Pointless Global Bundle Adjustment With Relative Motions Hessians
CoRR(2023)
摘要
Bundle adjustment (BA) is the standard way to optimise camera poses and to produce sparse representations of a scene. However, as the number of camera poses and features grows, refinement through bundle adjustment becomes inefficient. Inspired by global motion averaging methods, we propose a new bundle adjustment objective which does not rely on image features' reprojection errors yet maintains precision on par with classical BA. Our method averages over relative motions while implicitly incorporating the contribution of the structure in the adjustment. To that end, we weight the objective function by local hessian matrices - a by-product of local bundle adjustments performed on relative motions (e.g., pairs or triplets) during the pose initialisation step. Such hessians are extremely rich as they encapsulate both the features' random errors and the geometric configuration between the cameras. These pieces of information propagated to the global frame help to guide the final optimisation in a more rigorous way. We argue that this approach is an upgraded version of the motion averaging approach and demonstrate its effectiveness on both photogrammetric datasets and computer vision benchmarks.
更多查看译文
关键词
camera poses,computer vision benchmarks,global frame help,global motion averaging methods,image features,local bundle adjustments,local hessian matrices,motion averaging approach,objective function,photogrammetric datasets,pointless global bundle adjustment,pose initialisation step,relative motions hessians,sparse representations
AI 理解论文
溯源树
样例
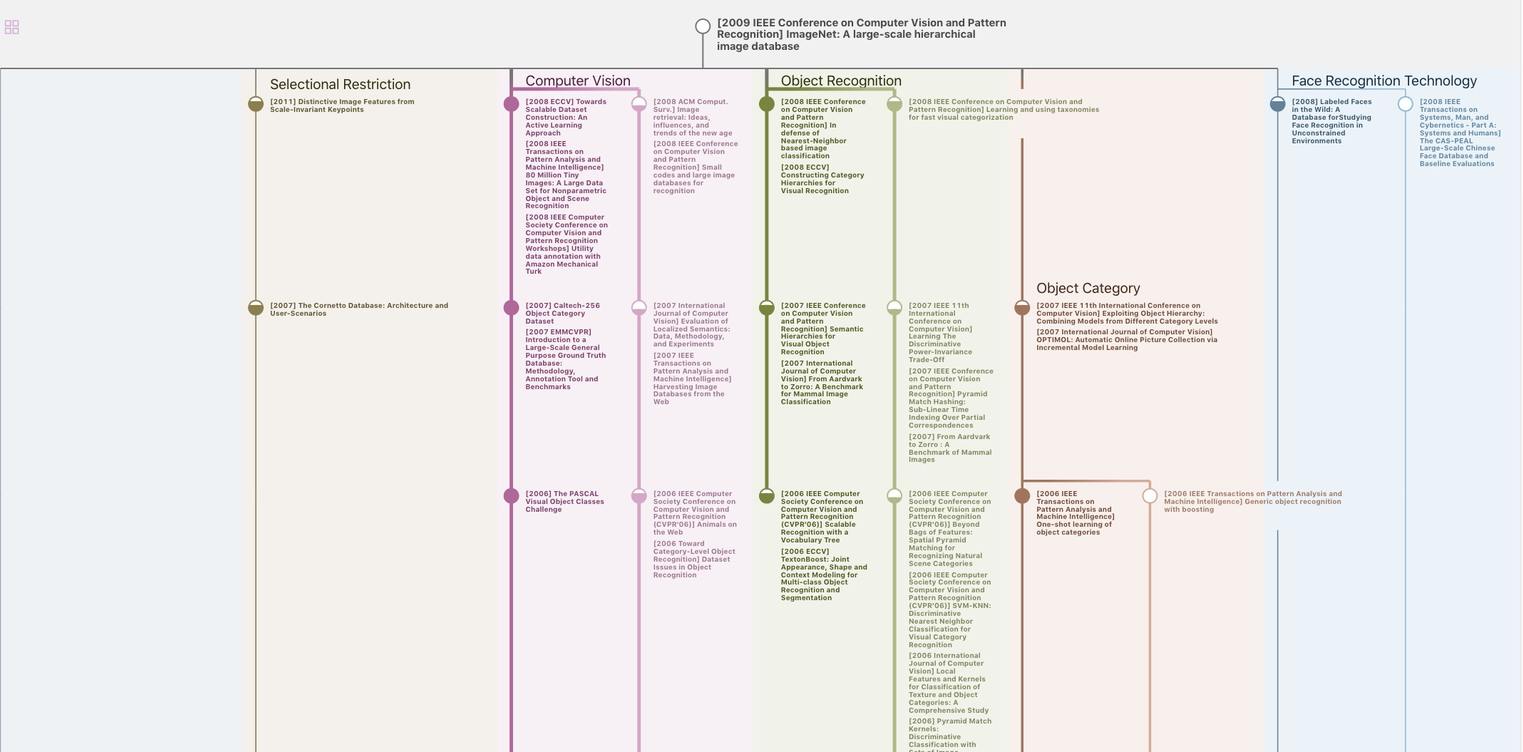
生成溯源树,研究论文发展脉络
Chat Paper
正在生成论文摘要