Causal Disentanglement for Implicit Recommendations with Network Information
ACM Transactions on Knowledge Discovery from Data(2023)
摘要
Online user engagement is highly influenced by various machine learning models, such as recommender systems. These systems recommend new items to the user based on the user’s historical interactions. Implicit recommender systems reflect a binary setting showing whether a user interacted (e.g., clicked on) with an item or not. However, the observed clicks may be due to various causes such as user’s interest, item’s popularity, and social influence factors. Traditional recommender systems consider these causes under a unified representation, which may lead to the emergence and amplification of various biases in recommendations. However, recent work indicates that by disentangling the unified representations, one can mitigate bias (e.g., popularity bias) in recommender systems and help improve recommendation performance. Yet, prior work in causal disentanglement in recommendations does not consider a crucial factor, that is, social influence. Social theories such as homophily and social influence provide evidence that a user’s decision can be highly influenced by the user’s social relations. Thus, accounting for the social relations while disentangling leads to less biased recommendations. To this end, we identify three separate causes behind an effect (e.g., clicks): (a) user’s interest, (b) item’s popularity, and (c) user’s social influence. Our approach seeks to causally disentangle the user and item latent features to mitigate popularity bias in implicit feedback–based social recommender systems. To achieve this goal, we draw from causal inference theories and social network theories and propose a causality-aware disentanglement method that leverages both the user–item interaction network and auxiliary social network information. Experiments on real-world datasets against various state-of-the-art baselines validate the effectiveness of the proposed model for mitigating popularity bias and generating de-biased recommendations.
更多查看译文
关键词
Causal recommender systems,popularity bias,conformity bias,social recommender systems
AI 理解论文
溯源树
样例
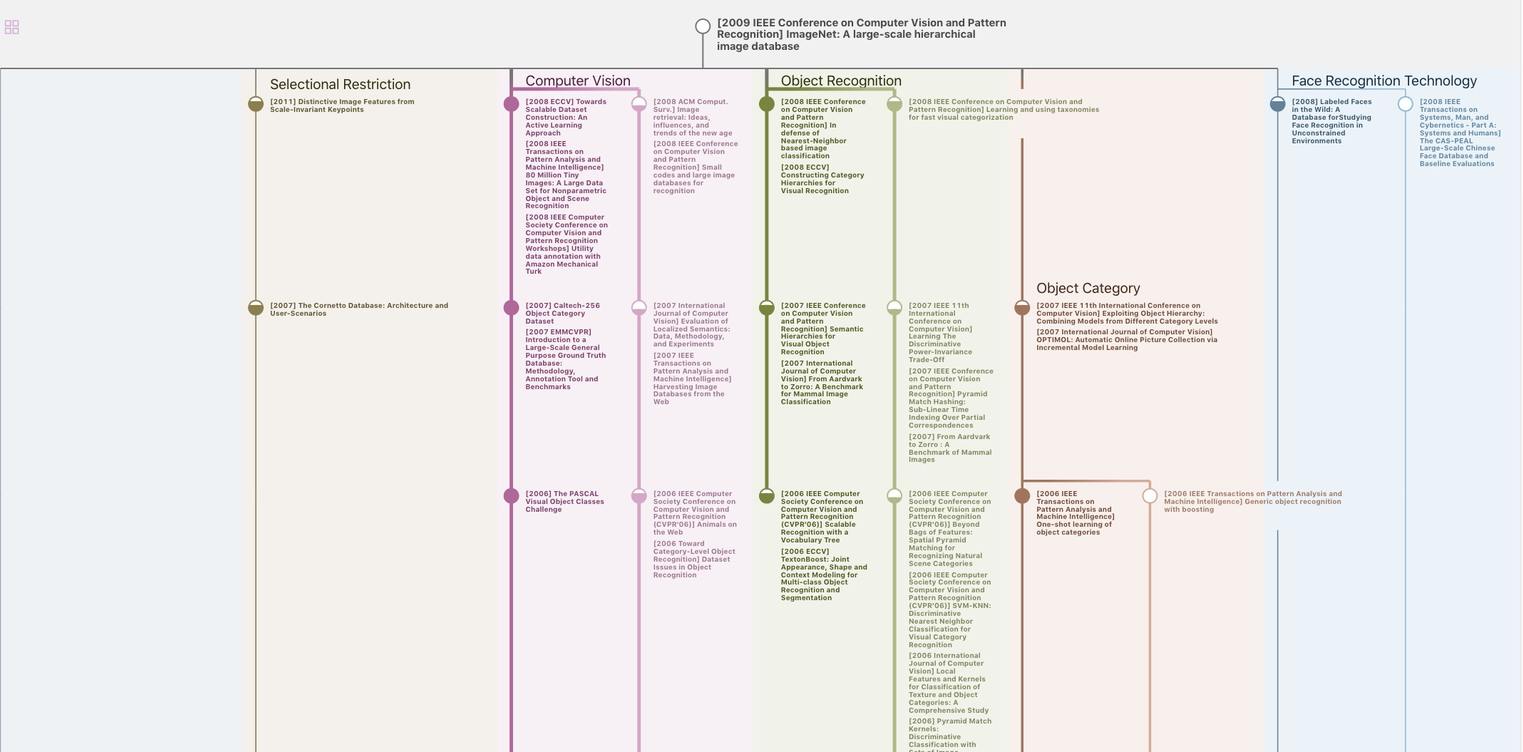
生成溯源树,研究论文发展脉络
Chat Paper
正在生成论文摘要