Analog nanophotonic computing going practical: silicon photonic deep learning engines for tiled optical matrix multiplication with dynamic precision
Nanophotonics(2023)
摘要
Analog photonic computing comprises a promising candidate for accelerating the linear operations of deep neural networks (DNNs), since it provides ultrahigh bandwidth, low footprint and low power consumption computing capabilities. However, the confined photonic hardware size, along with the limited bit precision of high-speed electro-optical components, impose stringent requirements towards surpassing the performance levels of current digital processors. Herein, we propose and experimentally demonstrate a speed-optimized dynamic precision neural network (NN) inference via tiled matrix multiplication (TMM) on a low-radix silicon photonic processor. We introduce a theoretical model that relates the noise figure of a photonic neuron with the bit precision requirements per neural layer. The inference evaluation of an NN trained for the classification of the IRIS dataset is, then, experimentally performed over a silicon coherent photonic neuron that can support optical TMM up to 50 GHz, allowing, simultaneously, for dynamic-precision calculations. Targeting on a high-accuracy and speed-optimized classification performance, we experimentally applied the model-extracted mixed-precision NN inference scheme via the respective alteration of the operational compute rates per neural layer. This dynamic-precision NN inference revealed a 55% decrease in the execution time of the linear operations compared to a fixed-precision scheme, without degrading its accuracy.
更多查看译文
关键词
analog computing,deep learning,dynamic precision inference,photonic computing,silicon photonics,tiled matrix multiplication
AI 理解论文
溯源树
样例
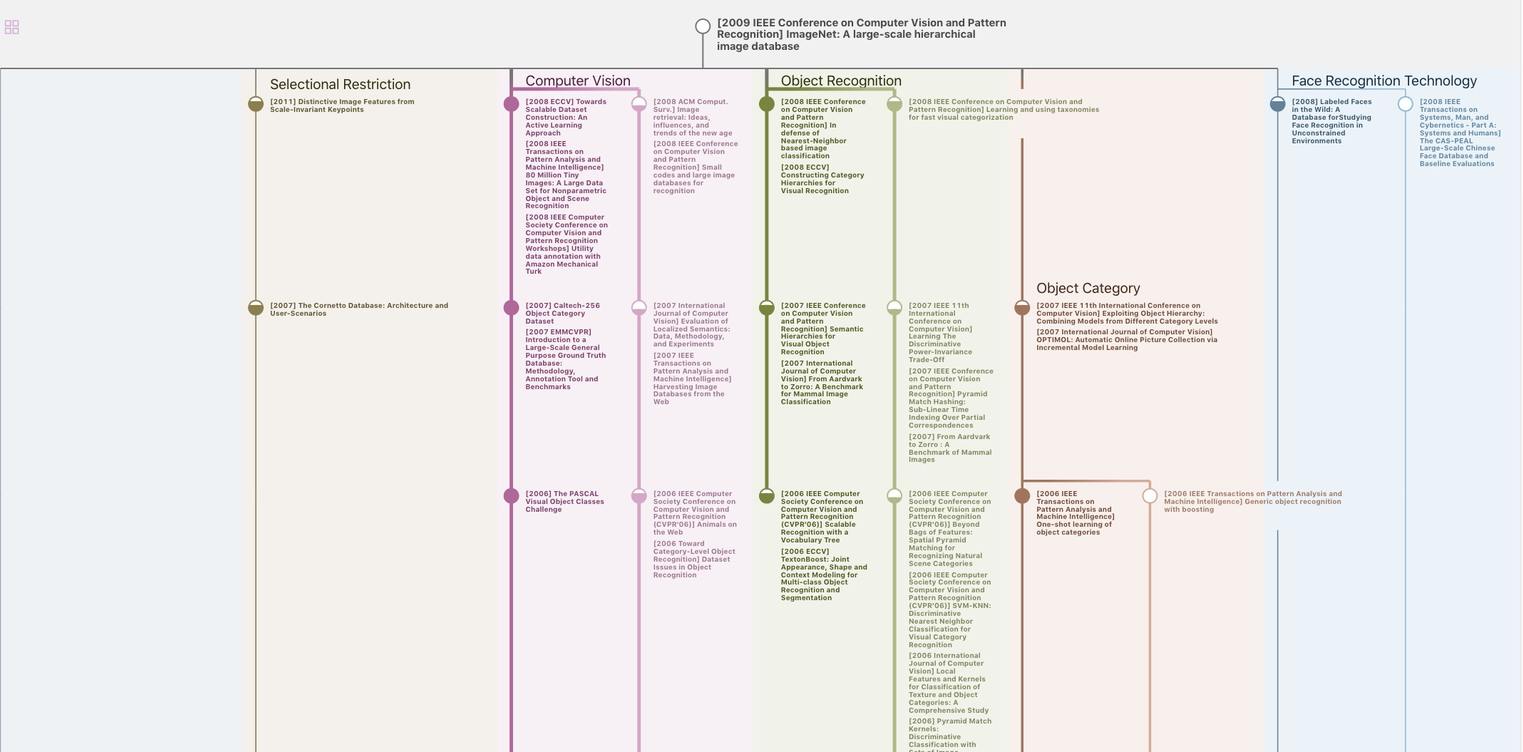
生成溯源树,研究论文发展脉络
Chat Paper
正在生成论文摘要