Benchmarking the Physical-world Adversarial Robustness of Vehicle Detection
CoRR(2023)
摘要
Adversarial attacks in the physical world can harm the robustness of detection models. Evaluating the robustness of detection models in the physical world can be challenging due to the time-consuming and labor-intensive nature of many experiments. Thus, virtual simulation experiments can provide a solution to this challenge. However, there is no unified detection benchmark based on virtual simulation environment. To address this challenge, we proposed an instant-level data generation pipeline based on the CARLA simulator. Using this pipeline, we generated the DCI dataset and conducted extensive experiments on three detection models and three physical adversarial attacks. The dataset covers 7 continuous and 1 discrete scenes, with over 40 angles, 20 distances, and 20,000 positions. The results indicate that Yolo v6 had strongest resistance, with only a 6.59% average AP drop, and ASA was the most effective attack algorithm with a 14.51% average AP reduction, twice that of other algorithms. Static scenes had higher recognition AP, and results under different weather conditions were similar. Adversarial attack algorithm improvement may be approaching its 'limitation'.
更多查看译文
关键词
detection,benchmarking,vehicle,physical-world
AI 理解论文
溯源树
样例
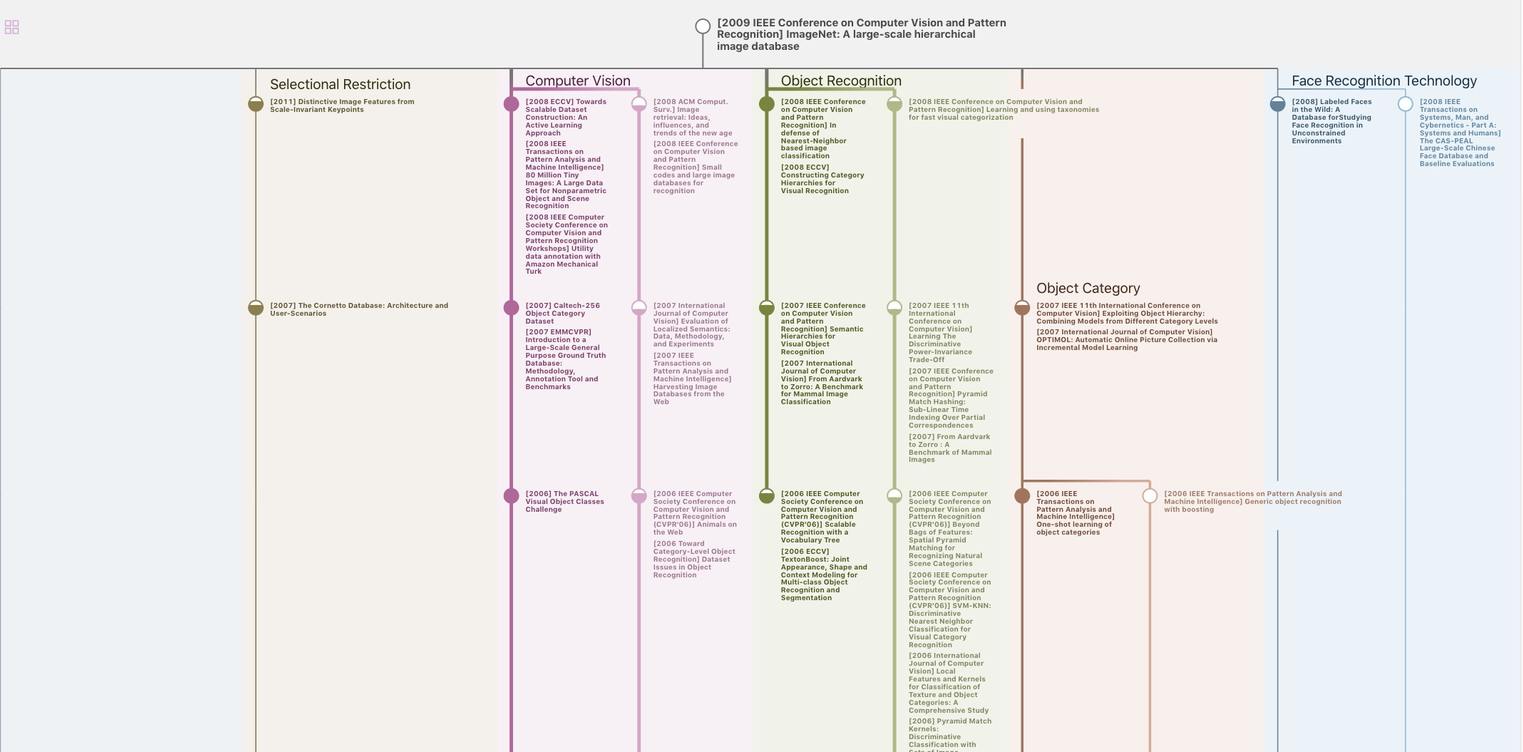
生成溯源树,研究论文发展脉络
Chat Paper
正在生成论文摘要