research. Space physics(2023)
摘要
Current inference techniques for processing multi-needle Langmuir probe (m-NLP) data are often based on adaptations of the Orbital Motion-Limited (OML) theory which relies on several simplifying assumptions. Some of these assumptions, however, are typically not well satisfied in actual experimental conditions, thus leading to uncontrolled uncertainties in inferred plasma parameters. In order to remedy this difficulty, three-dimensional kinetic particle in cell simulations are used to construct a synthetic data set, which is used to compare and assess different m-NLP inference techniques. Using a synthetic data set, regression-based models capable of inferring electron density and satellite potentials from 4-tuples of currents collected with fixed-bias needle probes similar to those on the NorSat-1 satellite, are trained and validated. The regression techniques presented show promising results for plasma density inferences with RMS relative errors less than 20%, and satellite potential inferences with RMS errors less than 0.2 V for potentials ranging from -6 to -1 V. The new inference approaches presented are applied to NorSat-1 data, and compared with existing state-of-the-art inference techniques.
更多查看译文
关键词
machine learning inference,multivariate regression,multi‐needle Langmuir probe,particle in cell simulation
AI 理解论文
溯源树
样例
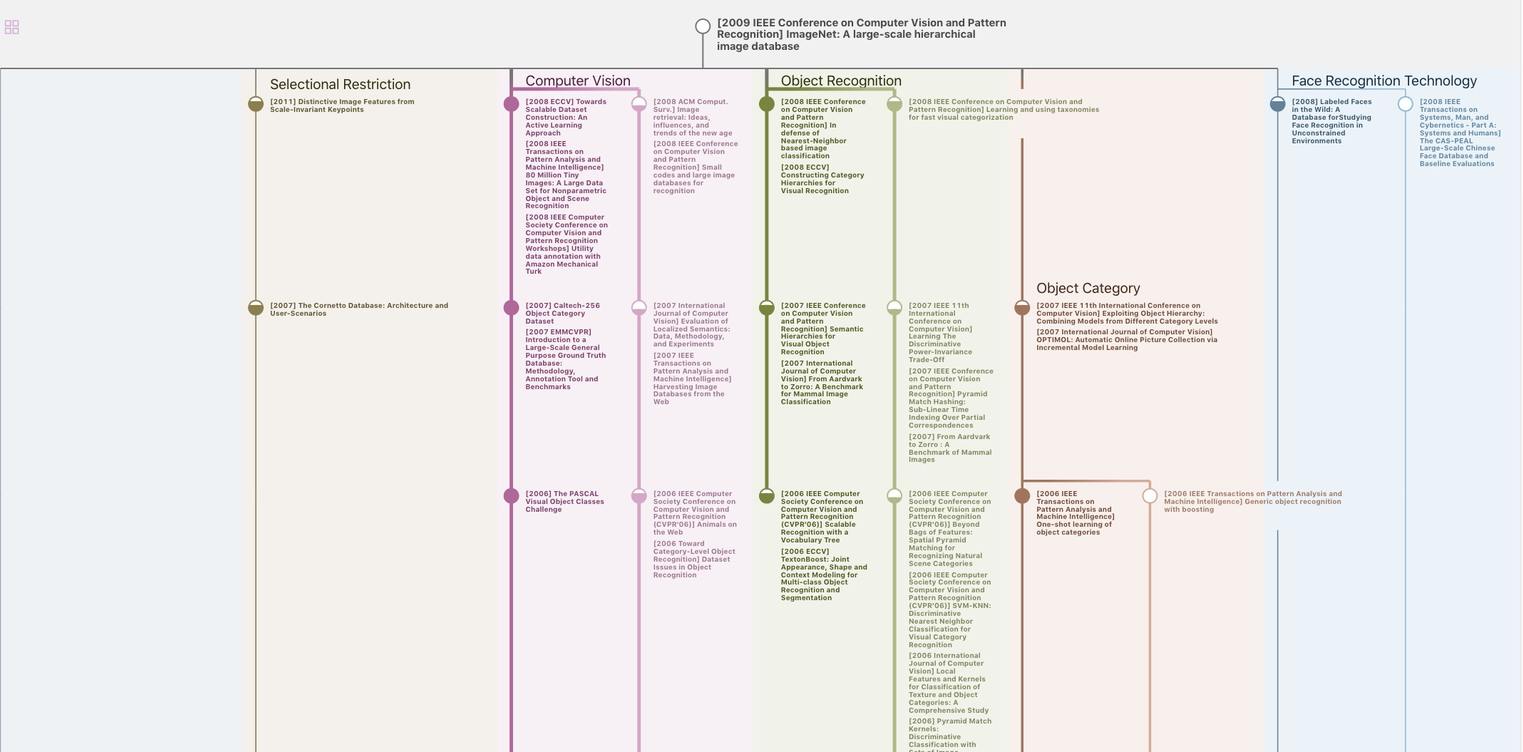
生成溯源树,研究论文发展脉络
Chat Paper
正在生成论文摘要