Detecting Online Grooming By Simple Contrastive Chat Embeddings
PROCEEDINGS OF THE 9TH ACM INTERNATIONAL WORKSHOP ON SECURITY AND PRIVACY ANALYTICS, IWSPA 2023(2023)
摘要
Protecting children against predators that use the internet as a medium to find their victims is essential, where the difficulty of monitoring online messaging platforms to avoid potential threats toward underage users is alarming. Online grooming detection requires a deep knowledge of predatory behaviour where an online conversation's positive or negative connotations rely on the context where it can change rapidly in an online chat. Therefore, it is essential to use robust feature vectors for predatory conversation detection. This research paper proposes a contrastive learning framework for feature extraction in a sentence-based manner where it can assign a feature vector to the conversation with misspellings using subword information. Also, it is vital to have a high detection rate of true positives to avoid potential non-wanted consequences. We propose a configuration of RoBERTa encoders and supervised SimCSE for training the SVM model, leading to a high rate of detecting relevant samples (predatory samples). Our proposed approach gains an F-0-score of 0.96, an F-1-score of 0.96, and an accuracy of 0.99 for predatory conversation detection that benchmarks the state-of-the-art. In order to improve the performance, we also perform experiments with various fusion approaches, where the sum fusion of all configurations obtains an accuracy of 0.99, an F-1-score of 0.97, and an F-0.5-score of 0.98.
更多查看译文
关键词
Predatory Conversation Detection,Online Grooming,Transfer Learning,Semantic Analysis,Bert,Sentence Embedding
AI 理解论文
溯源树
样例
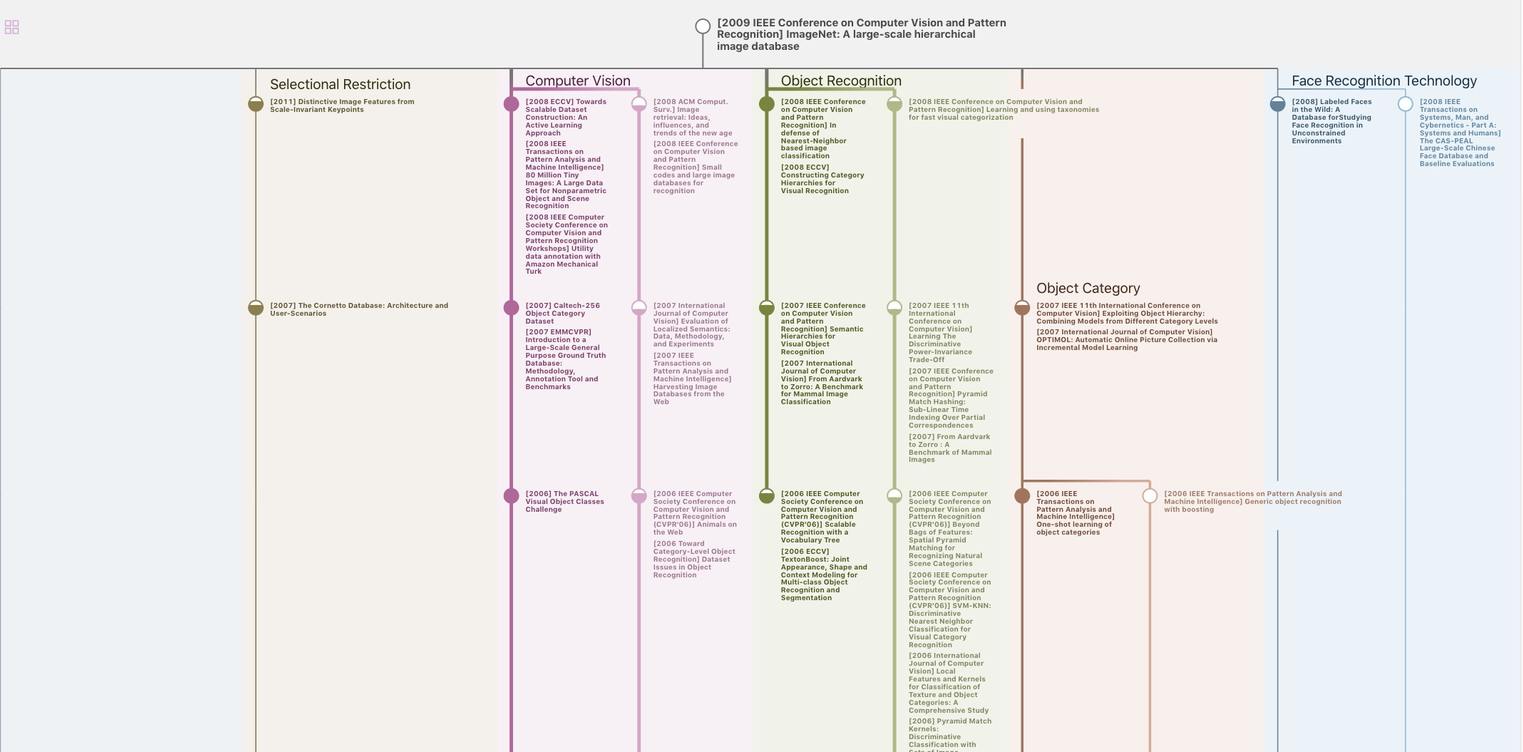
生成溯源树,研究论文发展脉络
Chat Paper
正在生成论文摘要