Predicting daily streamflow with a novel multi-regime switching ARIMA-MS-GARCH model
Journal of Hydrology: Regional Studies(2023)
摘要
Study region: Weihe River Basin of China Study focus: In recent decades, changing environments destroyed the natural structure of streamflow, making accurate streamflow prediction challenging. This study develops a multi -regime Markov-switching Generalized Autoregressive Conditional Heteroskedasticity (MS-GARCH) model to predict daily streamflow time series with structural breaks (SB), named ARIMA (Autoregressive Integrated Moving Average)-MS-GARCH model. Consequently, the multi-regime ARIMA-MS-GARCH model is compared with other classical single-regime ARIMA-GARCH models to evaluate whether and to what extent it improves streamflow prediction accuracy. New hydrology insights for the region: There exist structural breaks in the daily streamflow time series, and the number of breakpoints at each station varies. The GARCH model ignores the description of volatility aggregation in the daily streamflow time series (& alpha; < & beta;). The ARIMA-MS-GARCH model (R2 and NSE in the range of 0.682-0.984 and 0.582-0.935, respectively) out-performs the ARIMA-GARCH model (R2 and NSE in the range of 0.558-0.935 and 0.077-0.721, respectively) for daily streamflow prediction, and the ARIMA-GARCH model seriously over-estimates the peak values. The MS-GARCH model based on the Student's t distribution is more suitable for daily streamflow prediction than that based on the normal distribution, in which MAE and RE reduce by 23.90%-52.28% and 23.46%-54.67%, respectively, and R2 and NSE increase by 5.09% -15.54% and 1.63% -60.65%.
更多查看译文
关键词
Weihe River Basin,Daily streamflow,Heteroscedasticity,Multi -regime,MS-GARCH
AI 理解论文
溯源树
样例
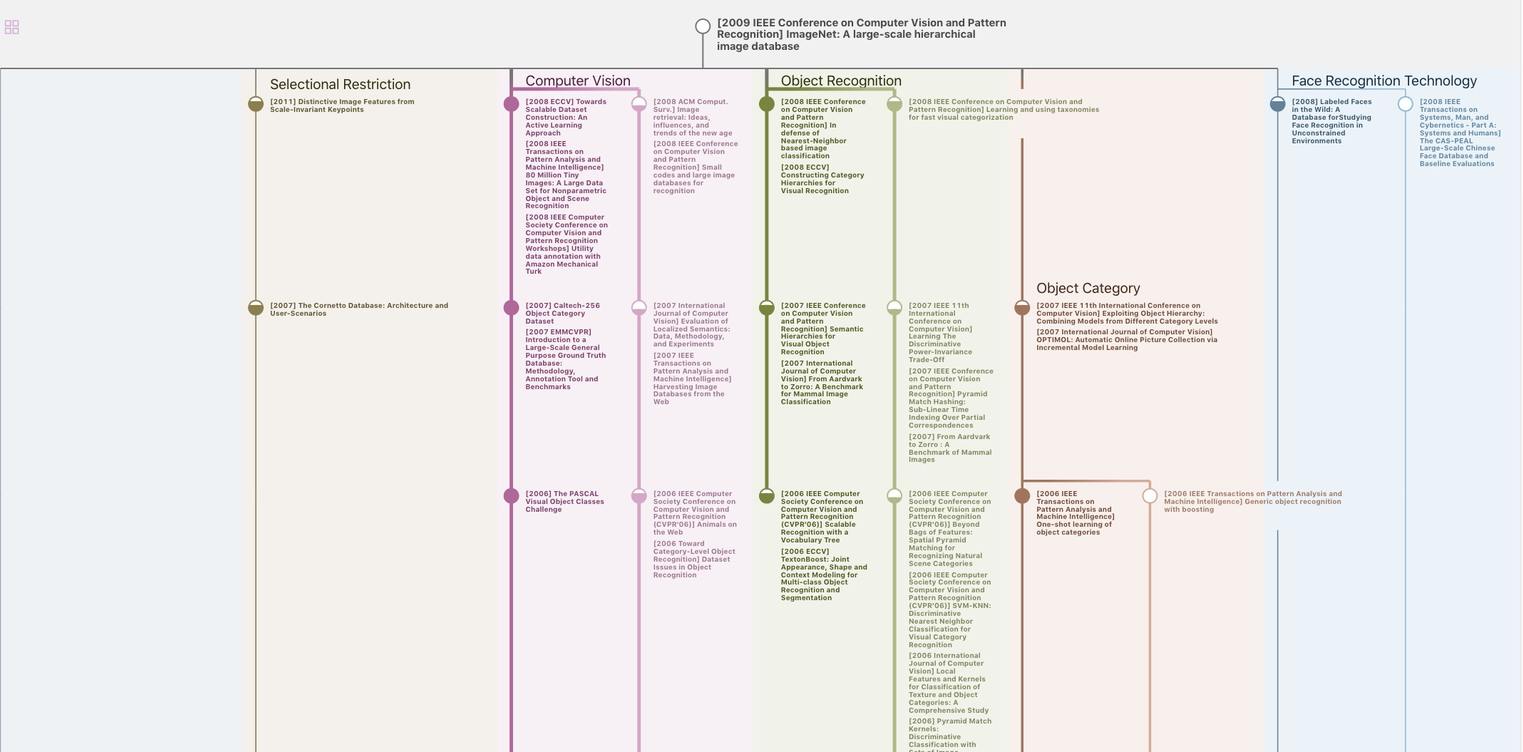
生成溯源树,研究论文发展脉络
Chat Paper
正在生成论文摘要