ATPP: A Mobile App Prediction System Based on Deep Marked Temporal Point Processes
ACM Transactions on Sensor Networks(2023)
摘要
Predicting the next application (app) a user will open is essential for improving the user experience, e.g., app pre-loading and app recommendation. Unlike previous solutions that only predict which app the user will open, this article predicts both the next app and the time to open it. Time prediction is essential to avoid loading the next app too early and consuming unnecessary resources on smartphones. To predict the next app and open time jointly, we model the app usage sequence as a marked temporal point process (MTPP), whose conditional intensity function can capture the probability of a new app usage event. We develop a novel data-driven MTPP-based app prediction system, named ATPP (App Temporal Point Process), which adopts a recurrent neural network architecture to learn the MTPP conditional intensity function for app prediction. ATPP adopts a set of techniques to incorporate the unique features of app prediction in our RNN architecture, including learning the correlated usage behavior of different apps by representation learning, the temporal dependency of app usage by an attention mechanism, and the location-related app usage behavior by feature extraction and fusion layer. We conduct extensive experiments on a large-scale anonymized app usage dataset to verify ATPP’s effectiveness.
更多查看译文
关键词
Mobile devices,application usage prediction,marked temporal point process,recurrent neural networks
AI 理解论文
溯源树
样例
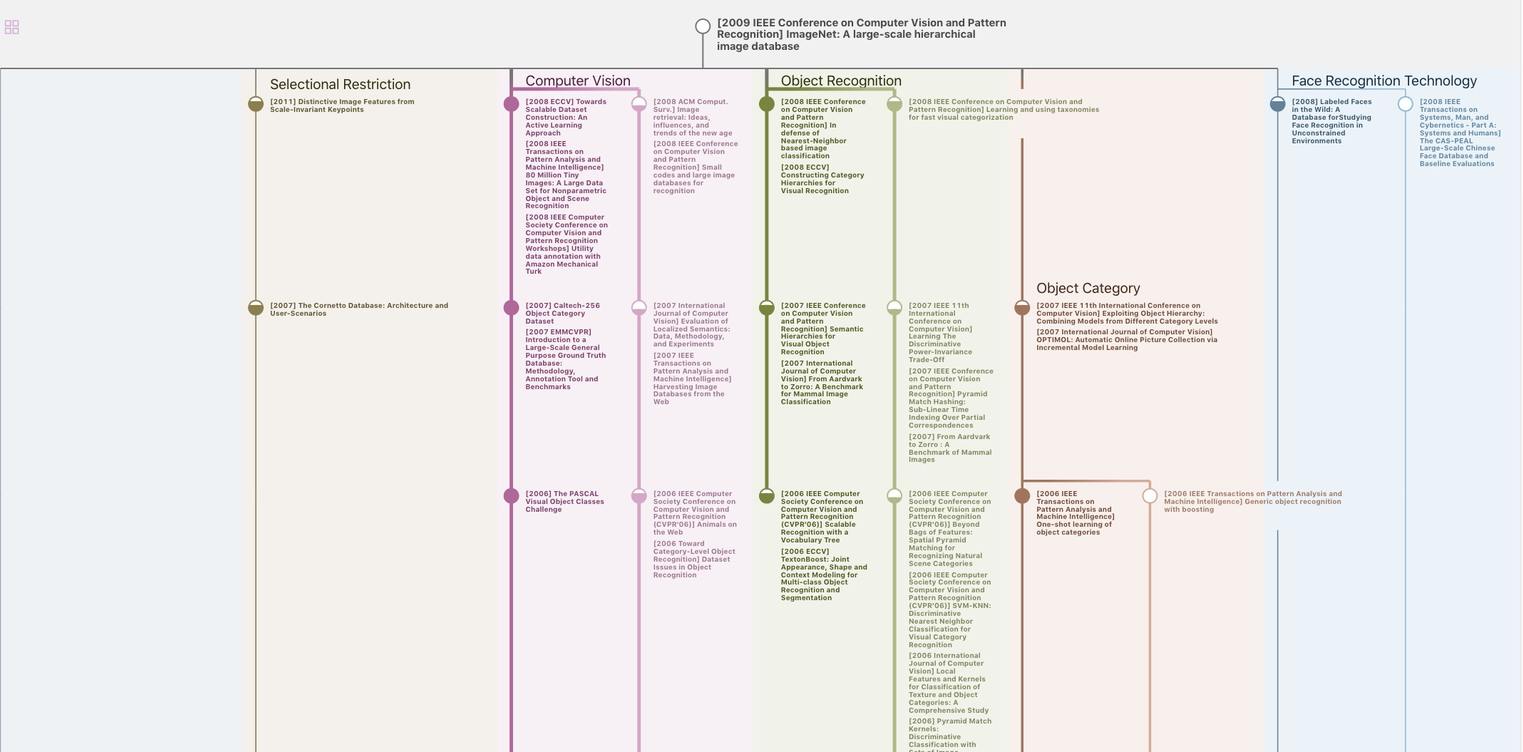
生成溯源树,研究论文发展脉络
Chat Paper
正在生成论文摘要