Fast IMU-based Dual Estimation of Human Motion and Kinematic Parameters via Progressive In-Network Computing
IFAC PAPERSONLINE(2023)
摘要
Many applications involve humans in the loop, where continuous and accurate human motion monitoring provides valuable information for safe and intuitive human-machine interaction. Portable devices such as inertial measurement units (IMUs) are applicable to monitor human motions, while in practice often limited computational power is available locally. The human motion in task space coordinates requires not only the human joint motion but also the nonlinear coordinate transformation depending on the parameters such as human limb length. In most applications, measuring these kinematics parameters for each individual requires undesirably high effort. Therefore, it is desirable to estimate both, the human motion and kinematic parameters from IMUs. In this work, we propose a novel computational framework for dual estimation in real-time exploiting in-network computational resources. We adopt the concept of field Kalman filtering, where the dual estimation problem is decomposed into a fast state estimation process and a computationally expensive parameter estimation process. In order to further accelerate the convergence, the parameter estimation is progressively computed on multiple networked computational nodes. The superiority of our proposed method is demonstrated by a simulation of a human arm, where the estimation accuracy is shown to converge faster than with conventional approaches.Copyright (c) 2023 The Authors.
更多查看译文
关键词
human motion estimation,dual estimation,Kalman filtering,networked system,progressive algorithm,IMU
AI 理解论文
溯源树
样例
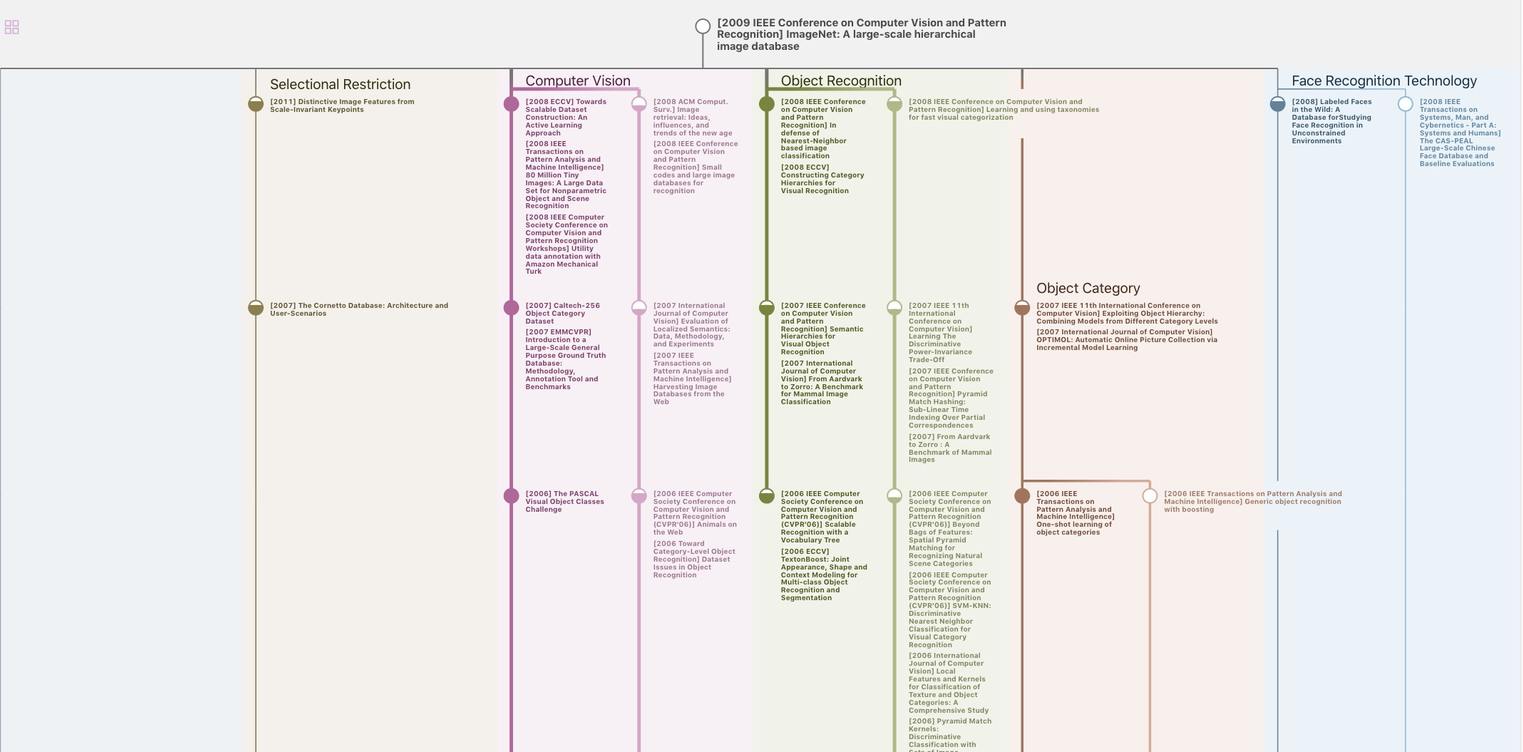
生成溯源树,研究论文发展脉络
Chat Paper
正在生成论文摘要